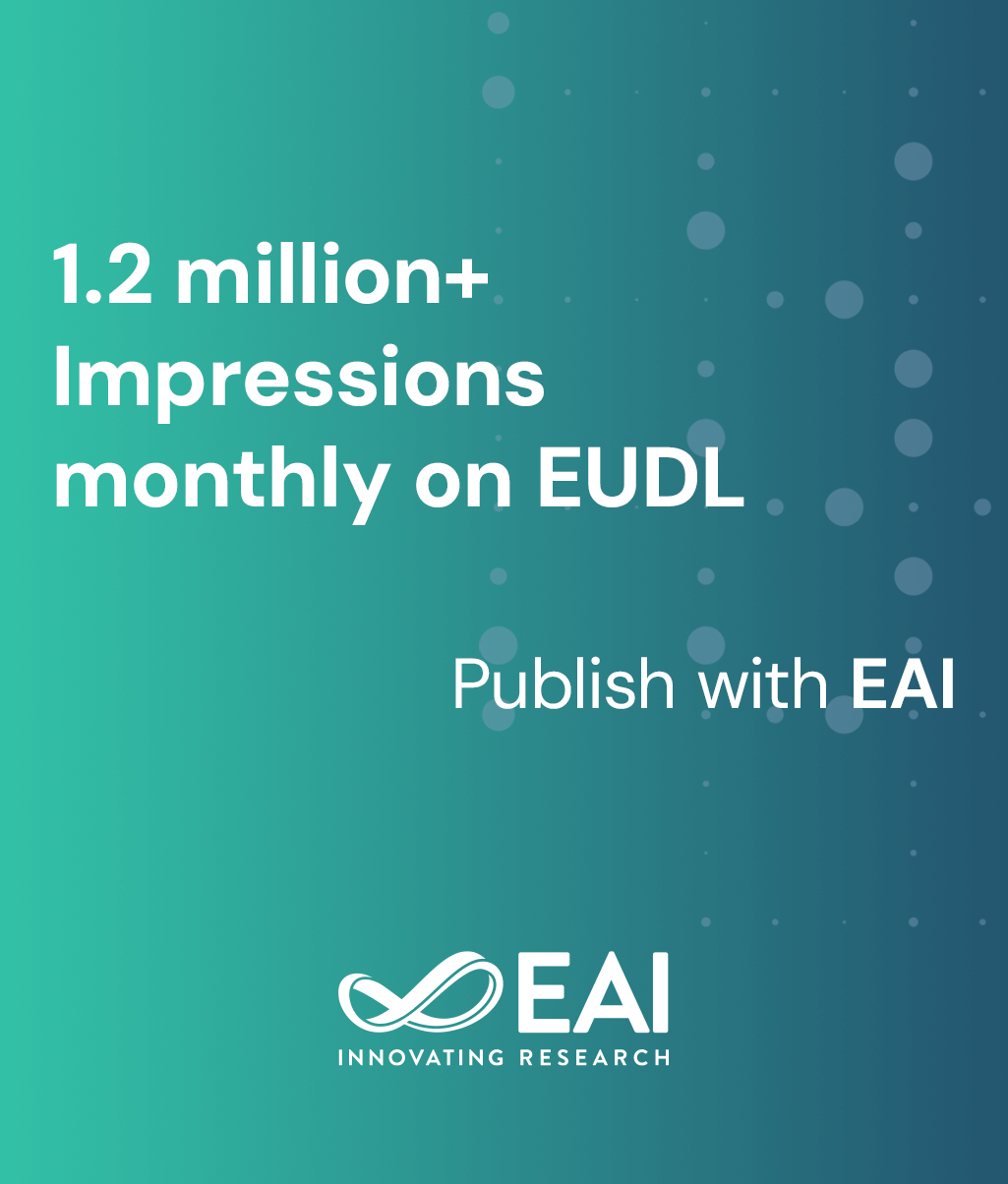
Research Article
Proactive Job Scheduling and Migration using Artificial Neural Networks for Volunteer Grid
@INPROCEEDINGS{10.4108/eai.27-2-2017.152277, author={Saddaf Rubab and Mohd Fadzil Hassan and Ahmad Kamil Mahmood and Syed Nasir Mehmood Shah}, title={Proactive Job Scheduling and Migration using Artificial Neural Networks for Volunteer Grid}, proceedings={First EAI International Conference on Computer Science and Engineering}, publisher={EAI}, proceedings_a={COMPSE}, year={2017}, month={3}, keywords={Volunteer grid job scheduling job migration artificial neural networks job failure resources}, doi={10.4108/eai.27-2-2017.152277} }
- Saddaf Rubab
Mohd Fadzil Hassan
Ahmad Kamil Mahmood
Syed Nasir Mehmood Shah
Year: 2017
Proactive Job Scheduling and Migration using Artificial Neural Networks for Volunteer Grid
COMPSE
EAI
DOI: 10.4108/eai.27-2-2017.152277
Abstract
A desktop grid is heterogeneous collections of local and volunteer resources. These resources can be assigned to heterogeneous jobs whereas these resources cannot be guaranteed to be available every time of job execution. Therefore, the resource availability and load forecast can help to minimize the job failures and job migration. In this paper, a forecast based proactive job scheduling and migration (PJS-ANN) has been proposed using artificial neural networks to make load forecasts for scheduling the jobs to reliable volunteer resources. The proposed method performance has been compared with conventional load balancing (LB) and no-migration (NM) algorithms. The performance comparisons demonstrate that the PJS-ANN has lower turnaround time per job and job failure rate has been significantly improved.