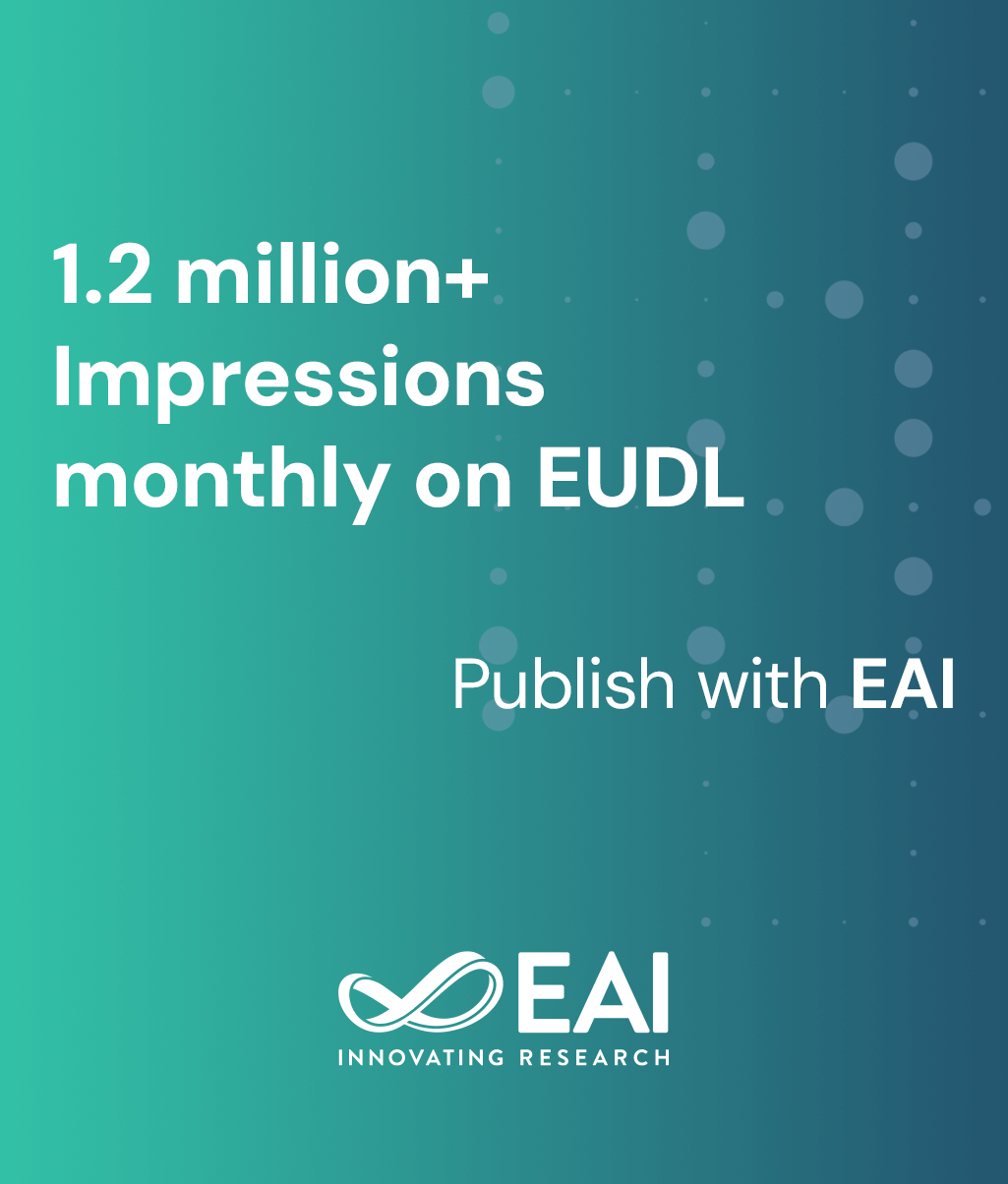
Research Article
Application of EEG-based Machine Learning in Stock Trading-related Emotion Recognition
@INPROCEEDINGS{10.4108/eai.27-10-2023.2342016, author={Mingliang Zuo and Bingbing Yu and Li Sui}, title={Application of EEG-based Machine Learning in Stock Trading-related Emotion Recognition}, proceedings={Proceedings of the 4th International Conference on Economic Management and Big Data Applications, ICEMBDA 2023, October 27--29, 2023, Tianjin, China}, publisher={EAI}, proceedings_a={ICEMBDA}, year={2024}, month={1}, keywords={emotion recognition machine learning stock valence-arousal eeg dwt sgd nb lda knn}, doi={10.4108/eai.27-10-2023.2342016} }
- Mingliang Zuo
Bingbing Yu
Li Sui
Year: 2024
Application of EEG-based Machine Learning in Stock Trading-related Emotion Recognition
ICEMBDA
EAI
DOI: 10.4108/eai.27-10-2023.2342016
Abstract
This paper develops a stock emotion recognition system based on a valence/arousal model using electroencephalogram (EEG) signals. The dataset is collected from participants who engage in paper trading using real stock market data, virtual currencies, and emotional outputs. The dataset contains five frequency bands, features such as differential entropy (DE), differential asymmetry (DASM), and rational asymmetry (RASM). Feature selection is performed using mutual information-based filtering combined with chi-square statistics and embedded algorithms in deep learning classifiers. Stock sentiment classification uses established machine learning models, stochastic gradient descent (SGD), linear discriminant analysis (LDA), K nearest neighbor (KNN) and naive Bayes (NB) algorithms. Subsequently, a comprehensive performance analysis and comparative evaluation of each classification algorithm are conducted. Notably, the K-nearest neighbor (KNN) method achieves remarkable accuracy rates of 89.2% for arousal and 94.59% for valence. These results highlight its exceptional performance when compared to pre-existing algorithms applied to stock emotion datasets.