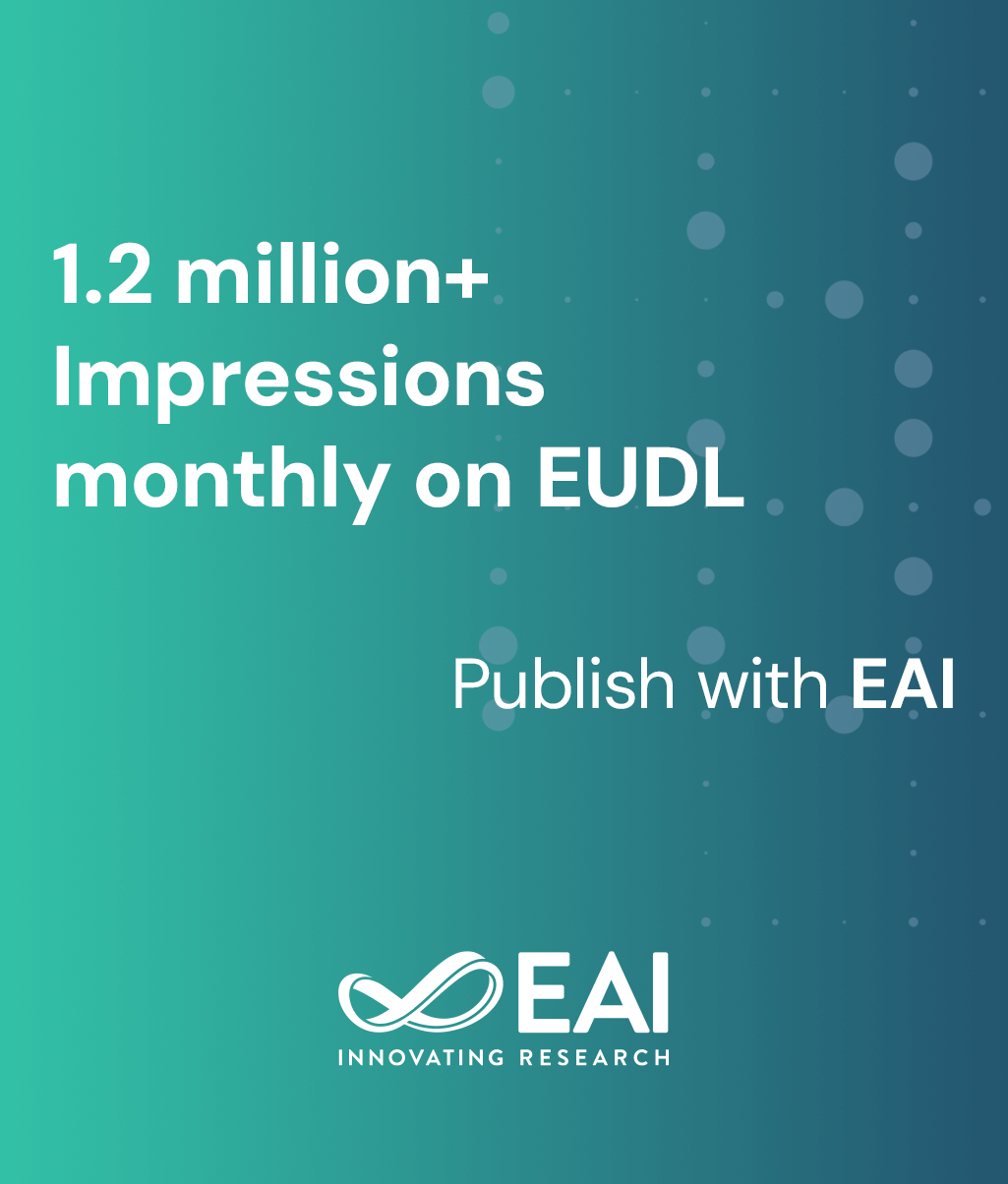
Research Article
Comparison of Deep Q-Learning Network and Double Deep Q-Learning Network for Trading Strategy
@INPROCEEDINGS{10.4108/eai.27-10-2023.2341940, author={Yang Chen}, title={Comparison of Deep Q-Learning Network and Double Deep Q-Learning Network for Trading Strategy}, proceedings={Proceedings of the 4th International Conference on Economic Management and Big Data Applications, ICEMBDA 2023, October 27--29, 2023, Tianjin, China}, publisher={EAI}, proceedings_a={ICEMBDA}, year={2024}, month={1}, keywords={deep q-learning network double deep q-learning network trading policy}, doi={10.4108/eai.27-10-2023.2341940} }
- Yang Chen
Year: 2024
Comparison of Deep Q-Learning Network and Double Deep Q-Learning Network for Trading Strategy
ICEMBDA
EAI
DOI: 10.4108/eai.27-10-2023.2341940
Abstract
This study introduces a unique program designed to provide a specialized trading environment for the purpose of training agents. Two principal agents, namely Deep Q-Leaning Network (DQN) and Double DQN (DDQN), were taught and afterwards evaluated, with their performance measured using profit metrics, providing insights into their effectiveness in formulating stock policies. The comparative examination of these agents, based on their respective training periods, consistently demonstrated the higher performance of DQN in comparison to DDQN. As for the progress into the future, there are several potential optimizations that might be considered. These optimizations encompass fine-tuning the elements of neural networks, extending the duration of training sessions, and integrating emerging brain designs and training paradigms. The suggested application demonstrates versatility and adaptability by offering opportunities to incorporate a wide range of financial market data sets. This feature holds the potential to provide a more comprehensive training environment and im-prove the trading techniques employed by the agents.