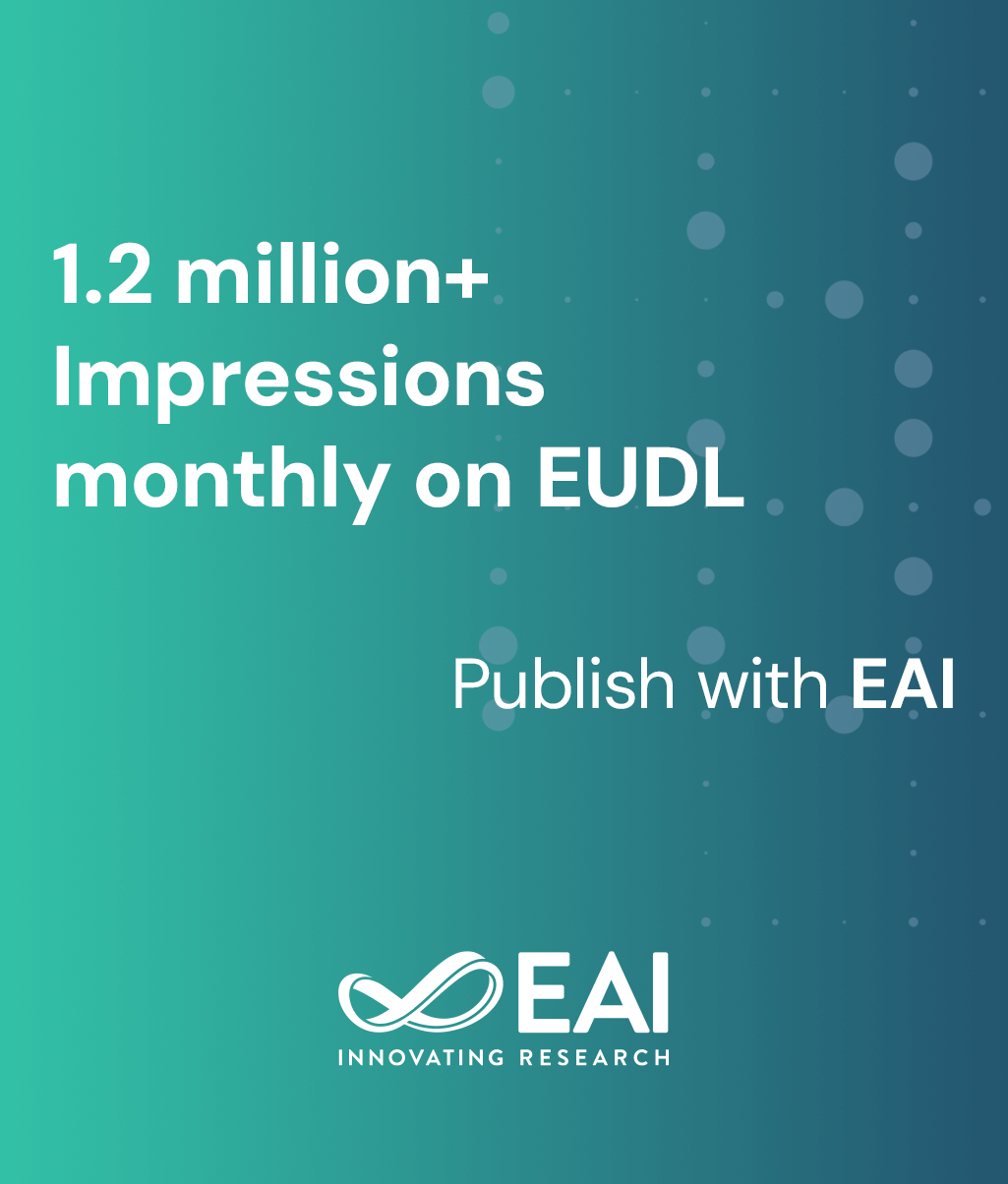
Research Article
Stock Investors' Preferences on Stock Forum Topics Based on FNS-LDA2vec
@INPROCEEDINGS{10.4108/eai.27-10-2023.2341922, author={Ganglong Duan and Jinkai Zhang and Mingyue Jiang}, title={Stock Investors' Preferences on Stock Forum Topics Based on FNS-LDA2vec}, proceedings={Proceedings of the 4th International Conference on Economic Management and Big Data Applications, ICEMBDA 2023, October 27--29, 2023, Tianjin, China}, publisher={EAI}, proceedings_a={ICEMBDA}, year={2024}, month={1}, keywords={share bar discussion; topic model; lda model; data mining;}, doi={10.4108/eai.27-10-2023.2341922} }
- Ganglong Duan
Jinkai Zhang
Mingyue Jiang
Year: 2024
Stock Investors' Preferences on Stock Forum Topics Based on FNS-LDA2vec
ICEMBDA
EAI
DOI: 10.4108/eai.27-10-2023.2341922
Abstract
This article constructs an investor topic preference mining model through stock bar text data and the FNS-LDA2vec method. Firstly, dynamic topic mining of the LDA model is achieved by dividing user documents in the stock community by time. Then, by combining the improved LDA topic model and the Word2vec word vector model, a dynamic mining model of stock bar user topic preference based on FNS-LDA2vec is constructed, and topic representation is learned through the joint learning of document vectors and word vectors. Finally, empirical results show that the topic extraction model constructed in this article is superior to the comparison model. The model has broad application value in personalized recommendation for investors and stock prediction.