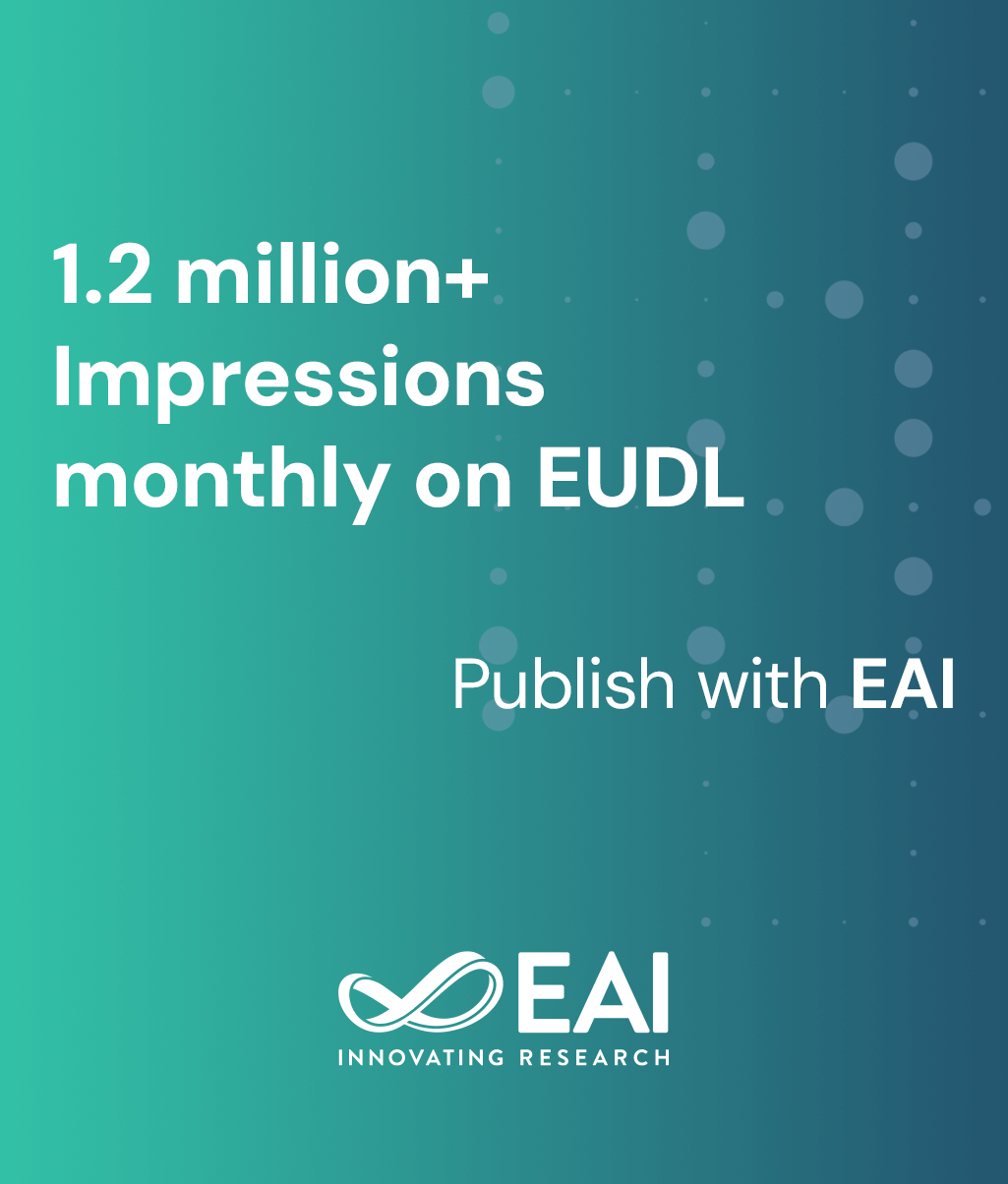
Research Article
Spatiotemporal Data Mining of Real Estate Registration
@INPROCEEDINGS{10.4108/eai.27-10-2023.2341903, author={Yidong Hu and Fenglei Mei and Chenling Pan and Tao Nie}, title={Spatiotemporal Data Mining of Real Estate Registration}, proceedings={Proceedings of the 4th International Conference on Economic Management and Big Data Applications, ICEMBDA 2023, October 27--29, 2023, Tianjin, China}, publisher={EAI}, proceedings_a={ICEMBDA}, year={2024}, month={1}, keywords={spatiotemporal data mining; real estate registration; clustering; spatial distribution}, doi={10.4108/eai.27-10-2023.2341903} }
- Yidong Hu
Fenglei Mei
Chenling Pan
Tao Nie
Year: 2024
Spatiotemporal Data Mining of Real Estate Registration
ICEMBDA
EAI
DOI: 10.4108/eai.27-10-2023.2341903
Abstract
Real estate registration is an important part of the field of natural resource management. Mining the value of registration data can help to improve the government's real estate asset management level, optimize urban planning and land use strategies. The data of real estate registration includes multi-dimensional information such as the right holder, area of property right, registration time and space location. Using registration data mining real estate registration space-time value can reveal the economic law of real estate transfer. Taking the transfer registration of existing houses in Jiang'an District of Wuhan as an example, analyze the spatial distribution characteristics from two spatial dimensions: natural buildings and cadastral sub-areas.We use econometrics and GIS spatial analysis methods to explore the overall spatial distribution of real estate through global autocorrelation, and analyze regional distribution characteristics through local autocorrelation. And researched the spatial clustering of cadastral sub-areas to study the spatial differentiation. Research has shown that dual factor clustering is more accurate in characterizing spatial clustering patterns and features than single factor clustering.