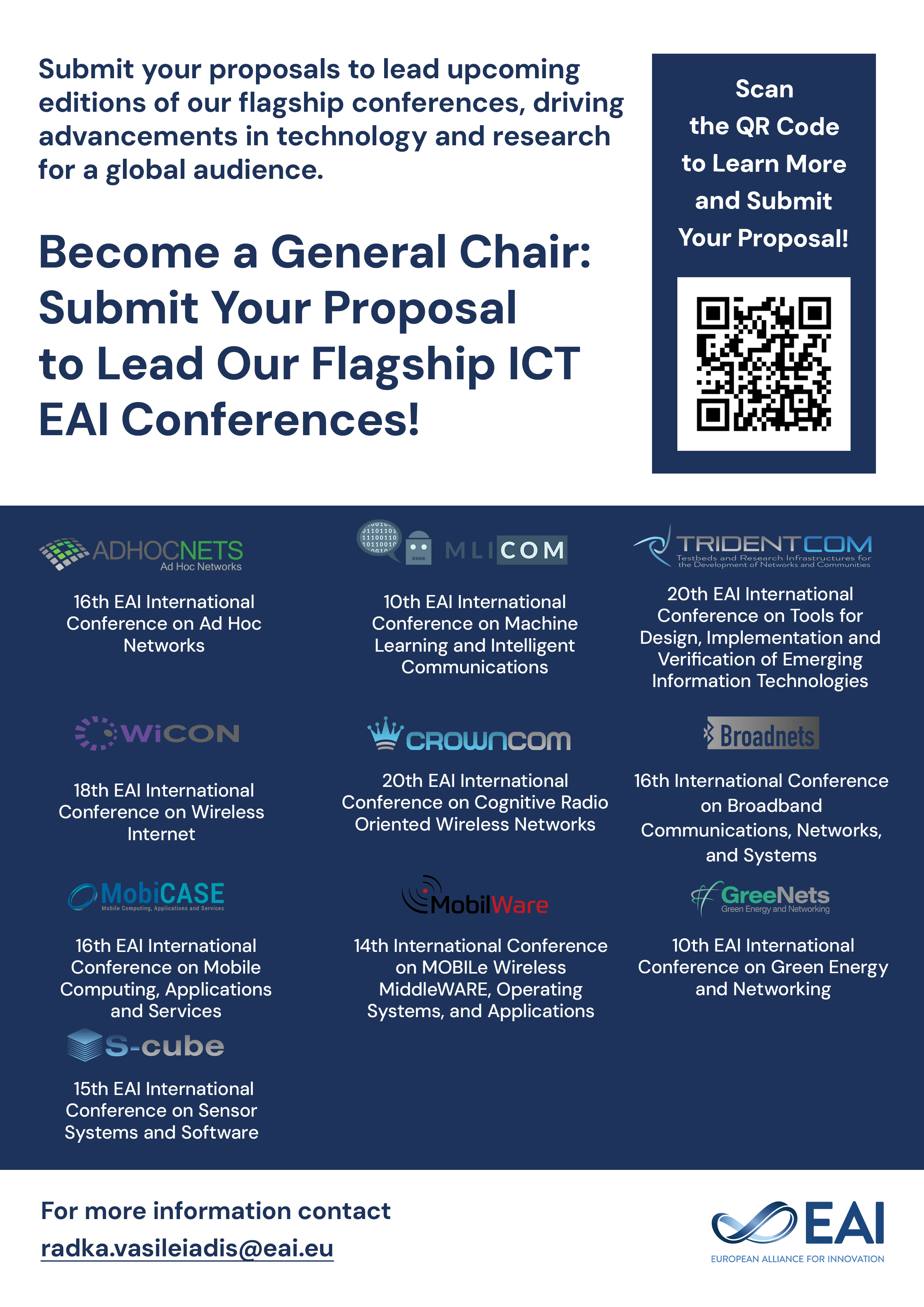
Research Article
Multi-Relational Graph Neural Networks Based Model Prediction for Subjective Well-Being of Middle-aged and Elderly People
@INPROCEEDINGS{10.4108/eai.26-5-2023.2337310, author={Yong Li and Di Wang and Xuan Sun}, title={Multi-Relational Graph Neural Networks Based Model Prediction for Subjective Well-Being of Middle-aged and Elderly People}, proceedings={Proceedings of the 4th International Conference on Education, Knowledge and Information Management, ICEKIM 2023, May 26--28, 2023, Nanjing, China}, publisher={EAI}, proceedings_a={ICEKIM}, year={2023}, month={9}, keywords={middle-aged and elderly; subjective well-being; deep learning; multi-relational graph neural networks; prediction}, doi={10.4108/eai.26-5-2023.2337310} }
- Yong Li
Di Wang
Xuan Sun
Year: 2023
Multi-Relational Graph Neural Networks Based Model Prediction for Subjective Well-Being of Middle-aged and Elderly People
ICEKIM
EAI
DOI: 10.4108/eai.26-5-2023.2337310
Abstract
happiness is the basis for measuring the mental health and quality of life of middle-aged and elderly people. Traditional research methods based on machine learning models are low complexity and poor universality, leading to inaccurate results, deep learning convenient structural information among multiple relationships, which is conducive to improving the accuracy of happiness prediction. In this paper, we pro-pose DL-GNN, a Multi-Relational Graph Neural Networks based Subjective Well-Being prediction model for middle-aged and elderly people. First, based on the CHARLS dataset, we construct the relational graph structure of the Subjective Well-Being of middle-aged and elderly people in terms of life satisfaction and depression; Second, we use a combination of Fully-Connected Network and Linear Regularization method to calculate the similarity of labels between nodes and select the similarity neighbor by neighbor sampling and similarity adaptive measurement to Finally, the at-tention mechanism is introduced to aggregate all the neighbor information within and between relations respectively, and the representation of target nodes is obtained through the layer-by-layer embedding between relations, which finally completes the construction of the DL-GNN model. The experiments show that the F1 score of DL-GNN model is 90.25% on the CHARLS dataset, the F1 score was improved by 4.78%,8.71%,10.93%and14.11% when compared with SDCN, GCN, XGBoost, and MLP, respectively.