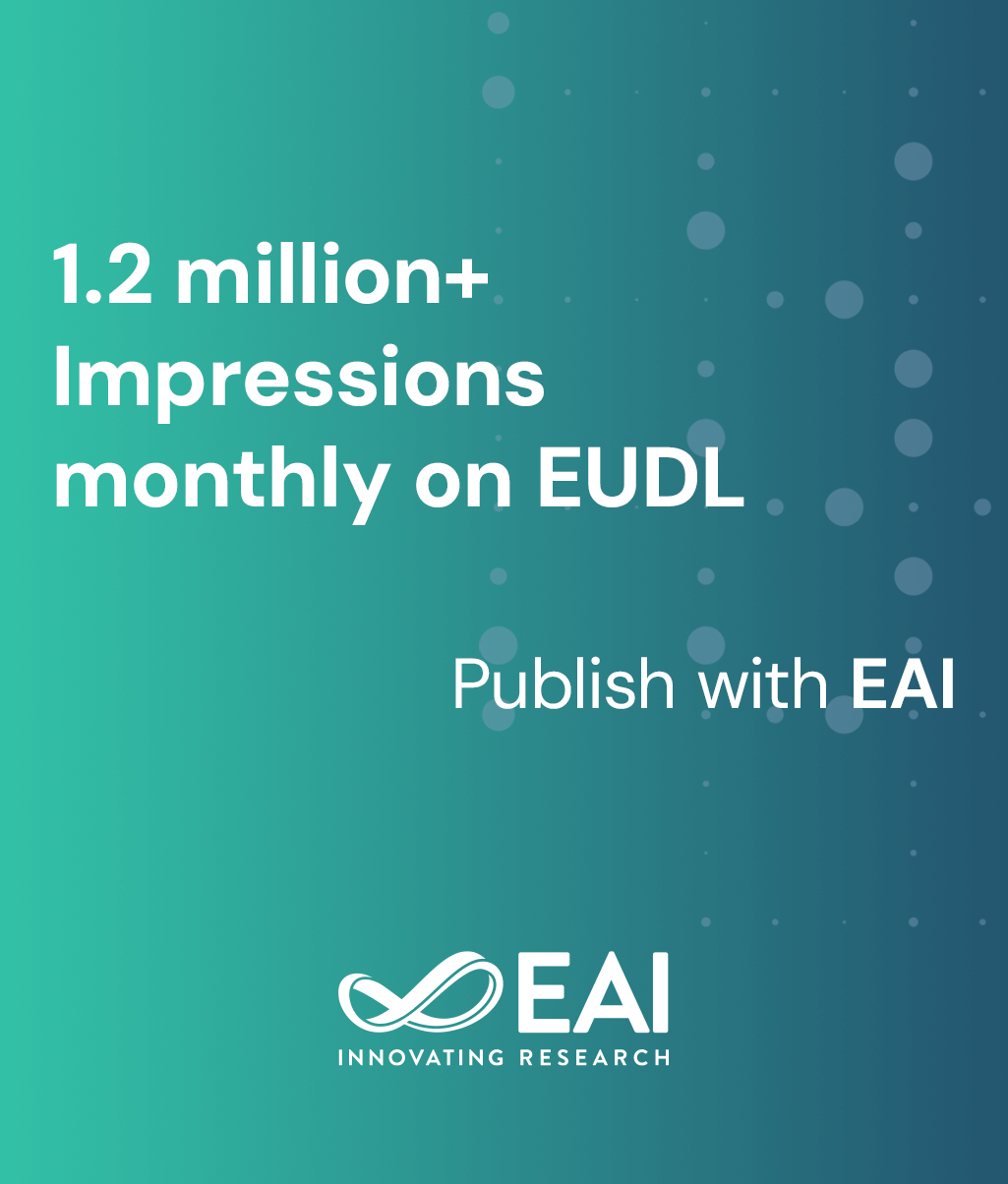
Research Article
Research and Application of Investment Risk Identification Based on Probability-Deep Neural Network
@INPROCEEDINGS{10.4108/eai.26-5-2023.2334474, author={Yuheng Sha and Qian Ma and Chao Xu and Xue Tan and Jun Yan and Yuqian Zhang}, title={Research and Application of Investment Risk Identification Based on Probability-Deep Neural Network}, proceedings={Proceedings of the 2nd International Conference on Mathematical Statistics and Economic Analysis, MSEA 2023, May 26--28, 2023, Nanjing, China}, publisher={EAI}, proceedings_a={MSEA}, year={2023}, month={7}, keywords={project risk identification; text classification; neural network; risk decision matrix; grid investment}, doi={10.4108/eai.26-5-2023.2334474} }
- Yuheng Sha
Qian Ma
Chao Xu
Xue Tan
Jun Yan
Yuqian Zhang
Year: 2023
Research and Application of Investment Risk Identification Based on Probability-Deep Neural Network
MSEA
EAI
DOI: 10.4108/eai.26-5-2023.2334474
Abstract
As the scale of power grid construction continues to expand, the scale of investment is also increasing, and investment risk factors are becoming more and more complex. To ensure the efficiency of power grid investment, it is necessary to identify power grid investment risks and formulate relevant preventive measures. Firstly, it uses natural language processing (NLP) and term frequency- inverse document frequency (TF-IDF) method to preprocess the text. Secondly, probabilistic neural network (PNN) is used to divide text materials into five kinds. Finally, back-propagation (BP) is used to evaluate the risk level of each text, achieving the risk category and risk level of the project investment. Furthermore, on this basis, the investment risk decision matrix is drawn and the investment risk response strategies are put forward, and an effective way for the practice of investment risk management in power grid projects are provided.