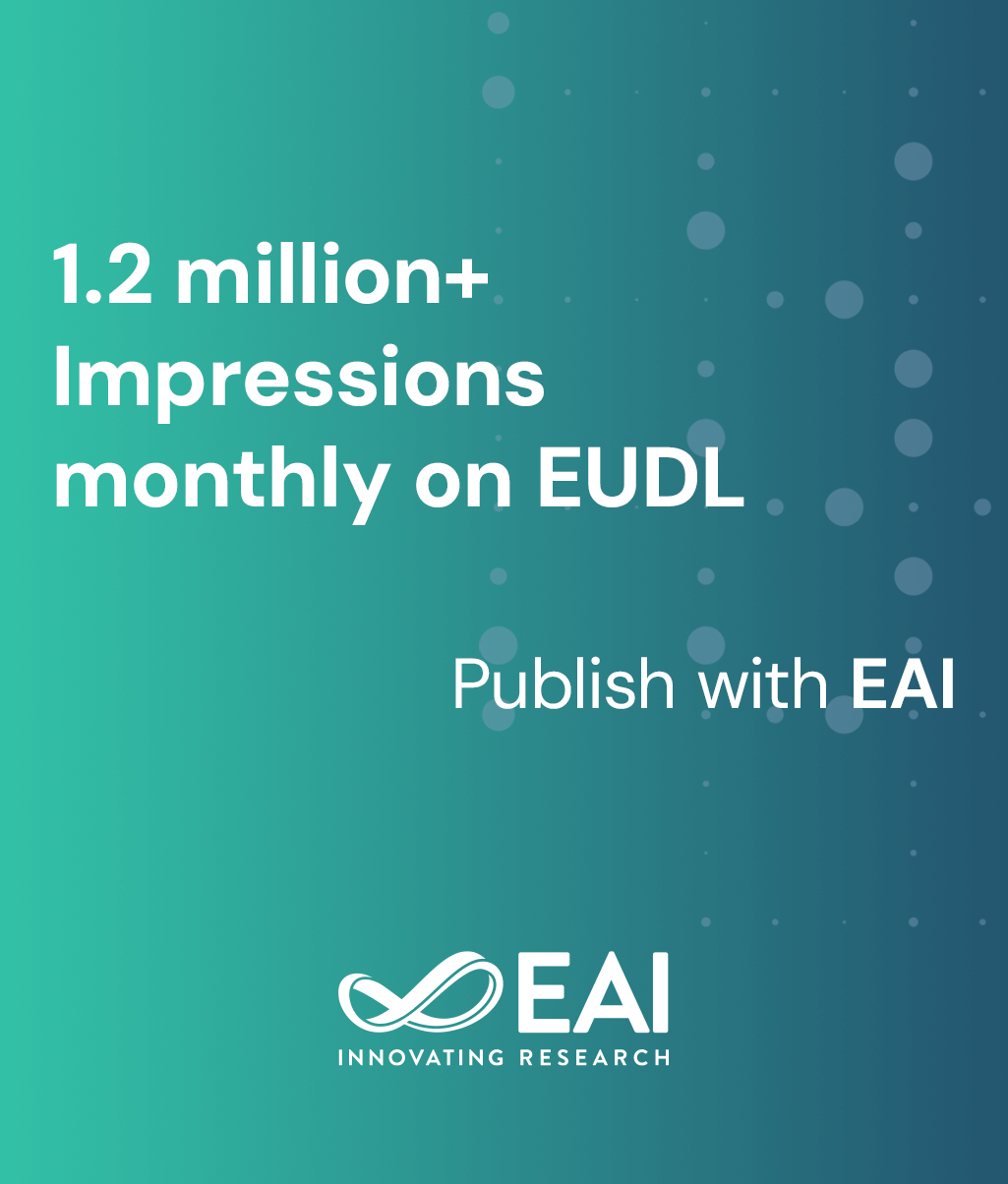
Research Article
Enhancing Beer Recommendations through Clustering: A Comparison of Hierarchical and K-means Clustering Methods on Normalized Data
@INPROCEEDINGS{10.4108/eai.26-5-2023.2334332, author={Tiansheng Zhu and Yina Han}, title={Enhancing Beer Recommendations through Clustering: A Comparison of Hierarchical and K-means Clustering Methods on Normalized Data}, proceedings={Proceedings of the 2nd International Conference on Mathematical Statistics and Economic Analysis, MSEA 2023, May 26--28, 2023, Nanjing, China}, publisher={EAI}, proceedings_a={MSEA}, year={2023}, month={7}, keywords={hierarchical method k-means clustering method beer recommendations data-driven approache}, doi={10.4108/eai.26-5-2023.2334332} }
- Tiansheng Zhu
Yina Han
Year: 2023
Enhancing Beer Recommendations through Clustering: A Comparison of Hierarchical and K-means Clustering Methods on Normalized Data
MSEA
EAI
DOI: 10.4108/eai.26-5-2023.2334332
Abstract
The process of normalization confers notable advantages in optimizing gradient descent algorithms for machine learning applications and simplifying data processing. Through normalization, the scale of numeric types in the dataset can be adjusted, thereby facilitating the identification of patterns and trends that would otherwise remain obscured by variations in the magnitude of the features. This technique also reduces the risk of certain features having a disproportionate influence on the model's decision-making process. The scale function is one such means of normalization that effectively mitigates such issues.In the context of clustering, normalization plays a crucial role in enhancing the accuracy and efficiency of the process. It is imperative to note that in the case of an unclassified dataset, where the distribution of data is unknown, unsupervised learning models must be employed. Unsupervised learning models enable the identification of patterns and structures within the dataset without the need for prior classification. Clustering is one such approach that can extract meaningful insights from unstructured datasets.Through clustering data, the present research offer practical insights for businesses seeking to leverage data-driven approaches to enhance their operations and improve customer satisfaction.