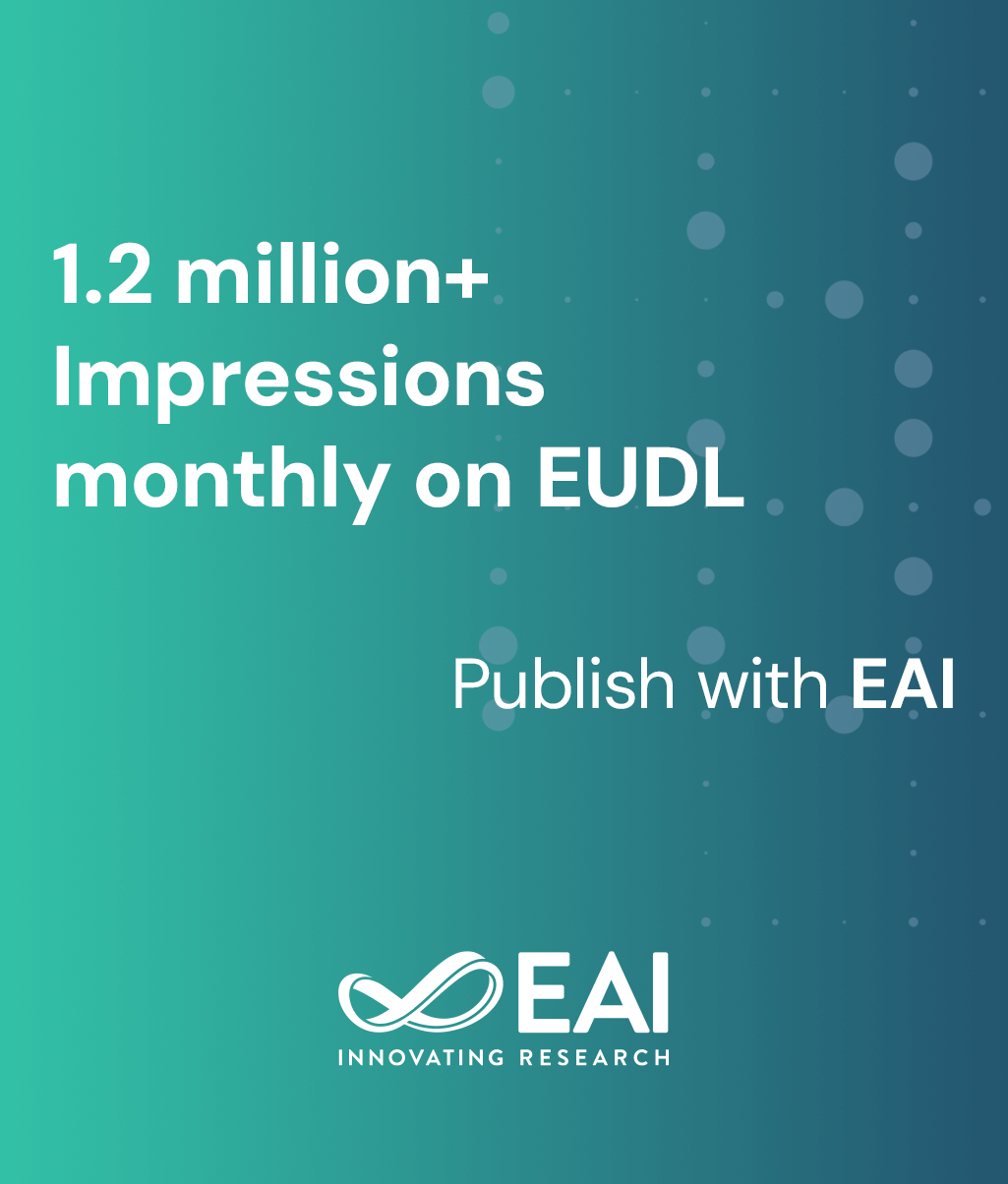
Research Article
Railway Freight Volume Prediction Based on SSA-BP
@INPROCEEDINGS{10.4108/eai.26-5-2023.2334263, author={Jiajun Luo and Juan He and Jiayun Zheng and Sijie Cheng}, title={Railway Freight Volume Prediction Based on SSA-BP}, proceedings={Proceedings of the 2nd International Conference on Mathematical Statistics and Economic Analysis, MSEA 2023, May 26--28, 2023, Nanjing, China}, publisher={EAI}, proceedings_a={MSEA}, year={2023}, month={7}, keywords={railway transportation; sparrow search algorithm; ssa-bp; freight volume forecasting}, doi={10.4108/eai.26-5-2023.2334263} }
- Jiajun Luo
Juan He
Jiayun Zheng
Sijie Cheng
Year: 2023
Railway Freight Volume Prediction Based on SSA-BP
MSEA
EAI
DOI: 10.4108/eai.26-5-2023.2334263
Abstract
In order to accurately predict the railway freight volume, this paper proposes a railway freight volume prediction method based on Sparrow search algorithm (SSA) optimized Back Propagation (BP) neural network. This method utilizes the characteristics of fast convergence speed and strong optimization ability of SSA to optimize the initial threshold and weight of BP neural network, and effectively overcomes the problem that BP neural network is easy to fall into local optimization. Taking the railway freight volume data of China from 2001 to 2020 as the research object, the grey correlation analysis method is used to select the relevant influencing factors, and then the SSA-BP model is established to fit and predict the railway freight volume, and the prediction is compared with the traditional BP neural network and PSO-BP model. The example analysis shows that the MAPE value of SSA-BP model is 2.03%, which is the minimum of the models used. The prediction accuracy of SSA-BP model is better than that of BP neural network and PSO-BP network model, and SSA has faster convergence speed and better optimization effect than PSO.