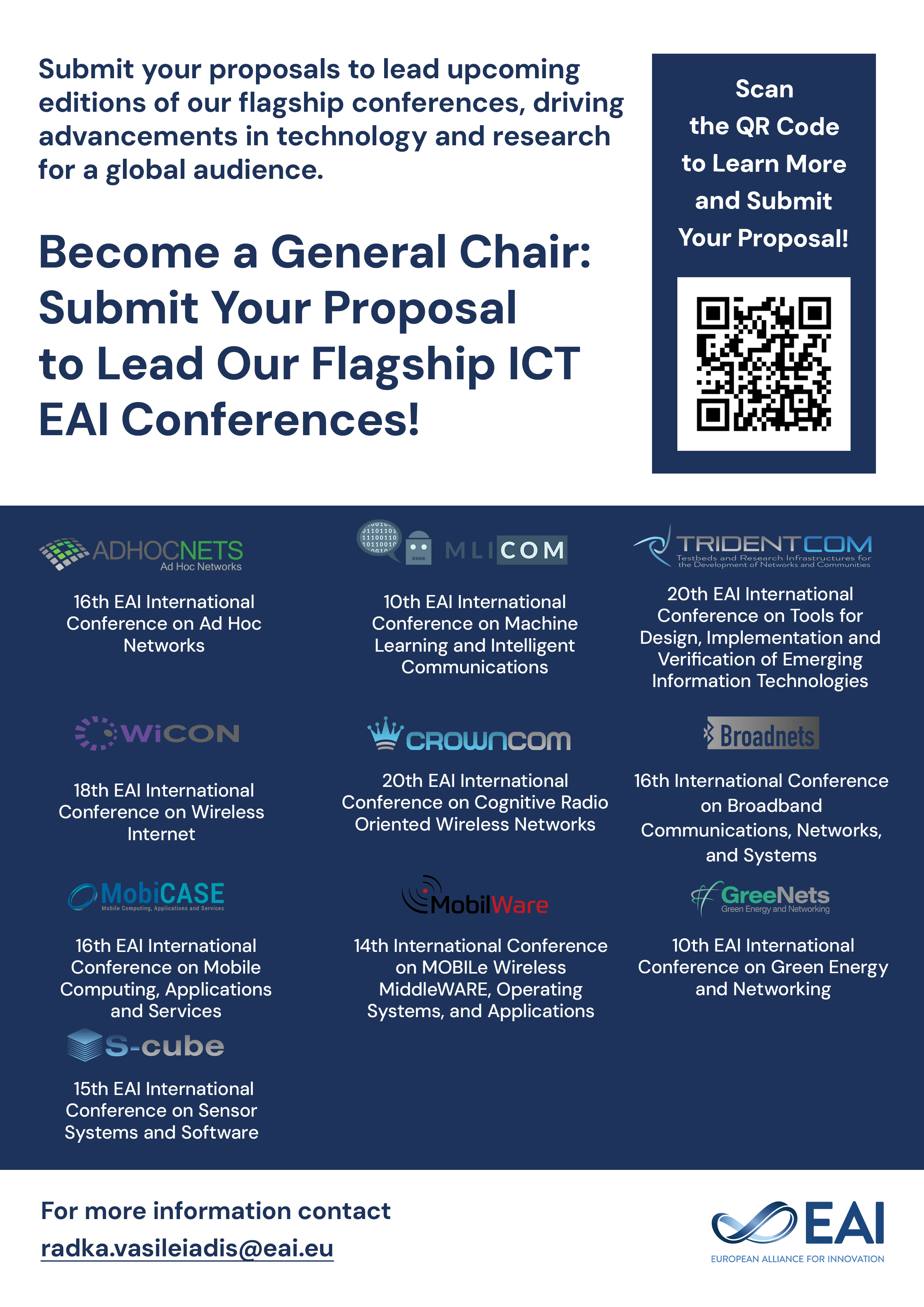
Research Article
A Self-Learning Energy Management System for a Smart-Grid-Ready Residential Building
@INPROCEEDINGS{10.4108/eai.24-8-2015.2261068, author={Fabiano Pallonetto and Yerlan Turenshenko and Eleni Mangina and Donal Finn}, title={A Self-Learning Energy Management System for a Smart-Grid-Ready Residential Building}, proceedings={1st EAI International Workshop on Energyaware Simulation}, publisher={ACM}, proceedings_a={ENERGY-SIM}, year={2015}, month={8}, keywords={energy management system heating system energy consumption smart controller building simulation optimisation residential building}, doi={10.4108/eai.24-8-2015.2261068} }
- Fabiano Pallonetto
Yerlan Turenshenko
Eleni Mangina
Donal Finn
Year: 2015
A Self-Learning Energy Management System for a Smart-Grid-Ready Residential Building
ENERGY-SIM
ACM
DOI: 10.4108/eai.24-8-2015.2261068
Abstract
Based on research and scientic advances in sensor and net- work technologies, machine learning, and standard statis- tical methods, a development and a deployment of energy management systems could reduce the cost of electricity in residential buildings.This paper details two implementations of an energy management systems that can improve an ef- ciency of the energy consumption of a residential building and minimise the energy expenditure of it, while maintain the comfort temperature inside the house. The rst proto- type used a rule based control ow and reduced the baseline consumption by 25% whereas the smart version of energy management system reached almost 50% minimisation of consumption by predicting future changes in the house tem- perature via a tree based machine learning models generated in R language. This Smart Controller with these predictions and energy cost calculations makes decision to either turn on or o the heating system of the house. To test and eval- uate the system, both energy management systems run a virtual building simulation environment such as EnergyPlus through its interface controller BCVTB and RESTful API service that controls the building simulation software and stores obtained results to its database.