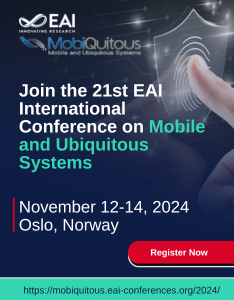
Research Article
Machine Learning-based Model to Predict Student's success in Higher Education
@INPROCEEDINGS{10.4108/eai.24-3-2022.2318766, author={Atul Garg and Nidhi Bansal Garg and Umesh Kumar Lilhore and Renu Popli and Sarita Simaiya and Ankit Bansal}, title={Machine Learning-based Model to Predict Student's success in Higher Education}, proceedings={Proceedings of the 3rd International Conference on ICT for Digital, Smart, and Sustainable Development, ICIDSSD 2022, 24-25 March 2022, New Delhi, India}, publisher={EAI}, proceedings_a={ICIDSSD}, year={2023}, month={5}, keywords={naive bayes k* students performance hei's machine learning big data}, doi={10.4108/eai.24-3-2022.2318766} }
- Atul Garg
Nidhi Bansal Garg
Umesh Kumar Lilhore
Renu Popli
Sarita Simaiya
Ankit Bansal
Year: 2023
Machine Learning-based Model to Predict Student's success in Higher Education
ICIDSSD
EAI
DOI: 10.4108/eai.24-3-2022.2318766
Abstract
Predictions are always helpful for making decisions. Students are the future of the world. Higher Education Institutions (HEI's) in developing countries cannot apply similar strategies to all the students. Academic achievement plays a crucial role in the academic system because it is often utilized for the educational establishment quality. Early identification of at-risk educators and prevention strategies can significantly improve their chances of succeeding. Education is affected by different environments, family backgrounds, social and personal responsibilities. In this research article students, performance is measured based on various parameters using Random Forest, Naive Bayes and K* method. Experimental analysis shows the strengthening of the random forest method over K* and Naive Bayes method.