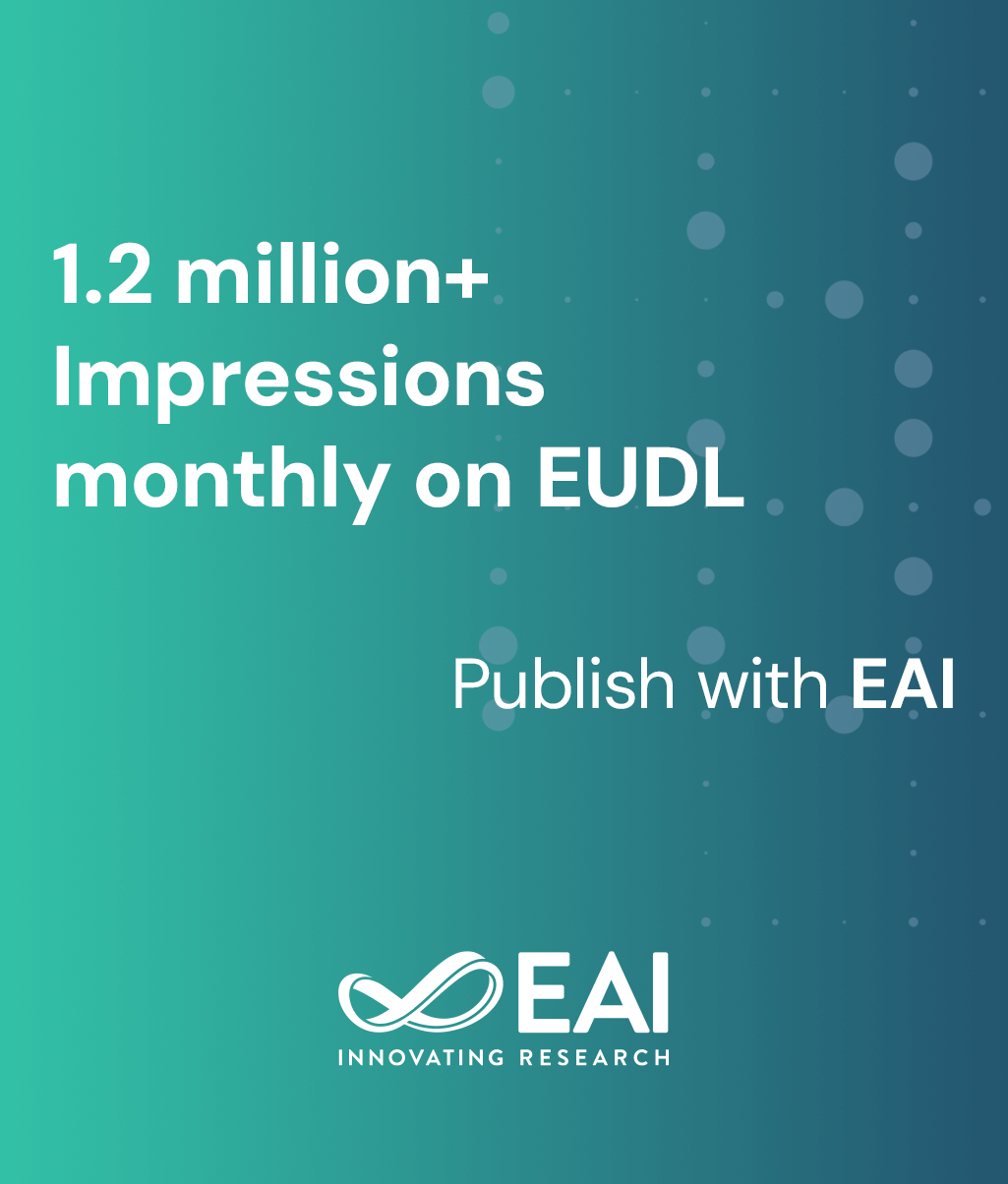
Research Article
Particulate matter forecasting using artificial neural network and support vector machine based models
@INPROCEEDINGS{10.4108/eai.24-3-2022.2318563, author={Adil Masood and Kafeel Ahmad}, title={Particulate matter forecasting using artificial neural network and support vector machine based models}, proceedings={Proceedings of the 3rd International Conference on ICT for Digital, Smart, and Sustainable Development, ICIDSSD 2022, 24-25 March 2022, New Delhi, India}, publisher={EAI}, proceedings_a={ICIDSSD}, year={2023}, month={5}, keywords={artificial neural network (ann); support vector machine (svm); pm10; roughness coefficient}, doi={10.4108/eai.24-3-2022.2318563} }
- Adil Masood
Kafeel Ahmad
Year: 2023
Particulate matter forecasting using artificial neural network and support vector machine based models
ICIDSSD
EAI
DOI: 10.4108/eai.24-3-2022.2318563
Abstract
Particulate matter (PM10) remains the most important air pollutant that plays a dominant negative role in deteriorating the air quality of Delhi and its surrounding regions. Accurate forecasting of this criteria pollutant is crucial for managing the air status and providing valuable information to the sensitive population groups. In this study, two machine learning-based techniques, i.e., Artificial neural network (ANN) and Support vector machine (SVM) were applied to predict PM10 using air quality and meteorological parameters as inputs. The data corresponding to a period of two years (2015-16) having 730 observations was used in this study. The model performances were assessed by R2, RMSE, and IA values. The results suggested that the ANN model with R2 = 0.896, RMSE = 46.6 and IA = 0.987, outperformed the SVM based model in terms of PM10 forecasting. Overall, the findings of this work highlighted that ANN is an accurate and reliable technique for forecasting PM10 concentrations.