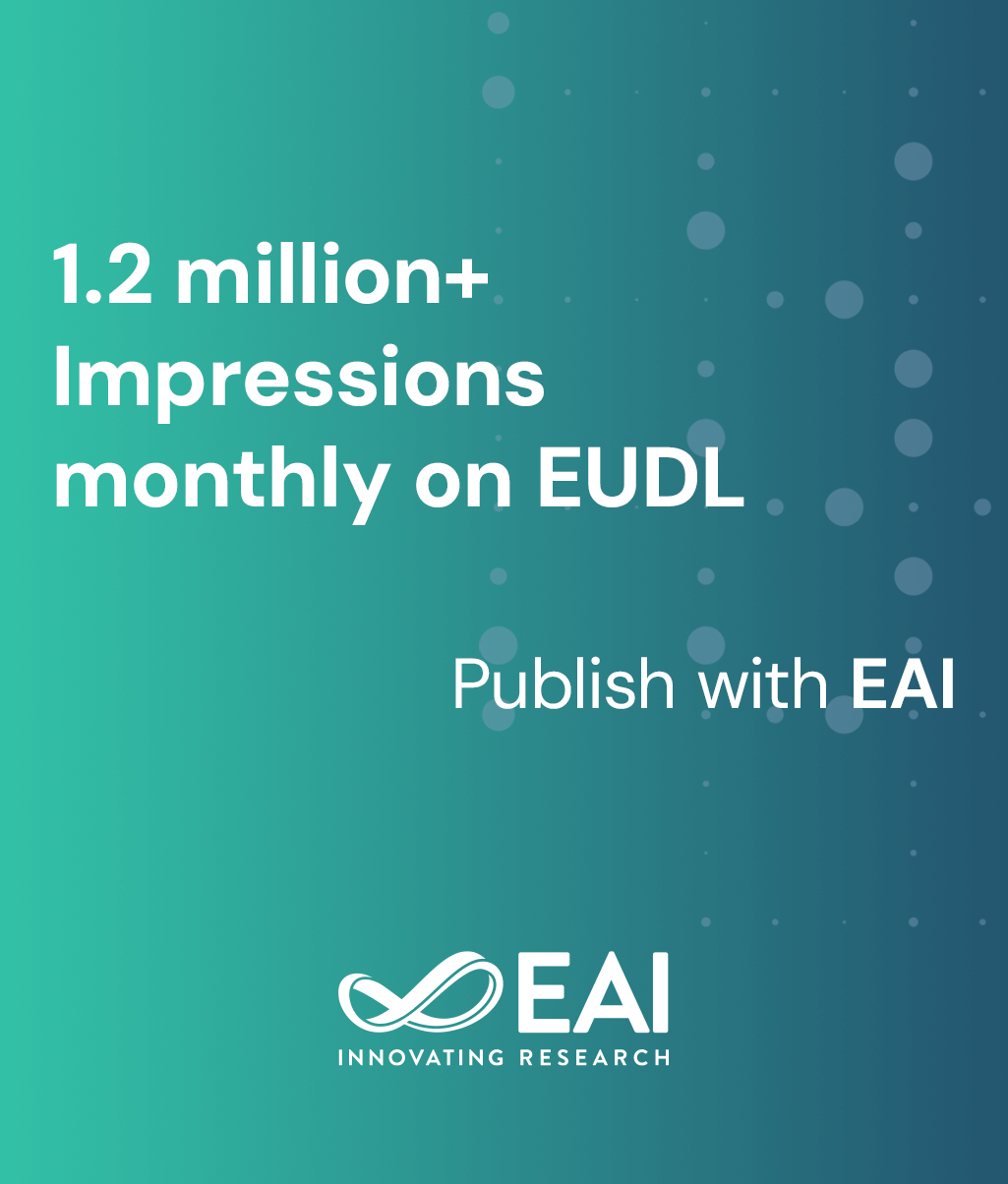
Research Article
Multiple Choice Question Generation Based on the Improved TextRank
@INPROCEEDINGS{10.4108/eai.24-11-2023.2343612, author={Lai Wei and Guosheng Hao and Xia Wang and Shuoshuo Meng and Xiaohan Yang and Yi Zhu}, title={Multiple Choice Question Generation Based on the Improved TextRank}, proceedings={Proceedings of the 3rd International Conference on Internet Technology and Educational Informatization, ITEI 2023, November 24--26, 2023, Zhengzhou, China}, publisher={EAI}, proceedings_a={ITEI}, year={2024}, month={4}, keywords={improved textrank; keywords extraction; mcq generation; question difficulty}, doi={10.4108/eai.24-11-2023.2343612} }
- Lai Wei
Guosheng Hao
Xia Wang
Shuoshuo Meng
Xiaohan Yang
Yi Zhu
Year: 2024
Multiple Choice Question Generation Based on the Improved TextRank
ITEI
EAI
DOI: 10.4108/eai.24-11-2023.2343612
Abstract
Currently, strategies for generating multiple choice questions (MCQ) seldom take the analysis of semantic and syntactic dependency features into consideration. A Chinese MCQ generation method is proposed based on the improved TextRank algorithm with semantic similarity and dependency relatedness to extract keywords, primarily entities, as knowledge points for MCQs. Verb weight is introduced to improve the accuracy of initial weight in keyword extraction to obtain knowledge points for MCQs more precisely in texts. Synonyms based on Word2Vec is used to generate distractors for MCQs, which are filtered to ensure that each distractor refers to a different entity. Experiments show that compared to human generated questions, the accuracy of identification is 59.5%, and the F_1 value is 0.58. The aspect of keyword extraction in the cloze questions generation task evaluation metrics shows some improvement. The calculated question difficulty exhibits a strong negative correlation with answer accuracy.