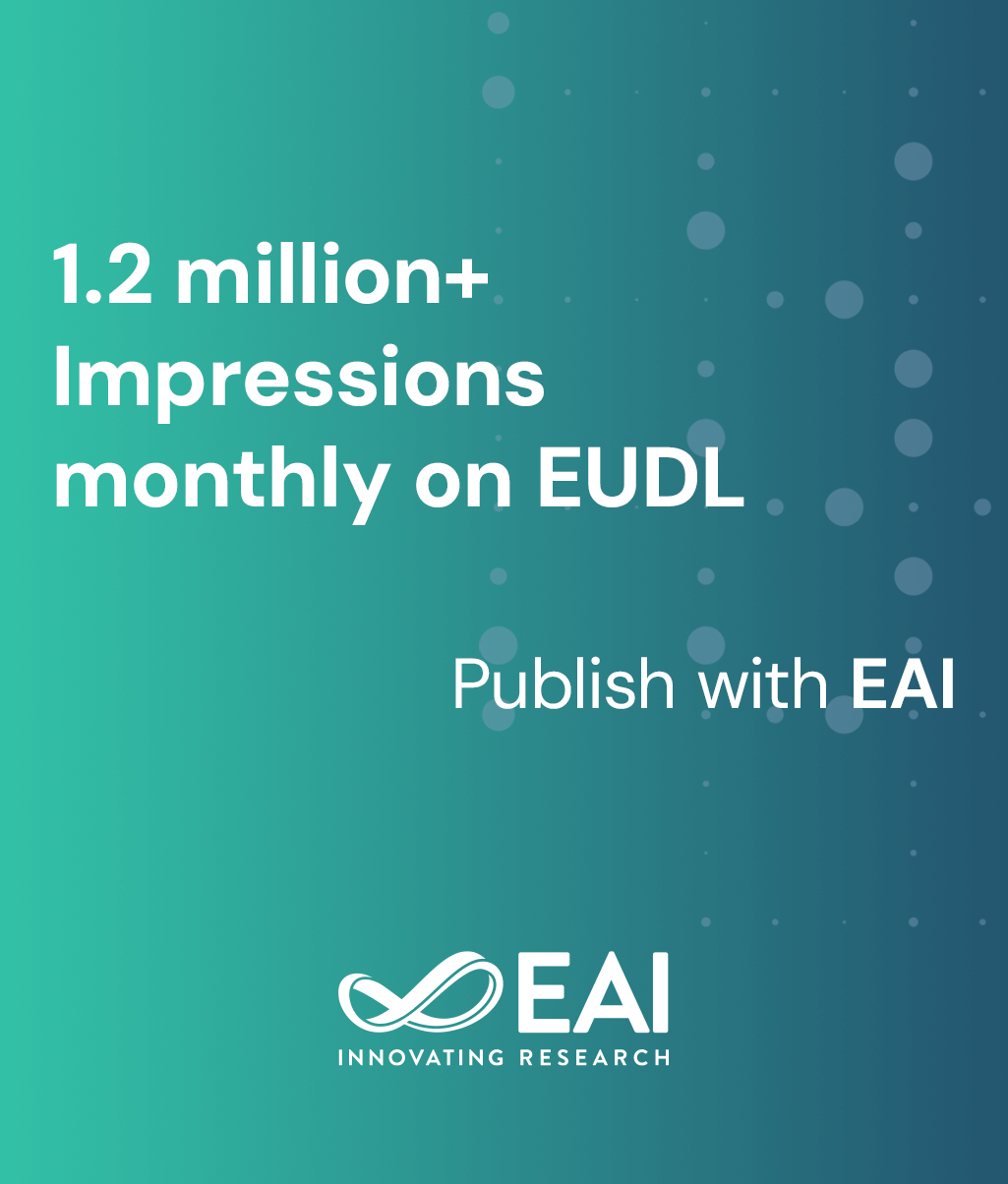
Research Article
Optimization of Feature Selection Using Genetic Algorithms to Increase Payment Delay Prediction Results (Subang Polytechnic State Case Study)
@INPROCEEDINGS{10.4108/eai.24-10-2018.2280507, author={Tri Herdiawan Apandi and Dwi Vernanda and Rian Piarna}, title={Optimization of Feature Selection Using Genetic Algorithms to Increase Payment Delay Prediction Results (Subang Polytechnic State Case Study)}, proceedings={The 1st International Conference on Computer Science and Engineering Technology Universitas Muria Kudus}, publisher={EAI}, proceedings_a={ICCSET}, year={2018}, month={11}, keywords={c45 algorithm cross validation confusion matrix polsub ukt}, doi={10.4108/eai.24-10-2018.2280507} }
- Tri Herdiawan Apandi
Dwi Vernanda
Rian Piarna
Year: 2018
Optimization of Feature Selection Using Genetic Algorithms to Increase Payment Delay Prediction Results (Subang Polytechnic State Case Study)
ICCSET
EAI
DOI: 10.4108/eai.24-10-2018.2280507
Abstract
The Indonesian government established a policy on tuition fees in higher education, namely the Single Tuition System (UKT), including the Subang State Polytechnic (POLSUB) using this system. Currently in POLSUB there are frequent delays in UKT payments, the number of delays is not much but continues to increase every semester. The purpose of this study is to classify students who are late and timely in making UKT payments using the C4.5 algorithm. Based on the comparison of evaluation and validation results from the four partitions shows that the data results from partitions 1 to 4 producing the best accuracy is on the 2nd partition has an accuracy rate of 75%. While the results using genetic algorithms improve accuracy results to 83.64%. In selecting the initial data collection feature, there are 13 features and after testing there are only 7 features that are most influential