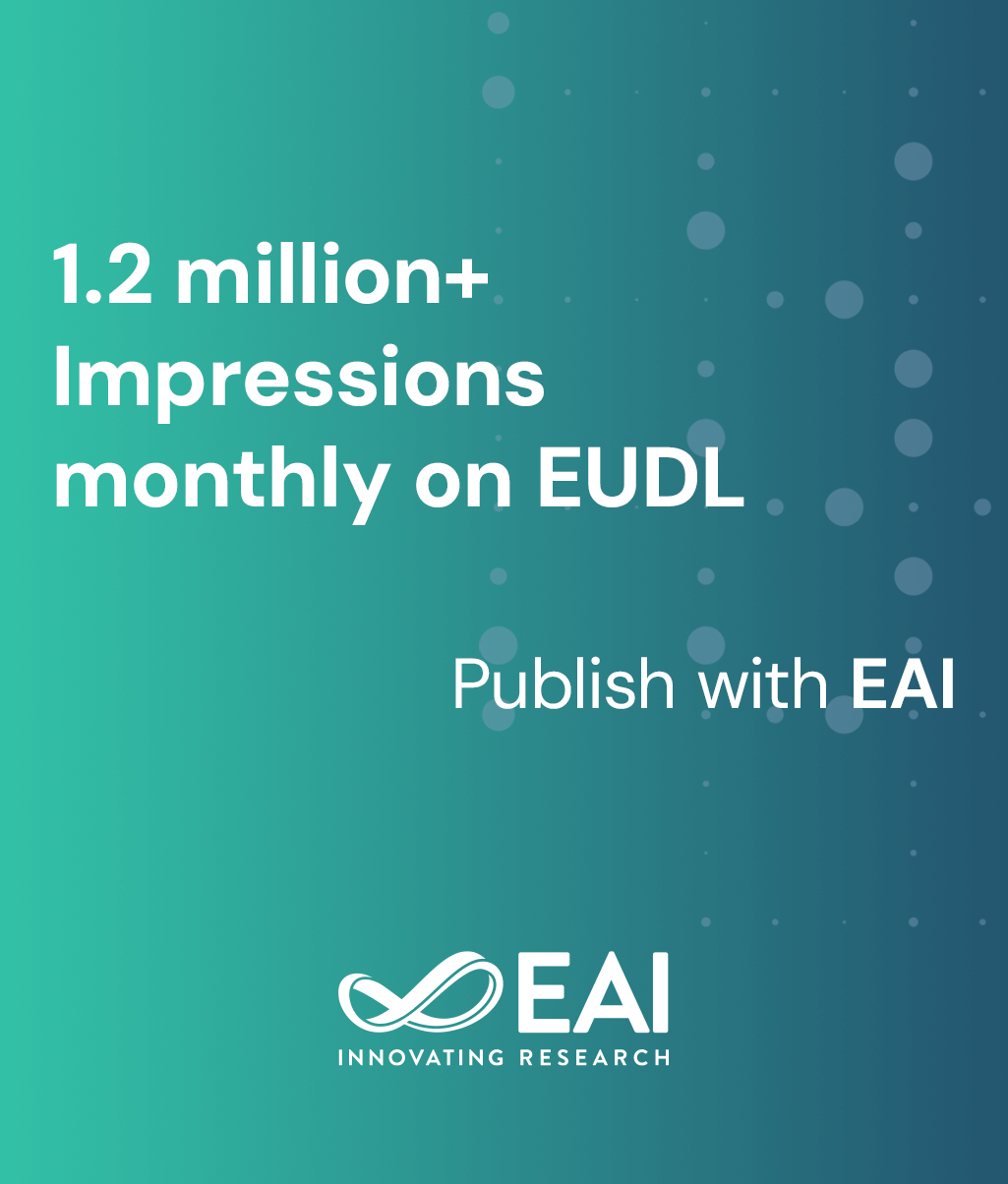
Research Article
Analysis of Student Demographic Information Using Data Mining classification with Decision Tree
@INPROCEEDINGS{10.4108/eai.23-4-2018.2277561, author={Citra Kurniawan and Ifit Novita Sari and Diah Puji Nali Brata}, title={Analysis of Student Demographic Information Using Data Mining classification with Decision Tree}, proceedings={Joint Workshop KO2PI and The 1st International Conference on Advance \& Scientific Innovation}, publisher={EAI}, proceedings_a={ICASI}, year={2018}, month={7}, keywords={data mining decision tree student demographic}, doi={10.4108/eai.23-4-2018.2277561} }
- Citra Kurniawan
Ifit Novita Sari
Diah Puji Nali Brata
Year: 2018
Analysis of Student Demographic Information Using Data Mining classification with Decision Tree
ICASI
EAI
DOI: 10.4108/eai.23-4-2018.2277561
Abstract
The purpose of this study was to process Student Demographic Information using data mining analysis using decision tree technique. This study retrieved data from 50 students with visual data attributes - verbal preferences, self-efficacy, gender, and interest. This study uses orange data mining to process data with decision tree technique. The study found that decision node as the best predictors are visual preferences, in which visuals had a 76% of 50 attributes. Female students had a 100% distribution as a visual preference, while male had 68.4% of the distribution as a visual preference. The results found that the attributes that predictor were visual preferences. The decision tree gets the rule from the root node to the leaf nodes as many as four rules are R1, R2, R3, and R4.