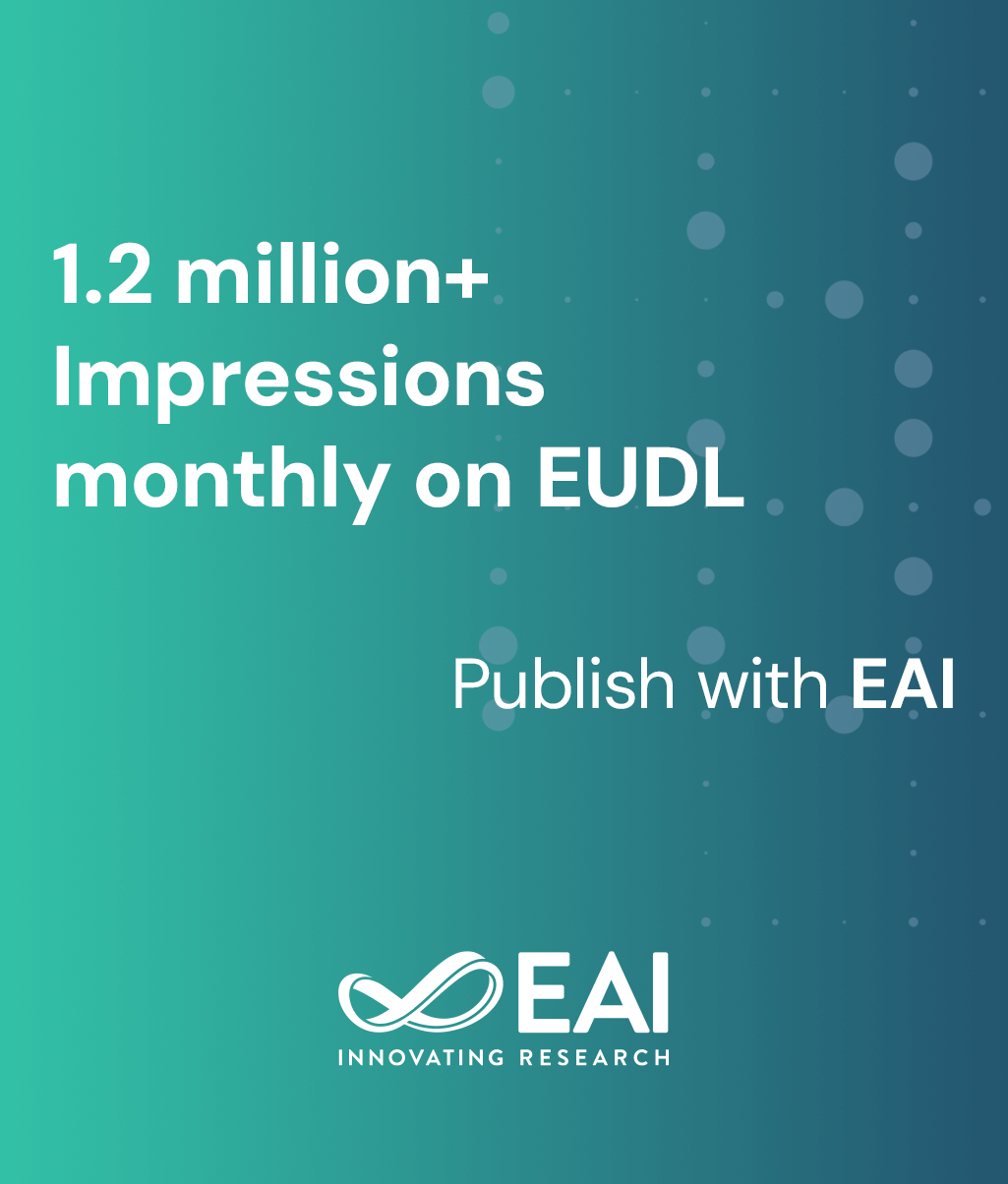
Research Article
Heart Disease Diagnosis Based on Deep Radial Basis Function Kernel Machine
@ARTICLE{10.4108/eai.23-3-2018.154774, author={Mashail Alsalamah and Saad Amin and Vasile Palade}, title={Heart Disease Diagnosis Based on Deep Radial Basis Function Kernel Machine}, journal={EAI Endorsed Transactions on Ambient Systems}, volume={5}, number={17}, publisher={EAI}, journal_a={AMSYS}, year={2018}, month={3}, keywords={machine Learning, Classification, Unsupervised Regression, Supervised Regression, Principal Component Analysis}, doi={10.4108/eai.23-3-2018.154774} }
- Mashail Alsalamah
Saad Amin
Vasile Palade
Year: 2018
Heart Disease Diagnosis Based on Deep Radial Basis Function Kernel Machine
AMSYS
EAI
DOI: 10.4108/eai.23-3-2018.154774
Abstract
Over the years Radial Basis Function (RBF) Kernel Machines have been used in Machine Learning tasks, but there are certain flaws that prevent their usage in some up-to-date applications (e.g., some Kernel Machines suffer from fast growth number of learning parameters whilst predicting data with large number of variations). Besides, Kernel Machines with single hidden layer have no mechanisms for features selection in multidimensional data space, and machine-learning task becomes intractable with enlargement of the data available for analysis. To address these issues, this paper investigates the usage of a framework for “deep learning” architecture composed of multilayered adaptive non-linear components – Multilayer RBF Kernel Machine. To be precise, three different approaches of features selection and dimensionality reduction to train RBF based on Multilayer Kernel Learning are explored, and comparisons between them in terms of accuracy, performance and computational complexity are made. As opposed to the “shallow learning” algorithm with usually single layer architecture, results show that the multilayered system produces better results with large and highly varied data. In particular, features selection and dimensionality reduction, as a class of the multilayer method, shows results that are more accurate. This paper proposes a novel scheme based on deep Multilayer RBF Kernel Machine learning for sleep apnea detection and quantification using statistical features of ECG signals. The results obtained show that the newly proposed approach provides significant accuracy improvements compared to state-of-the-art methods. Because of its noninvasive and low-cost nature, this algorithm has the potential for numerous applications in sleep medicine.
Copyright © 2018 Mashail Alsalamah et al., licensed to EAI. This is an open access article distributed under the terms of the Creative Commons Attribution licence (http://creativecommons.org/licenses/by/3.0/), which permits unlimited use, distribution and reproduction in any medium so long as the original work is properly cited.