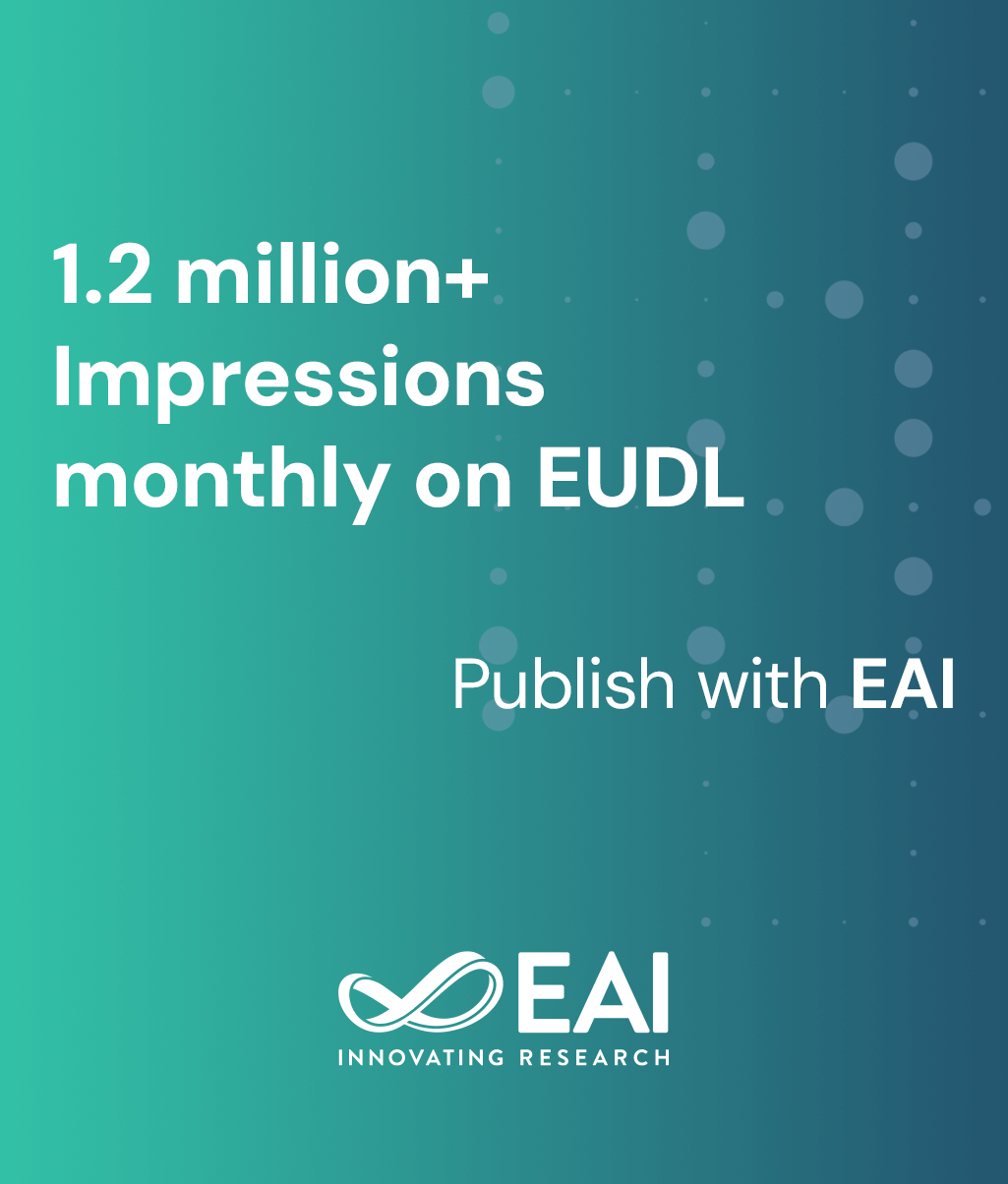
Research Article
Ensemble Based Medical Intelligent System for Discovering Cancerous Lung Nodules
@INPROCEEDINGS{10.4108/eai.23-11-2023.2343288, author={Chandrakala P}, title={Ensemble Based Medical Intelligent System for Discovering Cancerous Lung Nodules}, proceedings={Proceedings of the 1st International Conference on Artificial Intelligence, Communication, IoT, Data Engineering and Security, IACIDS 2023, 23-25 November 2023, Lavasa, Pune, India}, publisher={EAI}, proceedings_a={IACIDS}, year={2024}, month={3}, keywords={clustering classification ensemble}, doi={10.4108/eai.23-11-2023.2343288} }
- Chandrakala P
Year: 2024
Ensemble Based Medical Intelligent System for Discovering Cancerous Lung Nodules
IACIDS
EAI
DOI: 10.4108/eai.23-11-2023.2343288
Abstract
Lung cancer is the one of the risky diseases which has the highest mortality rate. Hence earliest detection of cancer can upturn the chance of human survival rate. This paper aims to discover the cancerous lung nodules in Computed Tomography (CT) images through Ensemble Based Medical Intelligent System (EBMIS). The proposed system firstly embeds image preprocessing mechanisms for enlightening the quality of CT images to improve the exactness of result. Then Nodule Detection System (NDS) is employed to segment the nodule candidates from lung region and set of intensity, geometric and texture features are extracted from the segmented nodules. Finally, distinguish the cancerous nodule from non-cancerous nodule is done by ensemble of classifiers encompassing Decision Trees, Naïve Bayes, K-NN and SVM Algorithms. The improvement in classification in terms of reduced error rate and improved accuracy is obtained by performing clustering ensemble prior to classification which includes K-Means, FCM, DBSCAN and Hierarchical Clustering algorithms. Open Lung Image dataset from Lung Image Database Consortium (LIDC) is experimented for quantifying the efficacy of proposed system. The proposed approach outperforms existing art of approaches by achieving stability even in increasing the dataset size. The Experimental outcome is confirmed that the proposed system is a promising tool in diagnosing cancerous lung nodules with accuracy of 99.63%.