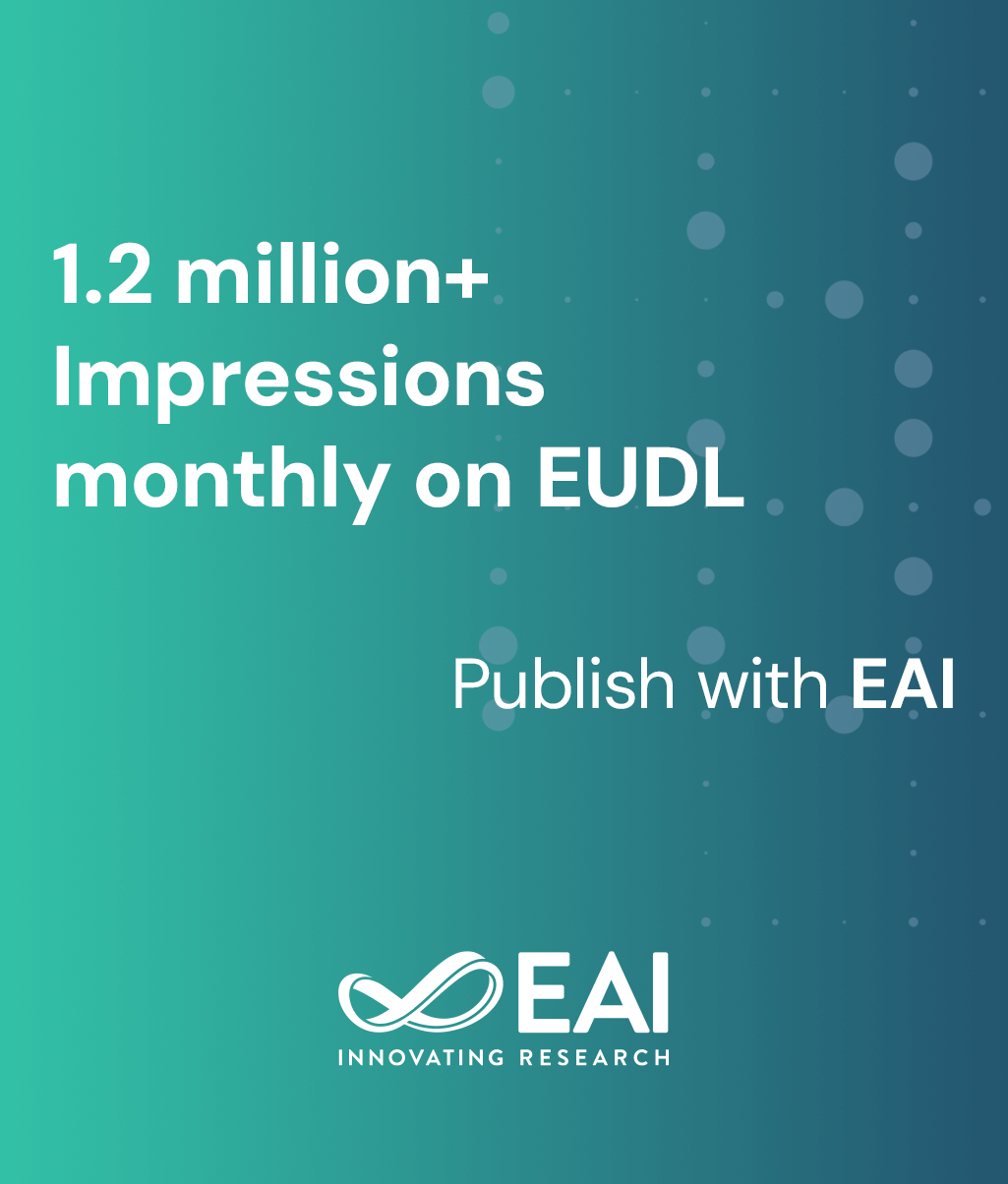
Research Article
A Framework for Performing an Analysis on Behavioral traits using Machine Learning
@INPROCEEDINGS{10.4108/eai.23-11-2023.2343255, author={Christy Jacqueline and Ranjith Singh K}, title={A Framework for Performing an Analysis on Behavioral traits using Machine Learning}, proceedings={Proceedings of the 1st International Conference on Artificial Intelligence, Communication, IoT, Data Engineering and Security, IACIDS 2023, 23-25 November 2023, Lavasa, Pune, India}, publisher={EAI}, proceedings_a={IACIDS}, year={2024}, month={3}, keywords={mental health behavioral traits k-means agglomerative hierarchical clustering classifiers}, doi={10.4108/eai.23-11-2023.2343255} }
- Christy Jacqueline
Ranjith Singh K
Year: 2024
A Framework for Performing an Analysis on Behavioral traits using Machine Learning
IACIDS
EAI
DOI: 10.4108/eai.23-11-2023.2343255
Abstract
Personality is a combination of an individual’s behavior, emotion, motivation and characteristics of their thought pattern. The main aim of this work is to find a better solution for identifying behavioral characteristics systematically using methods such as KMeans and Agglomerative Hierarchical clustering. In the first phase, clustering is used to identify the different personality traits. In the second phase different machine learning algorithms such as Naive Bayes, Logistic Regression, K Nearest Neighbor, Decision Tree and Random Forest are used. A real-time dataset is used for building the prediction models. To evaluate the effectiveness of the proposed framework, a step-by step model evaluation is done. The metrics such as accuracy, precision, recall, ROC AUC Score are used to evaluate the performance. The accuracy rate for the Random Forest and Decision tree was higher. Random Forest has slightly better .95 accuracy rate when compared to Decision Tree with 93.