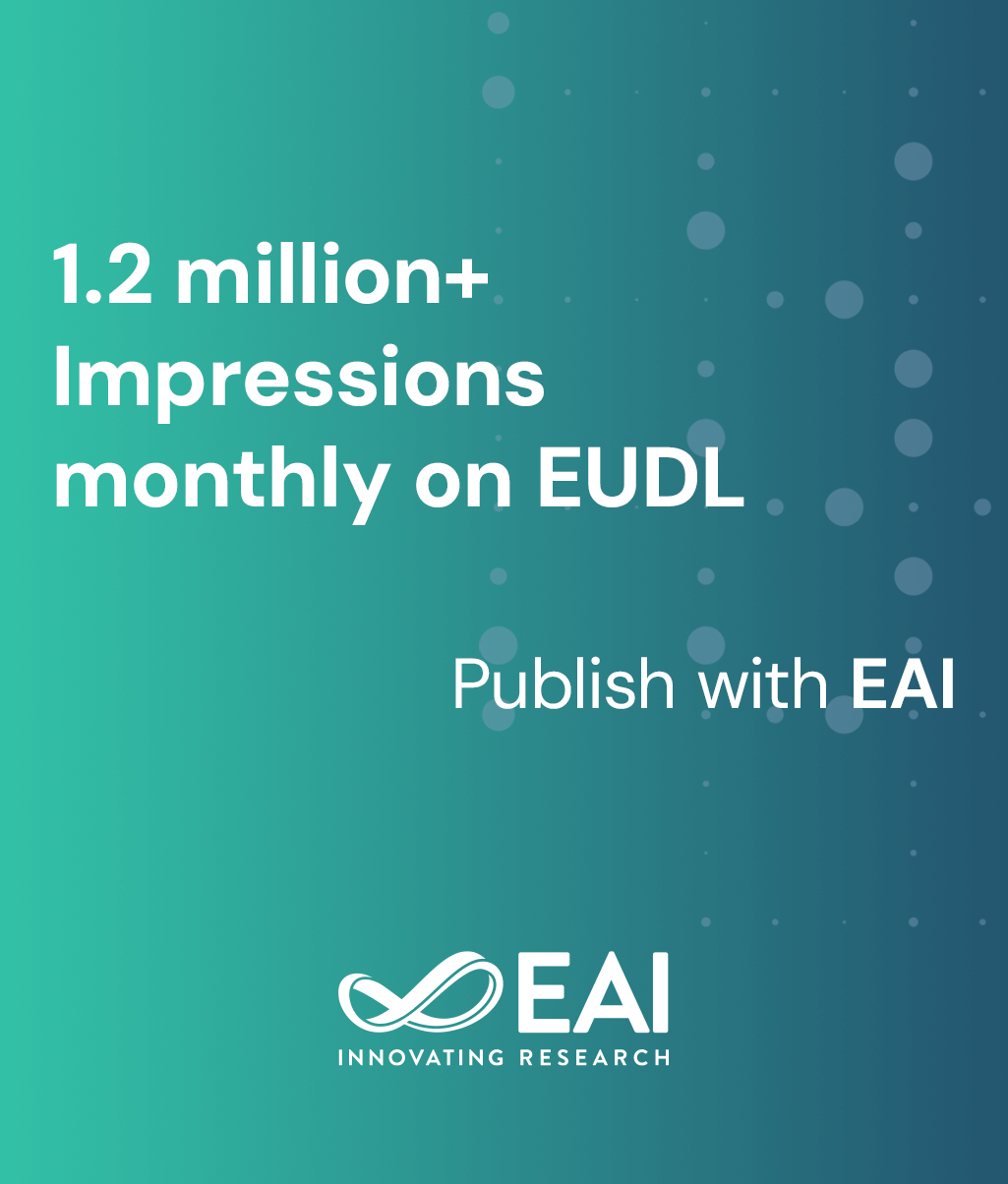
Research Article
Artificial Intelligence Based Anomaly Detection in Patient Health Monitoring Using Ensemble Learning Methods
@INPROCEEDINGS{10.4108/eai.23-11-2023.2343250, author={Ajitha P}, title={Artificial Intelligence Based Anomaly Detection in Patient Health Monitoring Using Ensemble Learning Methods}, proceedings={Proceedings of the 1st International Conference on Artificial Intelligence, Communication, IoT, Data Engineering and Security, IACIDS 2023, 23-25 November 2023, Lavasa, Pune, India}, publisher={EAI}, proceedings_a={IACIDS}, year={2024}, month={3}, keywords={artificial intelligence semi-supervised k-means health care isolation forest unsupervised anomaly detection}, doi={10.4108/eai.23-11-2023.2343250} }
- Ajitha P
Year: 2024
Artificial Intelligence Based Anomaly Detection in Patient Health Monitoring Using Ensemble Learning Methods
IACIDS
EAI
DOI: 10.4108/eai.23-11-2023.2343250
Abstract
A Novel anomaly detection algorithm called SemiAI-AnomalyDetect+ is proposed in this paper. It is specifically designed for large-scale patient datasets in the healthcare domain. Combining unsupervised K-means clustering and semi-supervised learning techniques, the algorithm achieves robust and adaptable anomaly detection. Its performance is evaluated on a diverse patient records dataset from the MIMIC-III critical care database, featuring various health-related attributes. Comparative analysis with anomaly detection algorithms, including Isolation Forest and One-Class SVM, revealed that SemiAI-AnomalyDetect+ outperforms the baselines in precision, recall, F1-score, and ROC-AUC. With an average precision of 0.86 and an ROC-AUC of 0.93, the proposed algorithm excels at identifying anomalies with greater accuracy and efficiency. The integration of a feedback loop and active learning mechanism allows it to continually improve, effective tool for anomaly detection in healthcare, addressing the dynamic challenges of patient data.