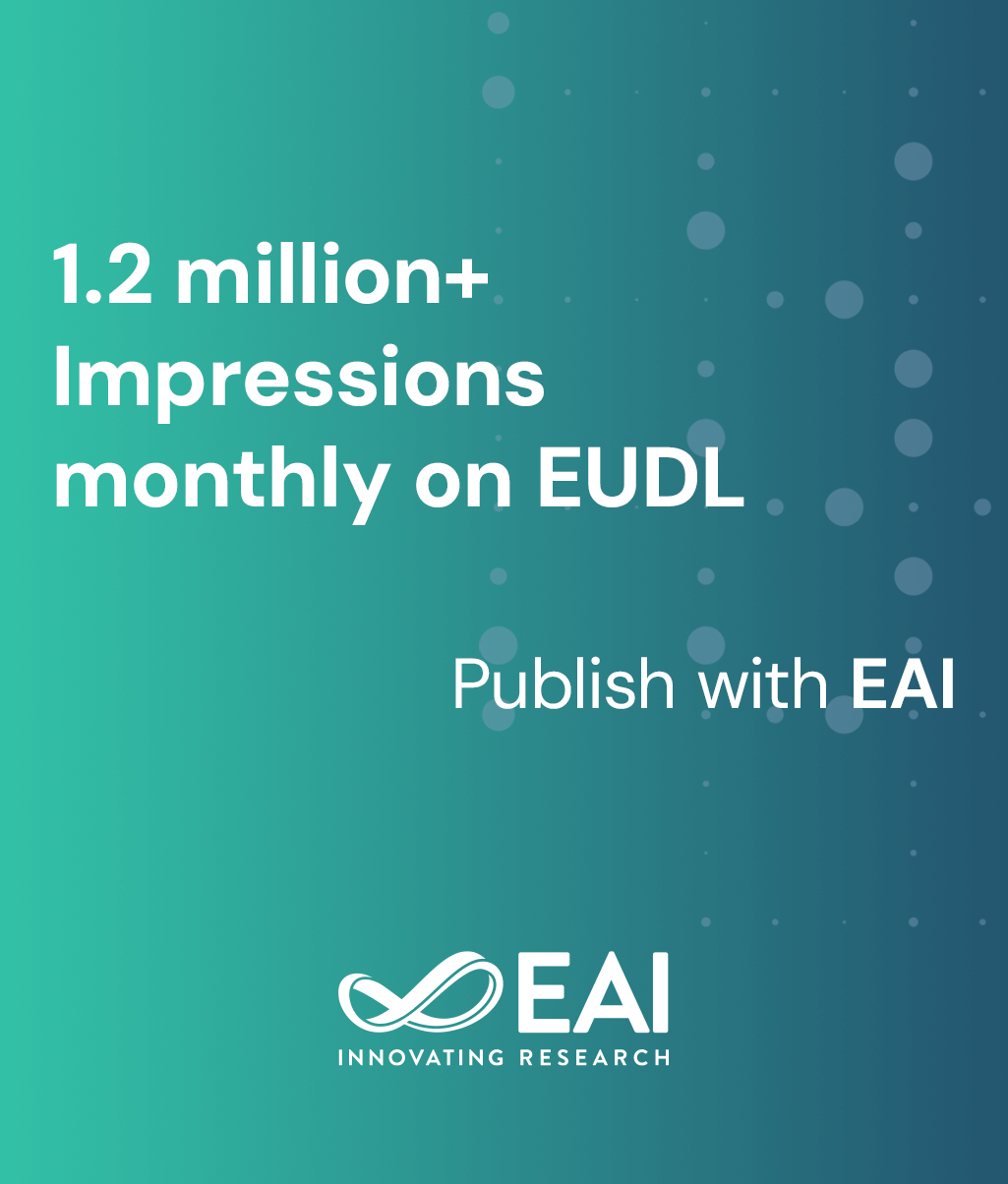
Research Article
End-to-End Implementation of Malware Detection Using Machine Learning
@INPROCEEDINGS{10.4108/eai.23-11-2023.2343241, author={Subbulakshmi T and Josiga Subramanian and Suganya R and Girish Subramanian and Yerramalli Sai Sreekar and Vasu Bansal}, title={End-to-End Implementation of Malware Detection Using Machine Learning}, proceedings={Proceedings of the 1st International Conference on Artificial Intelligence, Communication, IoT, Data Engineering and Security, IACIDS 2023, 23-25 November 2023, Lavasa, Pune, India}, publisher={EAI}, proceedings_a={IACIDS}, year={2024}, month={3}, keywords={machine learning malware detection cyber security malware feature extraction random forest principal component analysis adaboost gaussian na\~{n}ve bayes pe file}, doi={10.4108/eai.23-11-2023.2343241} }
- Subbulakshmi T
Josiga Subramanian
Suganya R
Girish Subramanian
Yerramalli Sai Sreekar
Vasu Bansal
Year: 2024
End-to-End Implementation of Malware Detection Using Machine Learning
IACIDS
EAI
DOI: 10.4108/eai.23-11-2023.2343241
Abstract
The trend in rising frequency of malware attacks provides the need for effective malware detection techniques. Machine learning has emerged as a promising approach for identifying and classifying malicious software, where the detection of new attacks are performed with the single or combination of machine learning algorithms. In this research work, a web application that utilizes machine learning techniques to classify the uploaded files as malware or not has been implemented. The system is based on a comprehensive dataset containing known malware and benign files, which are used for training and testing of the machine learning algorithms. Experimental results demonstrate that the implemented system is highly effective at detecting malware, achieving high accuracy rates even with previously unseen samples. The importance of machine learning for addressing the growing threat of malware attacks and provides a practical solution for identifying potentially harmful files has been highlighted.