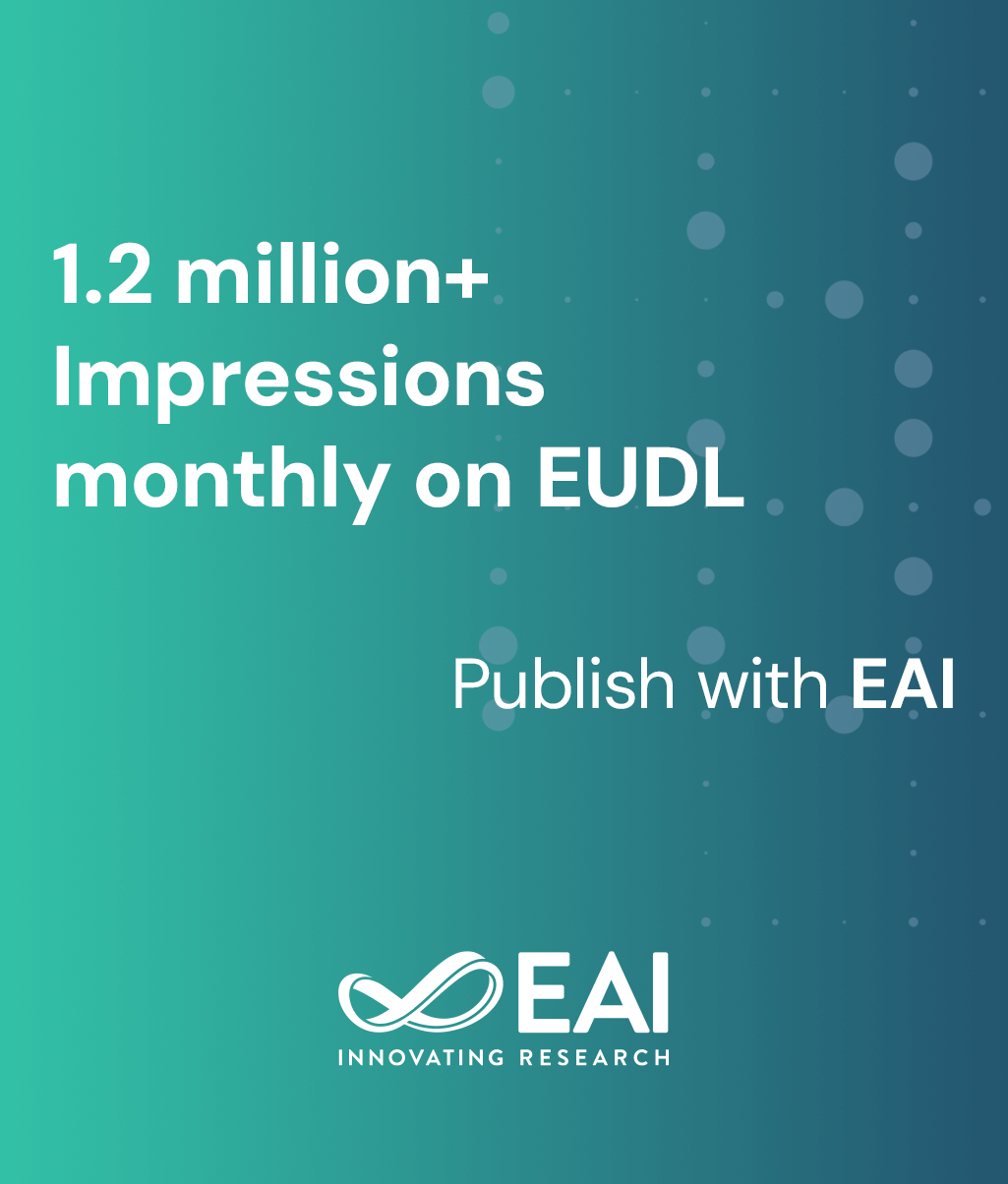
Research Article
Pattern Recognition in Medical Decision Support and Estimating Redundancy in Clinical Text
@INPROCEEDINGS{10.4108/eai.23-11-2023.2343233, author={Kalluri Shanmukha Sai and Rishi Reddy Thokala}, title={Pattern Recognition in Medical Decision Support and Estimating Redundancy in Clinical Text}, proceedings={Proceedings of the 1st International Conference on Artificial Intelligence, Communication, IoT, Data Engineering and Security, IACIDS 2023, 23-25 November 2023, Lavasa, Pune, India}, publisher={EAI}, proceedings_a={IACIDS}, year={2024}, month={3}, keywords={feature selection redundancy information medical health care data support vector machines}, doi={10.4108/eai.23-11-2023.2343233} }
- Kalluri Shanmukha Sai
Rishi Reddy Thokala
Year: 2024
Pattern Recognition in Medical Decision Support and Estimating Redundancy in Clinical Text
IACIDS
EAI
DOI: 10.4108/eai.23-11-2023.2343233
Abstract
Feature sets selected for multidimensional pattern classification are estimated using a novel criterion. The priority of features for each class is generally optimized to maximize relevancy and minimize redundancy between each class. While mutual information can be used to estimate relevancy information, redundancy information cannot be estimated since its dynamic ambit is determined by feature and class. In addition to assisting in adjust of changing patterns, support vector machines are helpful in classifying normal and abnormal patterns. In pattern matching, Random Forest Logic's pattern recognition algorithm lends itself easily to pattern matching algorithms. An algorithm for evaluating the classification performance of health care medical data is proposed. A variety of experimental results confirm that the proffered technique is more veracious than conventional algorithms relating to the classification of accuracy when the number of selected features is varied.