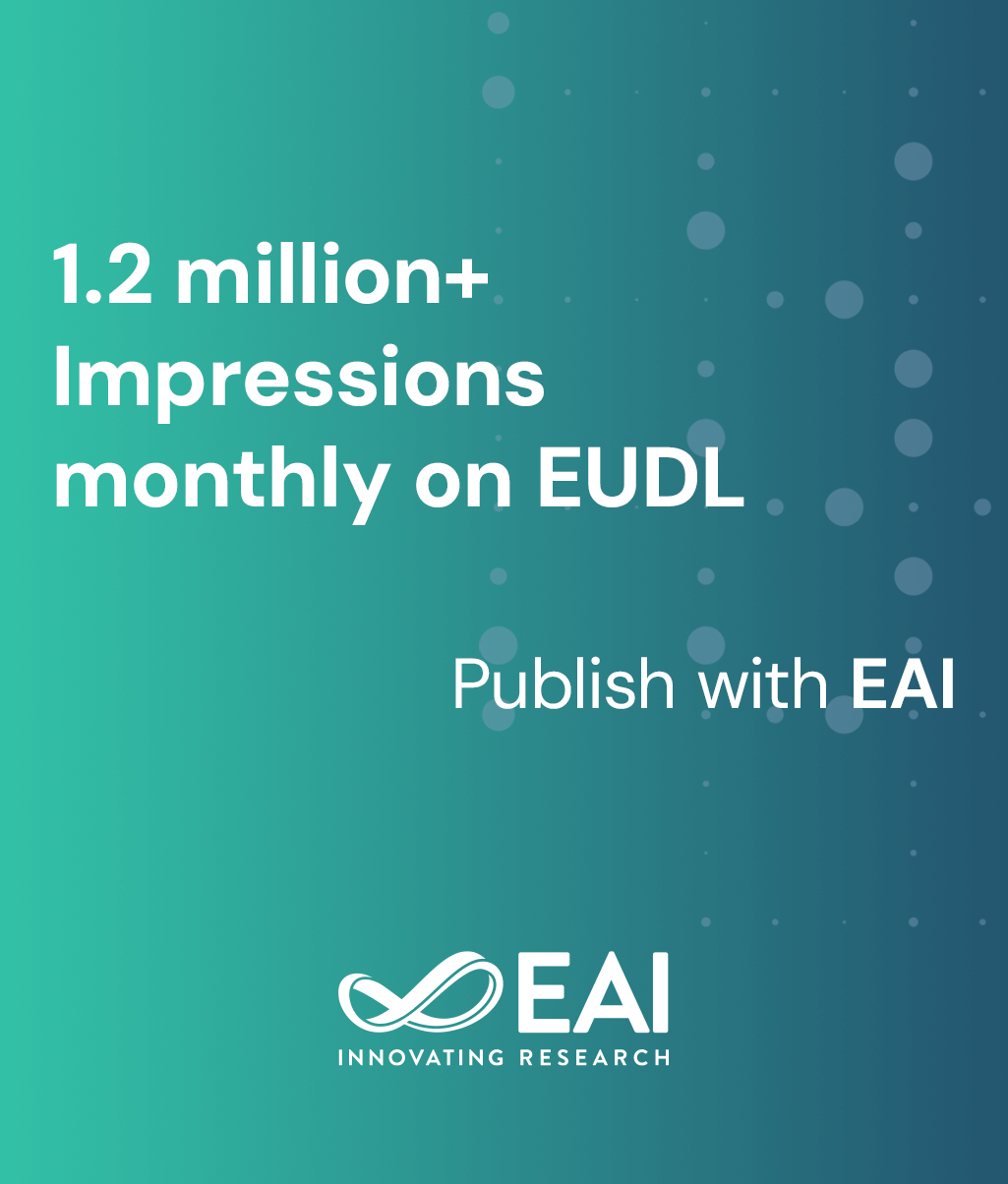
Research Article
An Inclusive Concurrent Approach To Diagnosing Oryza Sativa Leaf Disease Using Machine Learning Techniques
@INPROCEEDINGS{10.4108/eai.23-11-2023.2343230, author={Mary Shanthi Rani M and Arockia Jackuline Joni J}, title={An Inclusive Concurrent Approach To Diagnosing Oryza Sativa Leaf Disease Using Machine Learning Techniques}, proceedings={Proceedings of the 1st International Conference on Artificial Intelligence, Communication, IoT, Data Engineering and Security, IACIDS 2023, 23-25 November 2023, Lavasa, Pune, India}, publisher={EAI}, proceedings_a={IACIDS}, year={2024}, month={3}, keywords={rice leaf disease detection machine learning deep learning artificial intelligence dataset supervised learning}, doi={10.4108/eai.23-11-2023.2343230} }
- Mary Shanthi Rani M
Arockia Jackuline Joni J
Year: 2024
An Inclusive Concurrent Approach To Diagnosing Oryza Sativa Leaf Disease Using Machine Learning Techniques
IACIDS
EAI
DOI: 10.4108/eai.23-11-2023.2343230
Abstract
Rice diseases, impacting half the world's food supply, threaten yields by 37% annually. Machine learning (ML) and deep learning (DL) offer promising solutions for early detection. These powerful tools have revolutionized computer vision, enabling automated and accurate disease identification through image analysis. While existing algorithms like Logistic Regression and KNN show potential, research is limited. This study delves into rice diseases, explores ML/DL applications, and evaluates their effectiveness. It highlights recent advances and their performance, paving the way for effective disease control and safeguarding rice production.
Copyright © 2023–2025 EAI