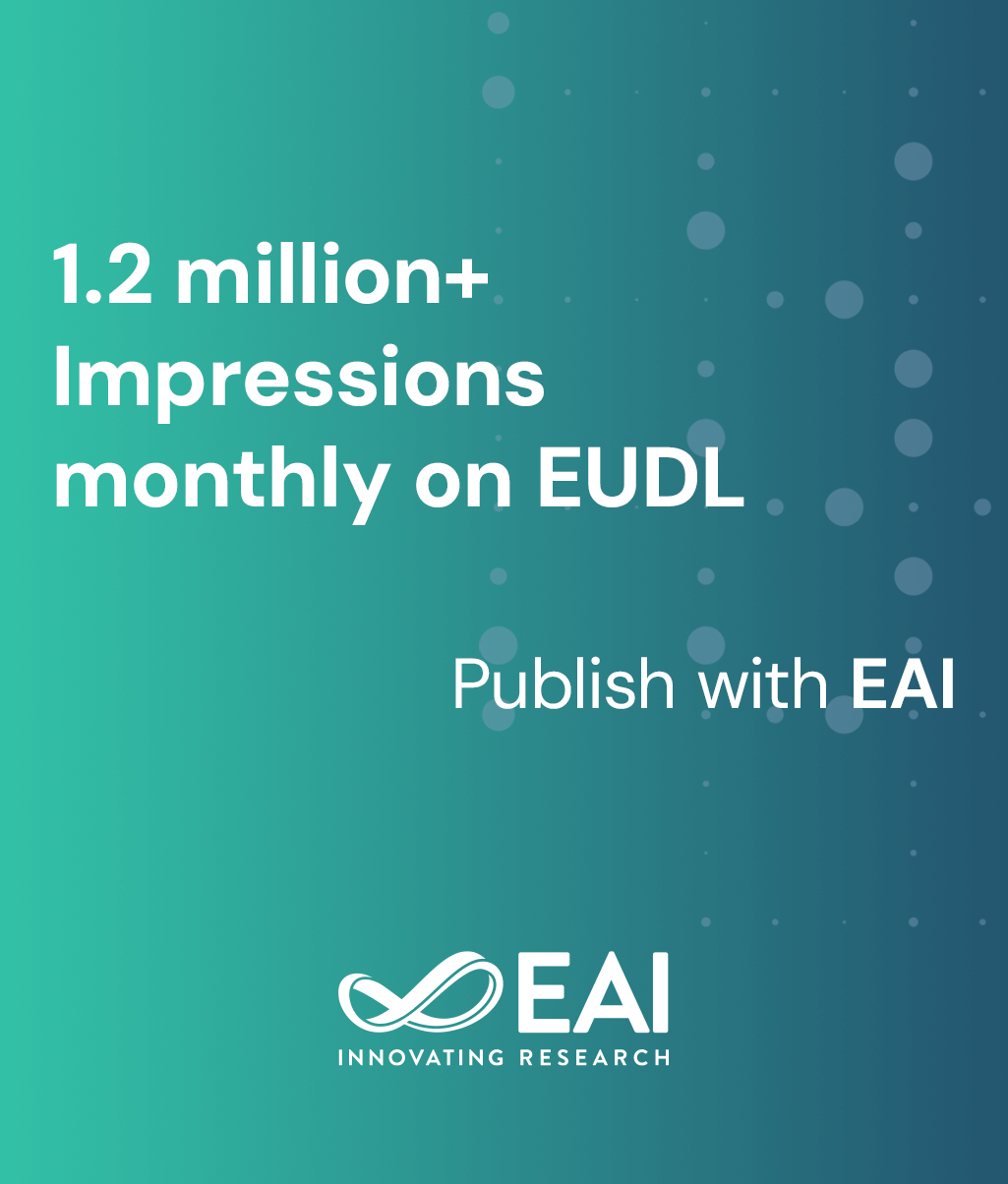
Research Article
3D Grad-CAM in Lung Cancer Images using Deep Learning Techniques
@INPROCEEDINGS{10.4108/eai.23-11-2023.2343226, author={Bhavani P and Chithra PL}, title={3D Grad-CAM in Lung Cancer Images using Deep Learning Techniques }, proceedings={Proceedings of the 1st International Conference on Artificial Intelligence, Communication, IoT, Data Engineering and Security, IACIDS 2023, 23-25 November 2023, Lavasa, Pune, India}, publisher={EAI}, proceedings_a={IACIDS}, year={2024}, month={3}, keywords={lung computed tomography (ct) 3d grad -- cam 3d convolutional neural networks 3d heatmap and overlay deep learning}, doi={10.4108/eai.23-11-2023.2343226} }
- Bhavani P
Chithra PL
Year: 2024
3D Grad-CAM in Lung Cancer Images using Deep Learning Techniques
IACIDS
EAI
DOI: 10.4108/eai.23-11-2023.2343226
Abstract
Medical image processing approach play a vital role in 3D Convolutional Neural Networks (CNN) using Grad-CAM (Gradient-Weighted Class Activation Mapping) techniques. This proposed 3D Grad-CAM architecture identifies the part of the tumor in the input image and utilizes the Gradient Class Activation map to classify the feature map and highlight the tumor to predict the tumor regions in the convolution last layer. This 3D network is a very difficult task to segment the regions in two-dimensional(2D) images into three dimensional(3D) images Digital Imaging and Communications in Medicine (2D DICOM).The 2D lung cancer DICOM dataset images are to A Large-Scale CT and PET/CT Dataset for Lung Cancer Diagnosis (Lung-PET-CT-Dx) taken from The Cancer Imaging Archive (TCIA) Portal. The proposed 3D DICOM Shape Conversion techniques are used to convert the 2D image dimension to 3D image dimension with the facilitate the slice and size of the lung image by applying normalization steps refine and enhance the lung images saved in 3D volume. After creating a 3D volume to perform training and testing with the proposed architecture of 3D GradCAM – CNN generates the Grad-CAM Map by combining heat map and overlay and predicting the highlight tumor region. Our experimental results achieved accuracy of 0.85, Precision of 0.90, recall of 0.82, and Fl–Score of 0.86 outperformed the results of highlighted tumor regions than pre-trained EfficientNet and ResNet (A Residual Neural Network) architectures.