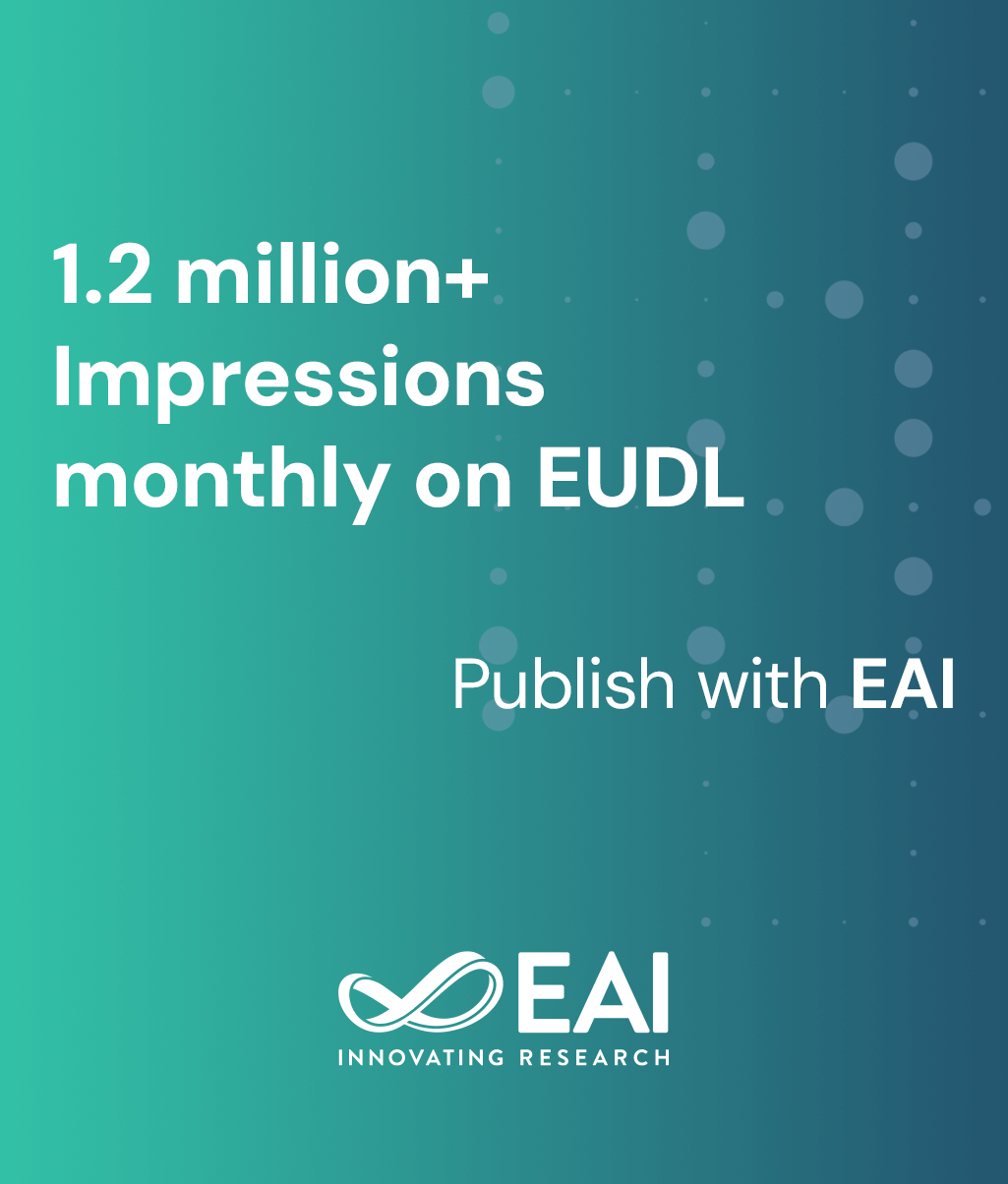
Research Article
MRI-Based Brain Tumor detection and classification using Artificial Neural Network
@INPROCEEDINGS{10.4108/eai.23-11-2023.2343221, author={Chithra PL and Yegammai T}, title={MRI-Based Brain Tumor detection and classification using Artificial Neural Network}, proceedings={Proceedings of the 1st International Conference on Artificial Intelligence, Communication, IoT, Data Engineering and Security, IACIDS 2023, 23-25 November 2023, Lavasa, Pune, India}, publisher={EAI}, proceedings_a={IACIDS}, year={2024}, month={3}, keywords={brain tumor classification segmentation artificial neural network tumor detection}, doi={10.4108/eai.23-11-2023.2343221} }
- Chithra PL
Yegammai T
Year: 2024
MRI-Based Brain Tumor detection and classification using Artificial Neural Network
IACIDS
EAI
DOI: 10.4108/eai.23-11-2023.2343221
Abstract
The process of brain tumor categorization and identification using MRI (magnetic resonance imaging) is one of the challenging domains in medical field. There were numerous malignancies such as glioma tumor, no tumor (benign), pituitary tumor and meningioma tumor.In this paper, an efficient automated methodhas been proposedto identify and classify tumor image from the MRI images.This proposed methodology includes three processing steps, including pre-processing, segmentation and feature classification from MRI images. In this,the Otsu thresholding technique is first applied to separate tumor from input brain image. Then then combination of three methods, namelyDWT(Discrete wavelet transform), PCA (Principal Component Analysis) and GLCM (Gray level co-occurrence matrix) to extract image attributes from the fragmented MRI data. Further, the extracted feature images are applied to the classifiers namely Naive Bayes (NB), Support Vector Machine (SVM), K-Nearest Neighbors (KNN) and Decision Tree (DT). Analysing the results of above machine learning classifiers, the Artificial Neural Network (ANN) model obtains a 97.6% accuracy rate and the minimum loss rate of 0.028817. It is evident from the experimental result, the proposed method has a great chance of detecting tumor efficiently.