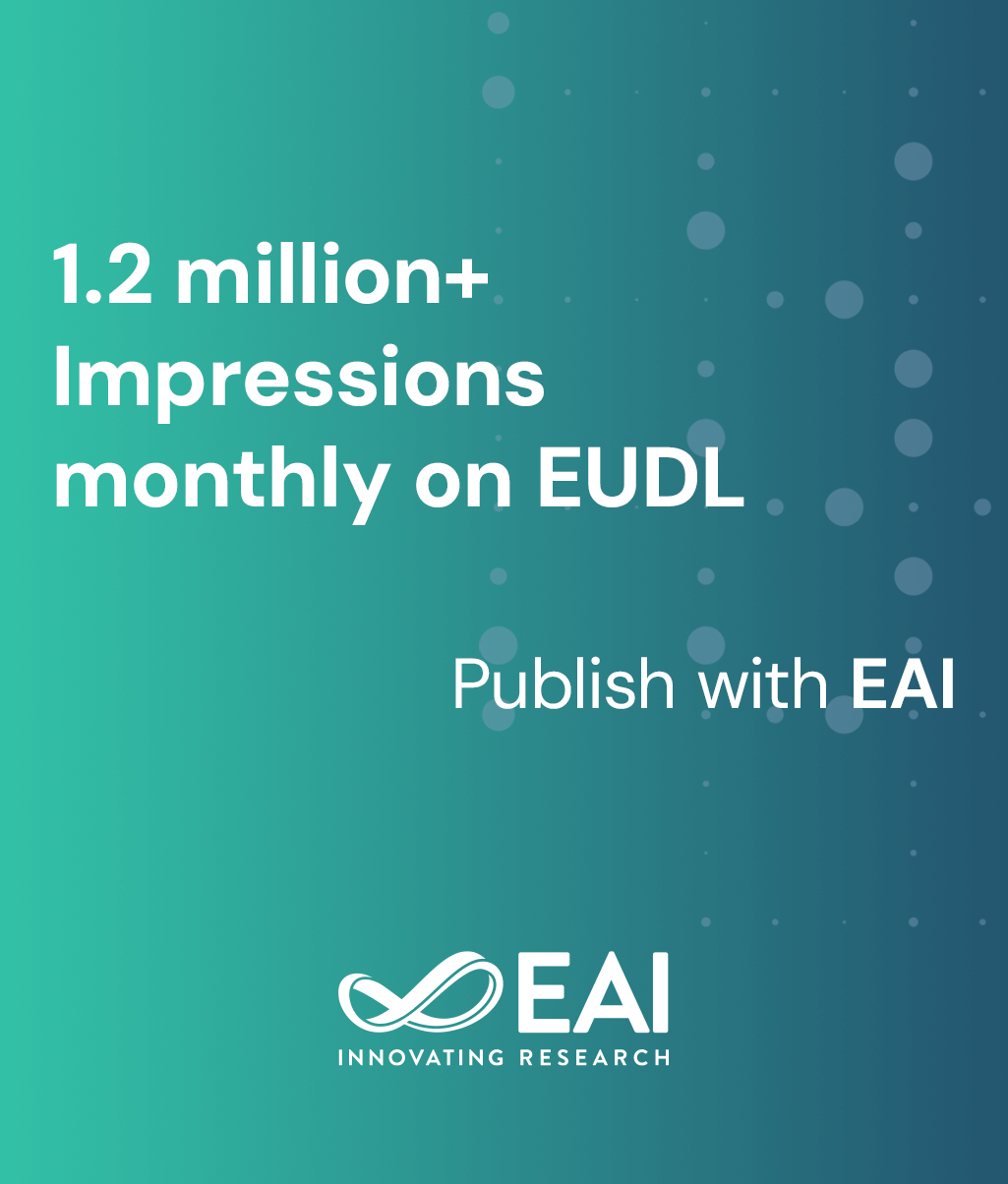
Research Article
The Classification Model for Plant Disease Detection with Segmentation and GLCM
@INPROCEEDINGS{10.4108/eai.23-11-2023.2343186, author={Shalini Sharma and Rajesh E and Baskar Kasi and Sakthi Govindaraju}, title={The Classification Model for Plant Disease Detection with Segmentation and GLCM}, proceedings={Proceedings of the 1st International Conference on Artificial Intelligence, Communication, IoT, Data Engineering and Security, IACIDS 2023, 23-25 November 2023, Lavasa, Pune, India}, publisher={EAI}, proceedings_a={IACIDS}, year={2024}, month={3}, keywords={plant disease glcm mlp decision tree random forest voting}, doi={10.4108/eai.23-11-2023.2343186} }
- Shalini Sharma
Rajesh E
Baskar Kasi
Sakthi Govindaraju
Year: 2024
The Classification Model for Plant Disease Detection with Segmentation and GLCM
IACIDS
EAI
DOI: 10.4108/eai.23-11-2023.2343186
Abstract
Diseases in plants may be detected automatically as the first symptoms show on leaves. In this research, we provide a method based on algorithms for identifying plant diseases. The four steps that make up this method are as follows: picture pre-processing, image segmentation, feature extraction, and disease classification. The GLCM algorithm is used to assess the severity of illness based on the presence or absence of symptoms on diseased leaves, and a voting classifier is used to rank the symptoms. By integrating the Random Forest and Decision Tree methods with Multi-Layer Perceptron, the voting classifier increases the reliability of early illness identification. The agriculture sector is crucial to the nation's gross domestic product. If farmers want to get the most of their crops, they need to spot illnesses early. Automation raises the bar for speed and accuracy in early disease diagnosis. Automatic detection of illness occurs after symptoms show on the leaves. In this study, an automated system was developed to help with diagnosis of plant illnesses. Its foundation is a four-step process: image preprocessing, image segmentation, feature extraction, and disease classification. The GLCM technique is used to assess a leaf's symptoms, and a vote classifier is offered to assist identify the condition. This classifier uses Random Forest, Decision Tree, and MLP methods to improve its ability to detect diseases at an early stage.