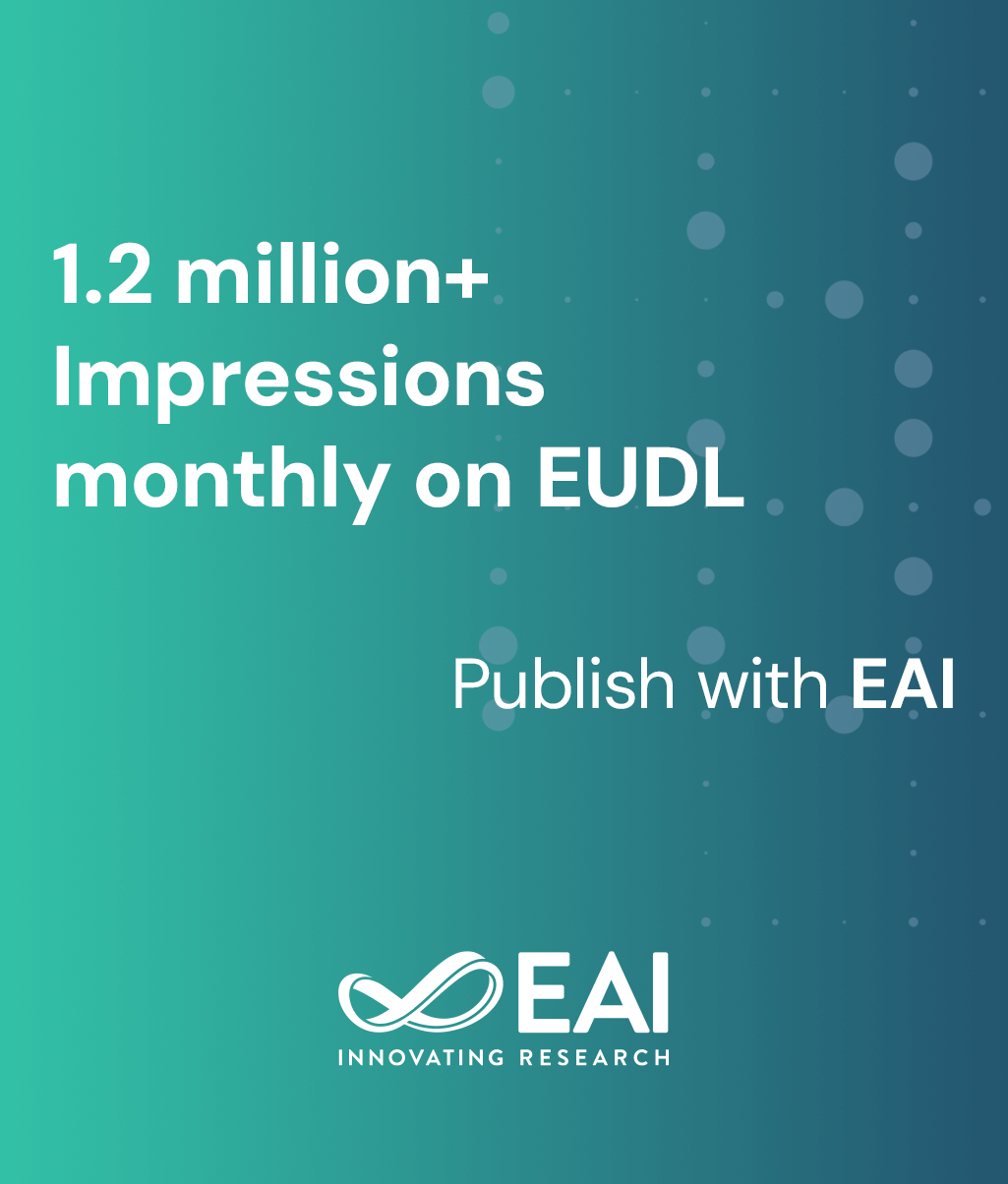
Research Article
BertSentiment-SO Model: Extracting Cloud Service Information from Stack Overflow’s Technical Texts
@INPROCEEDINGS{10.4108/eai.23-11-2023.2343182, author={Aishwarya Sundaram and Leow Jun Shou and Hema Subramaniam}, title={BertSentiment-SO Model: Extracting Cloud Service Information from Stack Overflow’s Technical Texts}, proceedings={Proceedings of the 1st International Conference on Artificial Intelligence, Communication, IoT, Data Engineering and Security, IACIDS 2023, 23-25 November 2023, Lavasa, Pune, India}, publisher={EAI}, proceedings_a={IACIDS}, year={2024}, month={3}, keywords={technical text sentiment analysis cloud service providers stack overflow}, doi={10.4108/eai.23-11-2023.2343182} }
- Aishwarya Sundaram
Leow Jun Shou
Hema Subramaniam
Year: 2024
BertSentiment-SO Model: Extracting Cloud Service Information from Stack Overflow’s Technical Texts
IACIDS
EAI
DOI: 10.4108/eai.23-11-2023.2343182
Abstract
The recent surge in sentiment analysis within software engineering texts has gained prominence due to its proven influence on productivity and collaborative work quality. Stack Overflow, a substantial repository of software engineering content, has become a focal point for sentiment analysis research, particularly in software development. Nevertheless, integrating sentiment analysis into software engineering has unveiled potential shortcomings in their efficacy. Researchers have had to adapt these tools to navigate limitations, as exemplified by the cases of SentiCR and Senti4SD. Moreover, natural language processing has made significant strides in recent times, primarily attributed to the emergence of pre-trained transformer-based models. Addressing this research gap, the study introduces a dedicated sentiment analysis model, BertSentiment-SO. This study delves explicitly into sentiment analysis for the three foremost cloud service providers: Amazon, Google, and Microsoft. Extracted from the Big Query public dataset, the data undergoes preprocessing and labelling via a semi-supervised learning approach. Harnessing the capabilities of BERT, our proposed model outperforms its counterparts, enabling a more precise score of 94.06% for the evaluation of sentiment within the domain of cloud services.