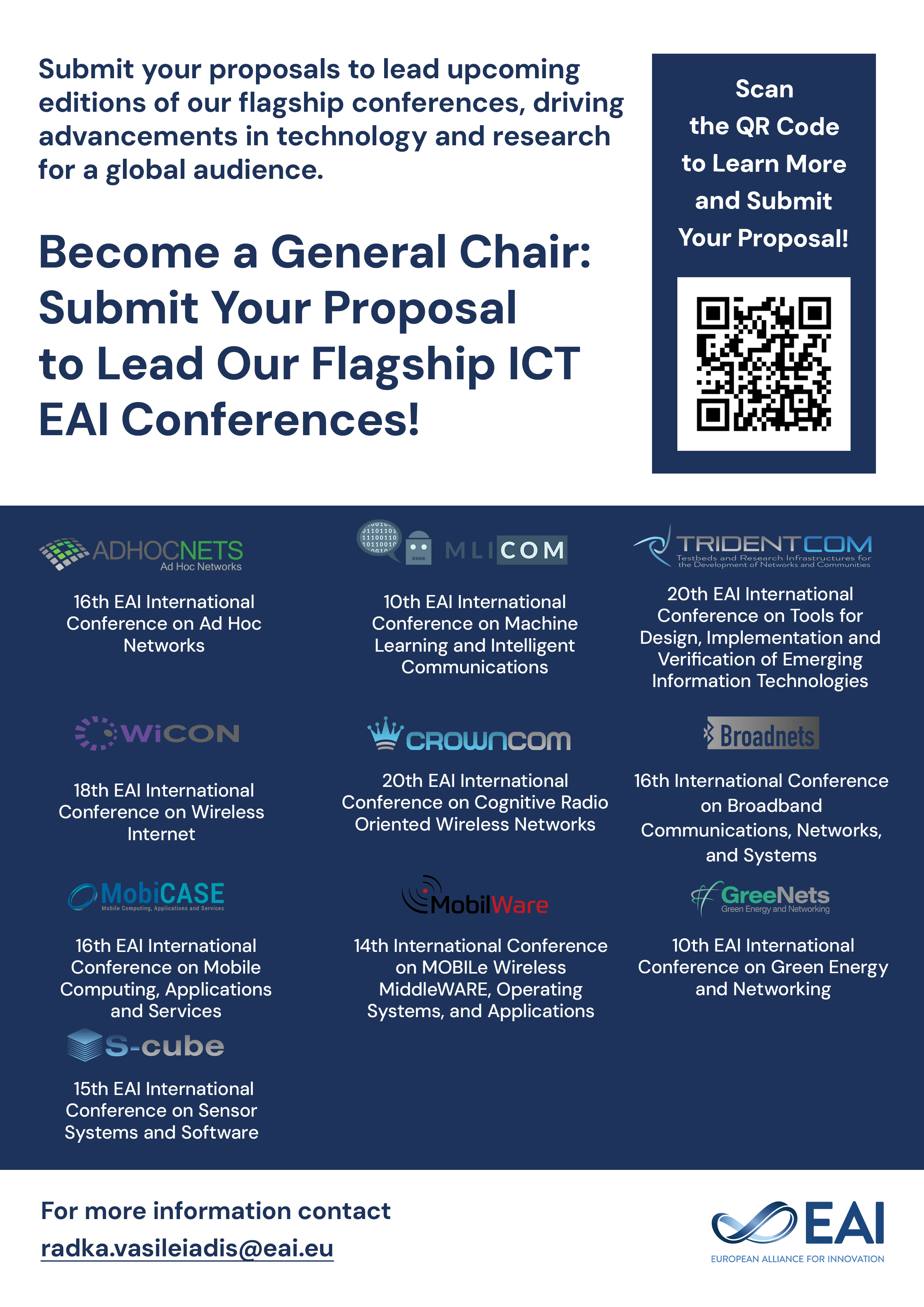
Research Article
Comparing Machine Learning Techniques for Loan Approval Prediction
@INPROCEEDINGS{10.4108/eai.23-11-2023.2343174, author={Krishnaraj P and Rita S and Jitendra Jaiswal}, title={ Comparing Machine Learning Techniques for Loan Approval Prediction}, proceedings={Proceedings of the 1st International Conference on Artificial Intelligence, Communication, IoT, Data Engineering and Security, IACIDS 2023, 23-25 November 2023, Lavasa, Pune, India}, publisher={EAI}, proceedings_a={IACIDS}, year={2024}, month={3}, keywords={loan approval automated loan machine learning logistic regression}, doi={10.4108/eai.23-11-2023.2343174} }
- Krishnaraj P
Rita S
Jitendra Jaiswal
Year: 2024
Comparing Machine Learning Techniques for Loan Approval Prediction
IACIDS
EAI
DOI: 10.4108/eai.23-11-2023.2343174
Abstract
Automated loan eligibility prediction plays a pivotal role in transforming the lending landscape by harnessing the power of data analytics and machine learning. It revolutionizes the loan approval process, making it more efficient and accessible. The use of advanced algorithms ensures greater accuracy and fairness in evaluating creditworthiness, mitigating the risk of human biases. It empowers lenders to assess a wider range of applicants, including those with limited credit history. Cost savings, improved fraud detection, and personalized loan offerings are additional benefits, making automated loan eligibility prediction an indispensable tool for lending institutions. In the last decade, machine learning techniques have obtained significant attention in automating and enhancing loan approval processes. This research paper focuses to provide a comparative analysis of different machine learning techniques applied in multiple areas such as loan approval, exploring their strengths, limitations, and performance. We applied three popular machine learning models, namely Logistic Regression, Decision Tree and its extension as Random Forest and to predict and classify the target variable. The primary objective was to perform a comparative analysis of these models and identify the most suitable one for the task at hand. After thorough analysis and comparison, we found that the Logistic Regression model demonstrated a slightly superior performance in comparison to the other models.