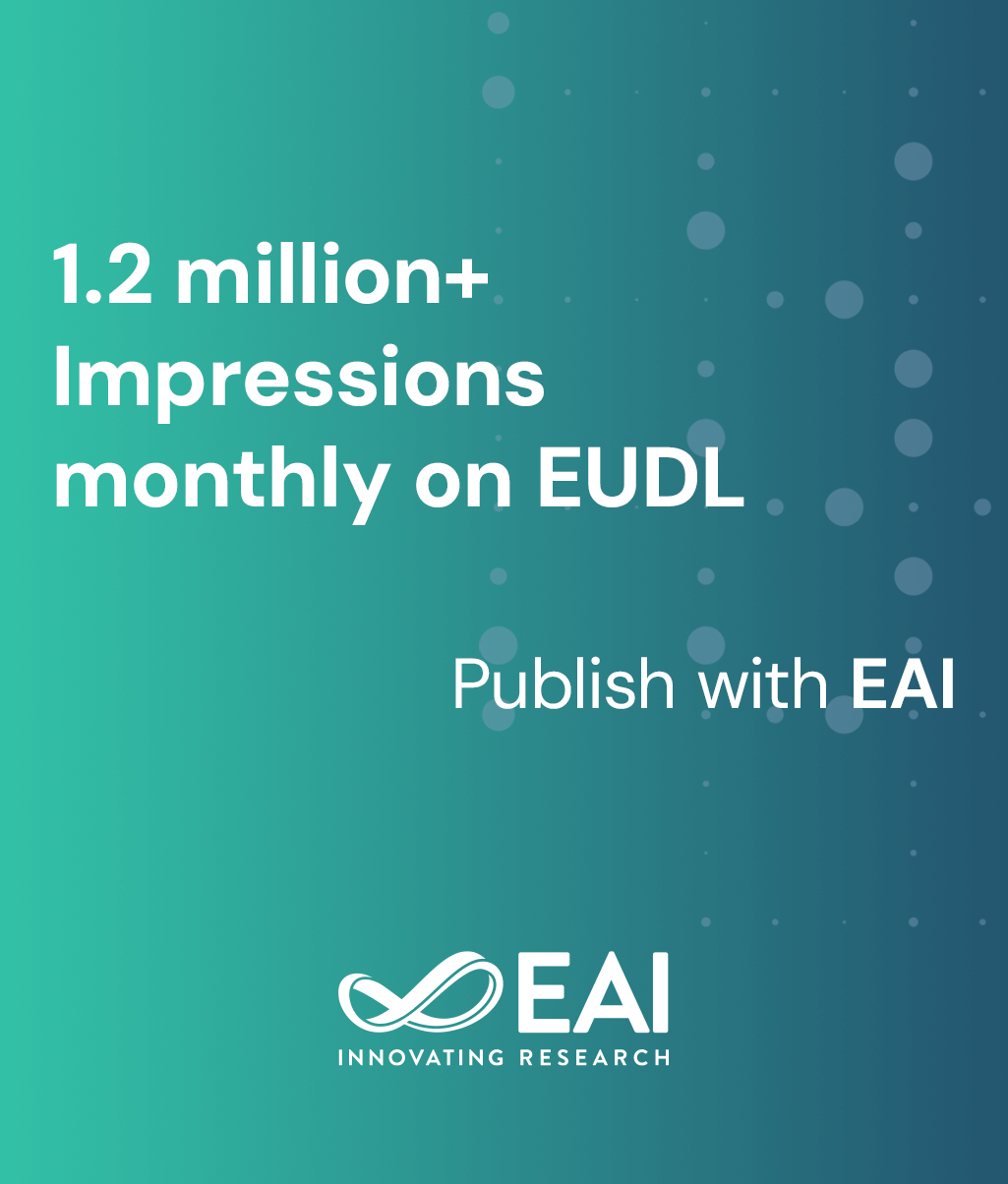
Research Article
Enhancing Pre-Trained Transfer Learning Performance Using Automated Brain Tumor Classification
@INPROCEEDINGS{10.4108/eai.23-11-2023.2343173, author={Sivakumar Karuppan and Kalpana G and Sika K and Abhirami J S}, title={Enhancing Pre-Trained Transfer Learning Performance Using Automated Brain Tumor Classification}, proceedings={Proceedings of the 1st International Conference on Artificial Intelligence, Communication, IoT, Data Engineering and Security, IACIDS 2023, 23-25 November 2023, Lavasa, Pune, India}, publisher={EAI}, proceedings_a={IACIDS}, year={2024}, month={3}, keywords={deep learning a detection model mri images disease prediction and disease classification}, doi={10.4108/eai.23-11-2023.2343173} }
- Sivakumar Karuppan
Kalpana G
Sika K
Abhirami J S
Year: 2024
Enhancing Pre-Trained Transfer Learning Performance Using Automated Brain Tumor Classification
IACIDS
EAI
DOI: 10.4108/eai.23-11-2023.2343173
Abstract
Classification and prediction of brain tumors are essential for the early diagnosis and treatment of brain-related conditions. Broad exploration and strategic enhancements over the long haul bear witness to the need of cerebrum cancer recognition and investigation for any sign framework. Exact cancer recognition is fundamental for this review, thus a productive robotized technique should be executed. To aid in and enhance the classification of brain tumors, numerous segmentation algorithms have been developed. Brain imaging segmentation is a well-known and challenging subfield of medical image processing. A novel automated detection and classification system is suggested by the study. Preprocessing MRI images, segmenting those images, extracting features, and classifying those features are the stages that make up the suggested strategy. In this review, we propose an exchange learning way to deal with precisely characterize mind growths and foresee their threat utilizing progressed profound learning methods. After the features from the MRI scans were extracted, a deep learning model was used to divide the images into gliomas, meningiomas, non-tumors, and pituitary tumors. For grouping, VGG-16 Net pre-prepared models were utilized. To upgrade the VGG-16 Net model's presentation, information expansion strategies are applied to lighten overfitting and further develop speculation.