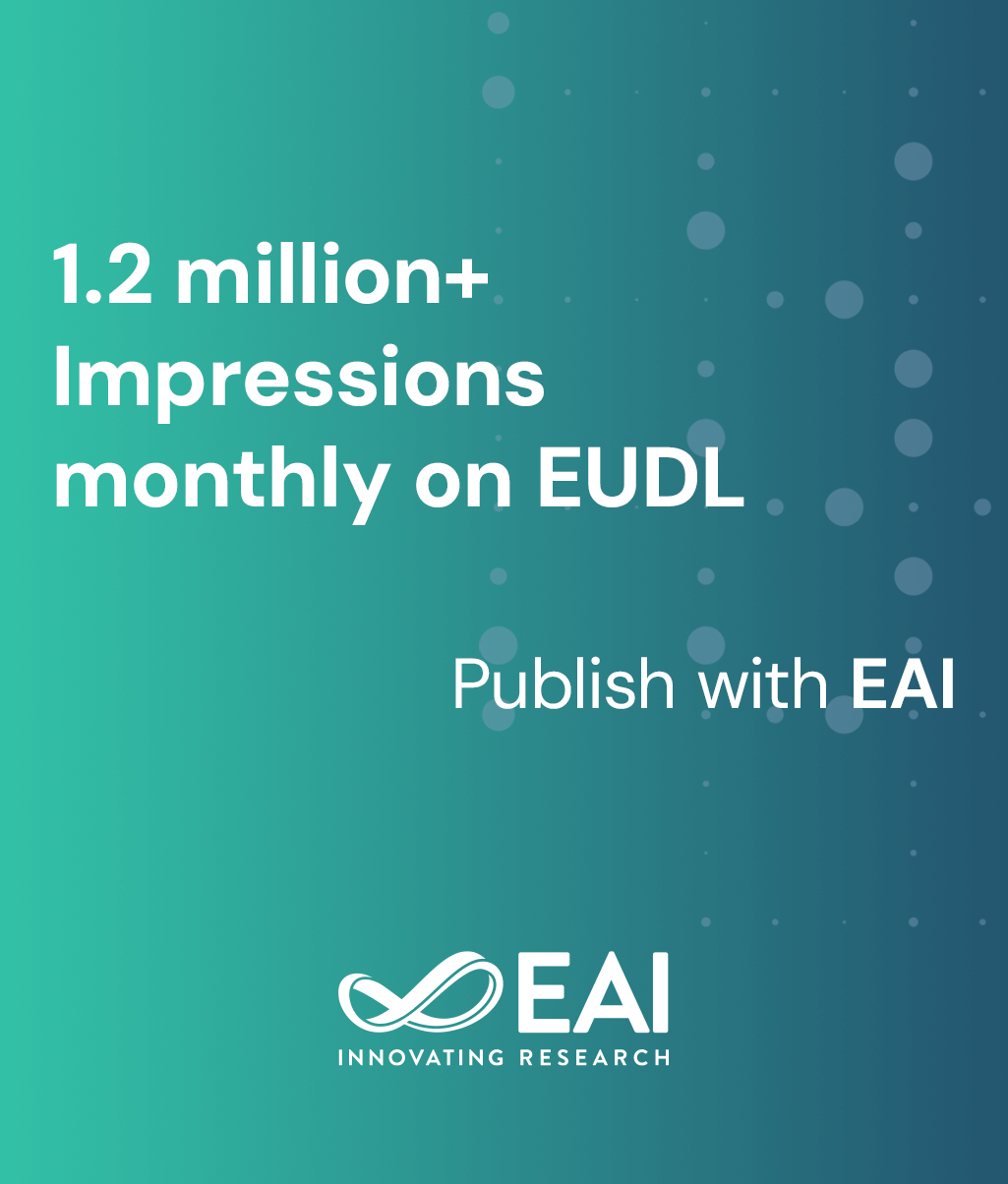
Research Article
An Effective Learning Ensemble based Prediction Model for Detecting COVID-19 from Human Chest X-ray Images
@INPROCEEDINGS{10.4108/eai.23-11-2023.2343172, author={Deepankumar S and MaruthaVeni R}, title={An Effective Learning Ensemble based Prediction Model for Detecting COVID-19 from Human Chest X-ray Images}, proceedings={Proceedings of the 1st International Conference on Artificial Intelligence, Communication, IoT, Data Engineering and Security, IACIDS 2023, 23-25 November 2023, Lavasa, Pune, India}, publisher={EAI}, proceedings_a={IACIDS}, year={2024}, month={3}, keywords={ensemble machine learning novel coronavirus disease 2019 (covid -- 19) intelligent prediction image and computer vision}, doi={10.4108/eai.23-11-2023.2343172} }
- Deepankumar S
MaruthaVeni R
Year: 2024
An Effective Learning Ensemble based Prediction Model for Detecting COVID-19 from Human Chest X-ray Images
IACIDS
EAI
DOI: 10.4108/eai.23-11-2023.2343172
Abstract
The emergence of the Coronavirus Disease-19 (COVID-19) has presented a formidable global challenge. Manual diagnosis has been hindered by limited access to radiologists and experts, as well as their varying proficiency in interpreting the intricate features within chest X-ray (CXR) images associated with the disease. In response to this, this study introduces an innovative automated screening ensemble model designed to differentiate between normal patients, those infected with COVID-19, and individuals with suspected COVID-19, all through the utilization of radiomic texture descriptors extracted from CXR images. The ensemble based prediciton model leverages a selection based classifier that combines and ensembles the strength of two well-established supervised classification algorithms. Remarkably, this ensemble model achieves a substantial enhancement in prediction accuracy, boasting an impressive 94% accuracy rate. Furthermore, it excels in the precise identification of COVID-19 cases, achieving an accuracy and precision of 93%. This advancement in automated screening not only streamlines the diagnostic process but also provides a reliable tool in the ongoing battle against the global COVID-19 pandemic.