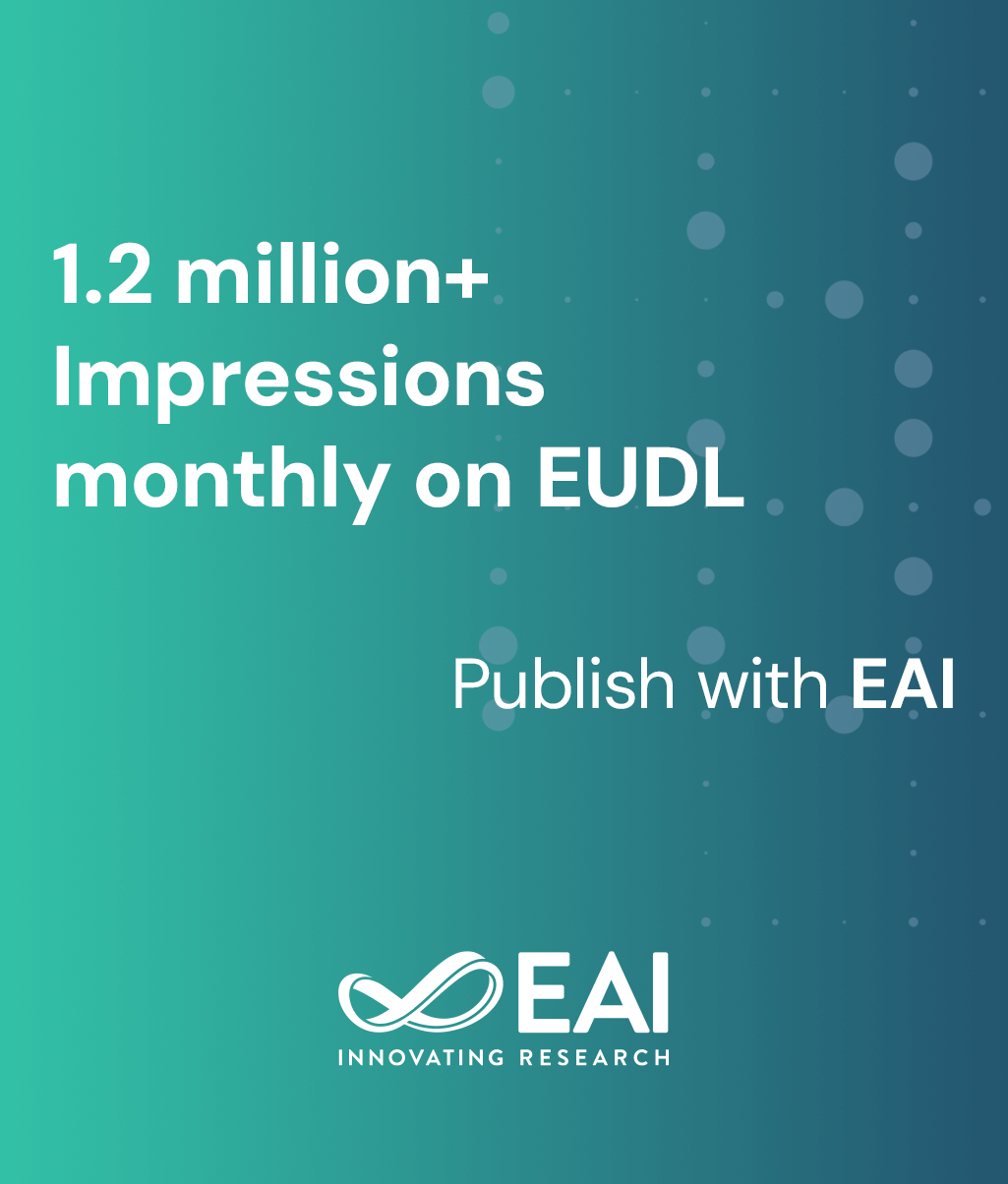
Research Article
Fake site detection using Machine Learning Algorithm and N-Gram Analysis
@INPROCEEDINGS{10.4108/eai.23-11-2023.2343171, author={Asha J and Saradha S and Mohanraj A and Devipriya C}, title={ Fake site detection using Machine Learning Algorithm and N-Gram Analysis}, proceedings={Proceedings of the 1st International Conference on Artificial Intelligence, Communication, IoT, Data Engineering and Security, IACIDS 2023, 23-25 November 2023, Lavasa, Pune, India}, publisher={EAI}, proceedings_a={IACIDS}, year={2024}, month={3}, keywords={fake sites feature extraction n-gram analysis svm knn lr}, doi={10.4108/eai.23-11-2023.2343171} }
- Asha J
Saradha S
Mohanraj A
Devipriya C
Year: 2024
Fake site detection using Machine Learning Algorithm and N-Gram Analysis
IACIDS
EAI
DOI: 10.4108/eai.23-11-2023.2343171
Abstract
The prevalence of fake websites increases as more people uses the internet. The identification of fake internet sites has thus been the subject of increased research in recent years.Website detection is extremely difficult because there aren't enough resources, or datasets available. This study seeks to identify fake websites by integrating two independent feature extraction techniques with Support vector machines, KNN, and logistic regression as learning algorithms. Term frequency (TF) and Term frequency-invented document frequency (TF-IDF) using N-gram analysis is also deteremined. To support the research, performance metrics are analyzed including accuracy, precision, and recall. The experiment results in a high TF feature extraction accuracy when N = 1, and a high TF-IDF feature extraction accuracy when employing the KNN algorithm (97.3%).