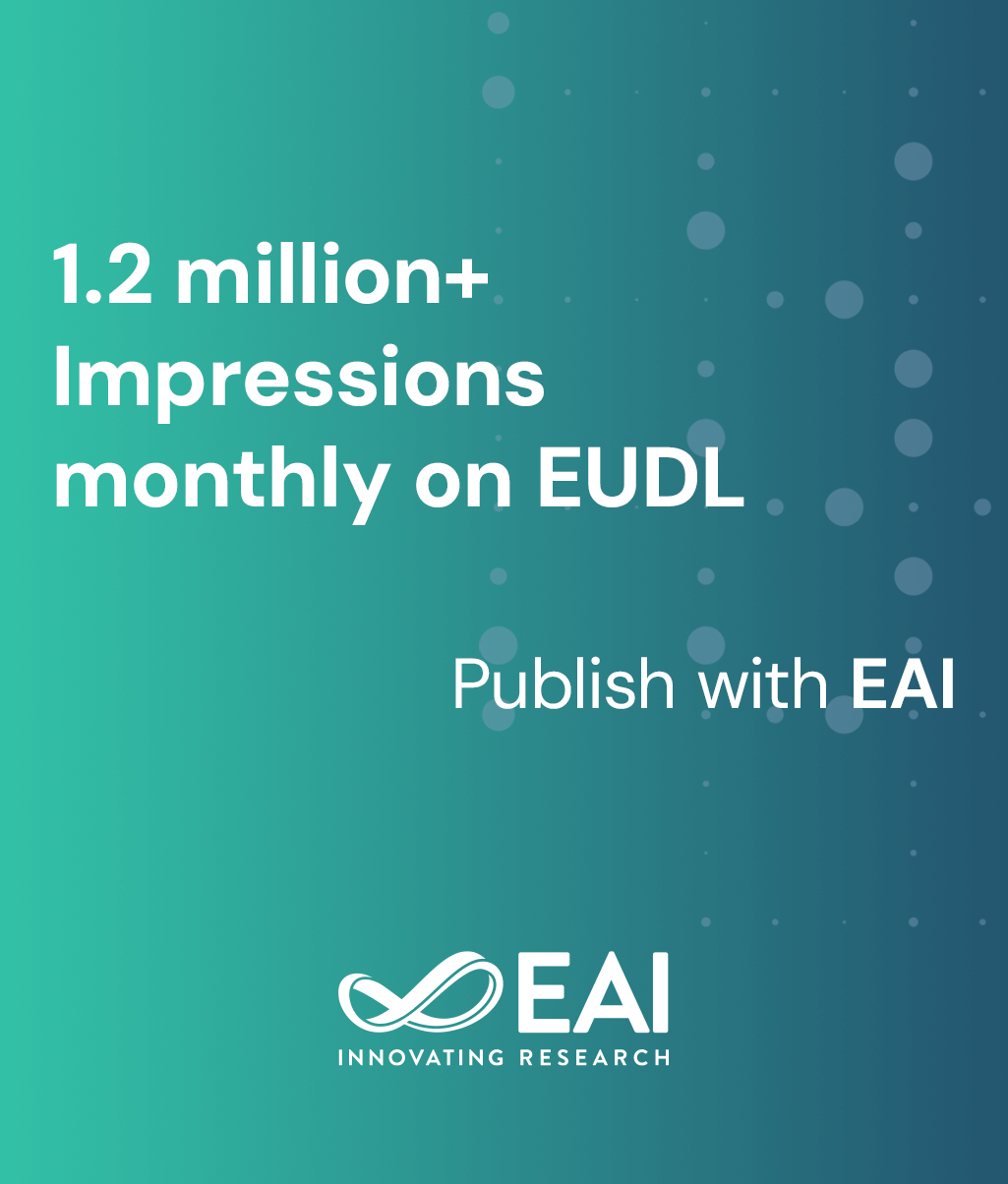
Research Article
An Effective Lung Cancer Diagnosis Model Using the CNN Algorithm
@INPROCEEDINGS{10.4108/eai.23-11-2023.2343145, author={Sonia Kukreja and Munish Sabharwal and Gill D S}, title={An Effective Lung Cancer Diagnosis Model Using the CNN Algorithm}, proceedings={Proceedings of the 1st International Conference on Artificial Intelligence, Communication, IoT, Data Engineering and Security, IACIDS 2023, 23-25 November 2023, Lavasa, Pune, India}, publisher={EAI}, proceedings_a={IACIDS}, year={2024}, month={3}, keywords={random forest image classification deep learning ct scan cnn}, doi={10.4108/eai.23-11-2023.2343145} }
- Sonia Kukreja
Munish Sabharwal
Gill D S
Year: 2024
An Effective Lung Cancer Diagnosis Model Using the CNN Algorithm
IACIDS
EAI
DOI: 10.4108/eai.23-11-2023.2343145
Abstract
Lung cancer is a very complicated disease and can be deadly if not detected on time or at the early phase. A cost effective and more accurate methodology is required to diagnose the lung cancer at an early stage. It results in improved diagnosis, saving the money as well as time and in simplifying the operations. This study focused on the 3Dimensional CNN architecture by categorizing the imaging data or histopathological images in three kinds of cancer: squamous cell carcinoma, adenocarcinoma, and Benign. The current research categorize these 3 types of cancer by implementing the CNN architecture and achieving the better accuracy by comparing with the other methologies used for the diagnosis of lung cancer. While implementing CNN method, accuracy achieved in training stage was 96.11 and accuracy achieved in validation was 97.2%. This proposed method has the potential to improve the detection of lung cancer by grouping them according to the symptoms they have. In this research, along with CNN, random forest technique has been used to to reduce the resources, labour required and time also. CNN model achieved the improved detection accuracy for lung cancer and saving the lives by indulging early disease recognition.