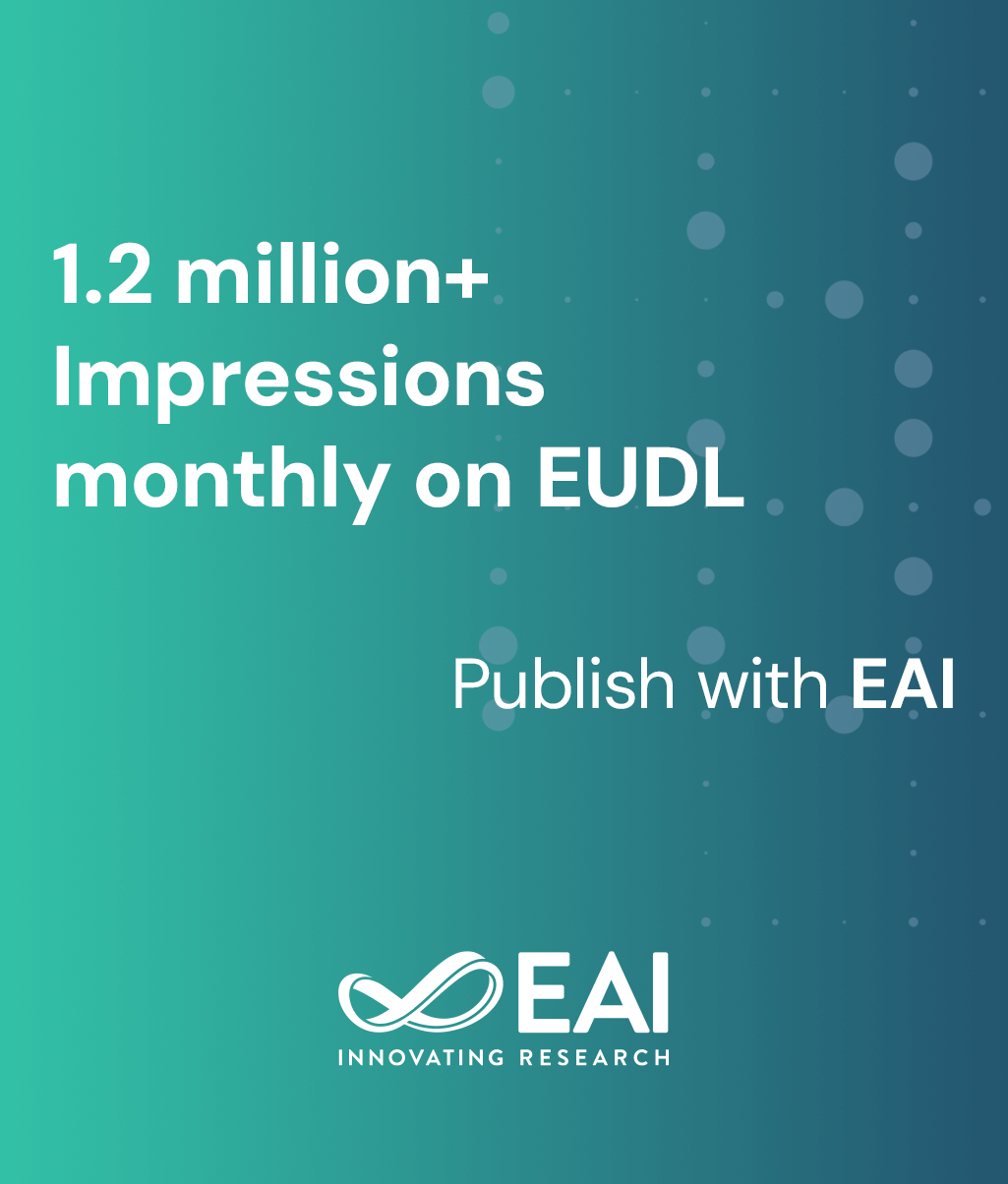
Research Article
Infrastructure-less Occupancy Detection and Semantic Localization in Smart Environments
@ARTICLE{10.4108/eai.22-7-2015.2260062, author={Md Abdullah Al Hafiz Khan and H M Sajjad Hossain and Nirmalya Roy}, title={Infrastructure-less Occupancy Detection and Semantic Localization in Smart Environments}, journal={EAI Endorsed Transactions on Context-aware Systems and Applications}, volume={2}, number={5}, publisher={ACM}, journal_a={CASA}, year={2015}, month={8}, keywords={crowdsourcing, opportunistic sensing, occupancy counting, semantic localization}, doi={10.4108/eai.22-7-2015.2260062} }
- Md Abdullah Al Hafiz Khan
H M Sajjad Hossain
Nirmalya Roy
Year: 2015
Infrastructure-less Occupancy Detection and Semantic Localization in Smart Environments
CASA
EAI
DOI: 10.4108/eai.22-7-2015.2260062
Abstract
Accurate estimation of localized occupancy related informa- tion in real time enables a broad range of intelligent smart environment applications. A large number of studies using heterogeneous sensor arrays reflect the myriad requirements of various emerging pervasive, ubiquitous and participatory sensing applications. In this paper, we introduce a zero- configuration and infrastructure-less smartphone based lo- cation specific occupancy estimation model. We opportunis- tically exploit smartphone’s acoustic sensors in a conversing environment and motion sensors in absence of any conver- sational data. We demonstrate a novel speaker estimation algorithm based on unsupervised clustering of overlapped and non-overlapped conversational data and a change point detection algorithm for locomotive motion of the users to infer the occupancy. We augment our occupancy detection model with a fingerprinting based methodology using smart- phone’s magnetometer sensor to accurately assimilate loca- tion information of any gathering. We postulate a novel crowdsourcing-based approach to annotate the semantic lo- cation of the occupancy. We evaluate our algorithms in dif- ferent contexts; conversational, silence and mixed in pres- ence of 10 domestic users. Our experimental results on real-life data traces in natural settings show that using this hybrid approach, we can achieve approximately 0.76 error count distance for occupancy detection accuracy on aver- age.
Copyright © 2015 M. A. A. H. Khan et al., licensed to EAI. This is an open access article distributed under the terms of the Creative Commons Attribution licence (http://creativecommons.org/licenses/by/3.0/), which permits unlimited use, distribution and reproduction in any medium so long as the original work is properly cited.