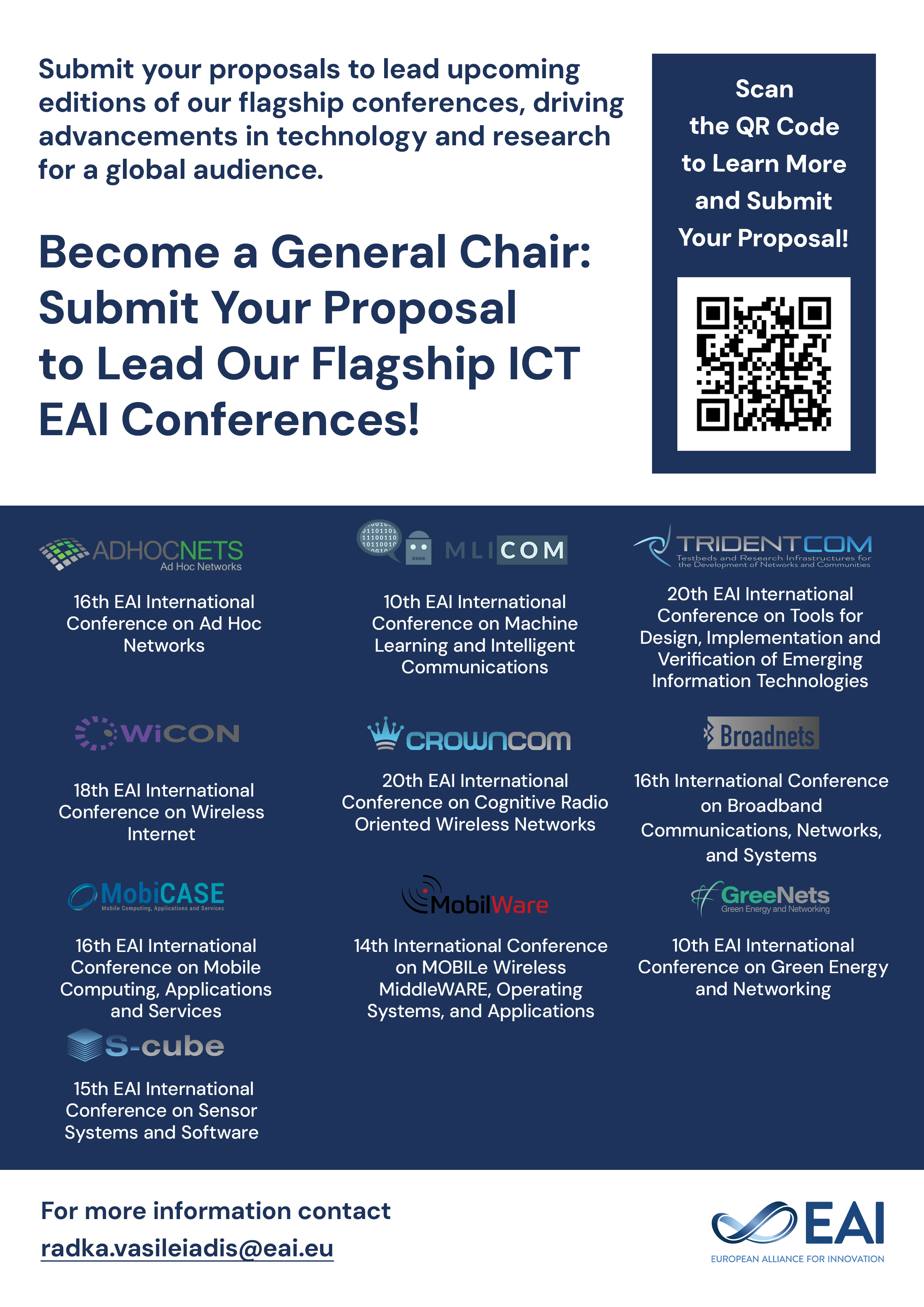
Research Article
Predicting Rice Production In Sumatra Island Using Linear Regression
@INPROCEEDINGS{10.4108/eai.21-9-2023.2342997, author={Uun Patrio and Yuliska Yuliska and Yohana Dewi Lulu Widyasari}, title={Predicting Rice Production In Sumatra Island Using Linear Regression}, proceedings={Proceedings of the 11th International Applied Business and Engineering Conference, ABEC 2023, September 21st, 2023, Bengkalis, Riau, Indonesia}, publisher={EAI}, proceedings_a={ABEC}, year={2024}, month={2}, keywords={prediction rice production sumatra island linear regression machine learning}, doi={10.4108/eai.21-9-2023.2342997} }
- Uun Patrio
Yuliska Yuliska
Yohana Dewi Lulu Widyasari
Year: 2024
Predicting Rice Production In Sumatra Island Using Linear Regression
ABEC
EAI
DOI: 10.4108/eai.21-9-2023.2342997
Abstract
This research explores the application of machine learning techniques to predict rice production in Sumatra Island using Linear Regression and compares it with five other algorithms: Random Forest Regression, Gradient Boosting, SVR, K-Nearest Neighbors Regression and Decision Tree Regression. After comparing the various models, the linear regression technique has the greatest R2-score (85.53%), demonstrating that it adequately explains the variation in the data. In comparison to some of the other algorithms examined, it has a Mean Absolute Error (MAE) value of 221938.68 and a Mean Squared Error (MSE) value of 176940698374.90, showing that Linear Regression tends to produce more precise predictions that are nearer to the true value. Therefore, this algorithm is considered the best choice for predicting agricultural production in Sumatra, in accordance with the research objectives. These findings highlight the potential of machine learning in improving rice production prediction models for agricultural planning and decision-making in the region.