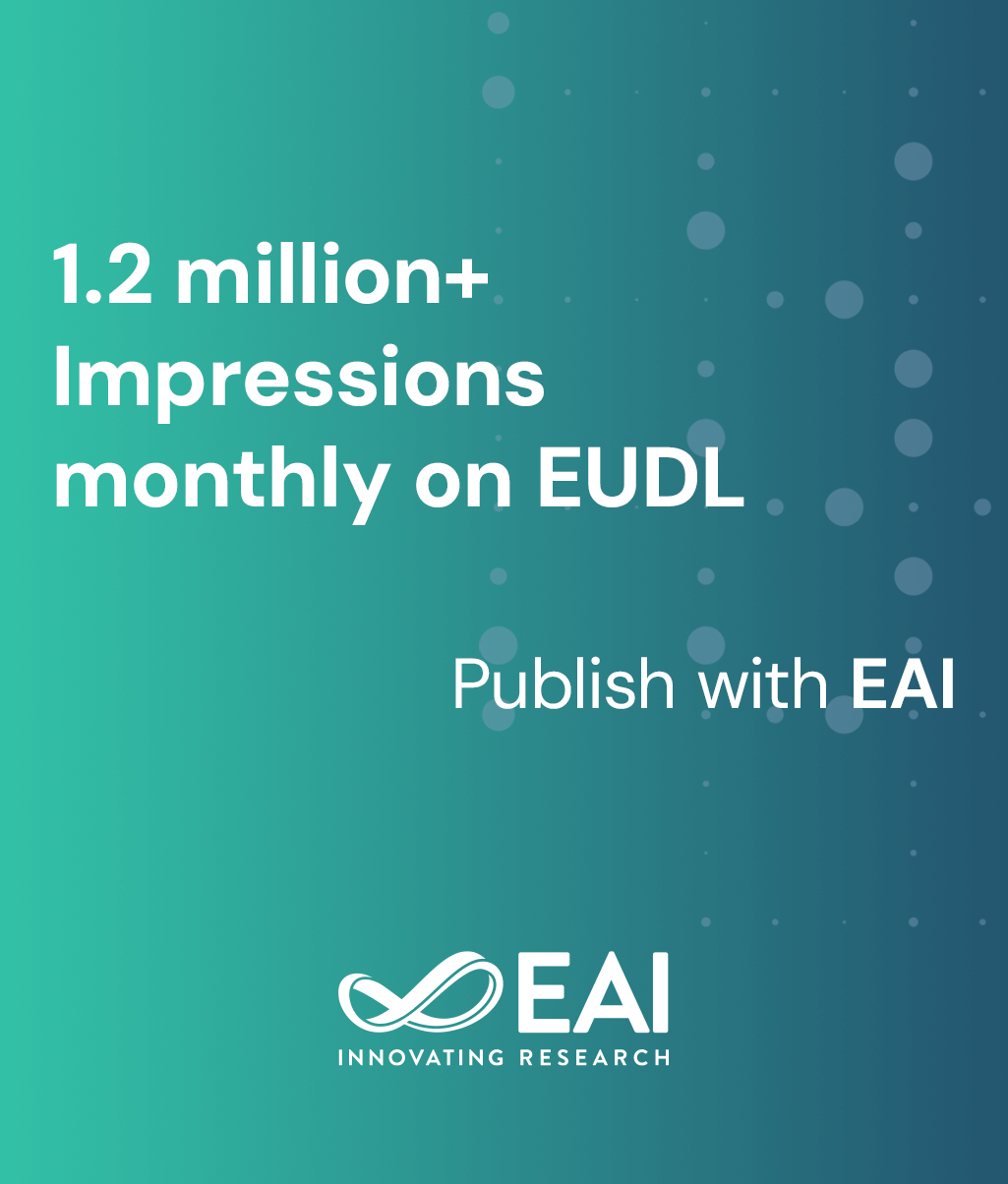
Research Article
Removing Background with Semantic Segmentation Based on Ensemble Learning
@INPROCEEDINGS{10.4108/eai.21-6-2018.2276586, author={Junhong Xu and Hanqing Guo and Aron Kageza and Shaoen Wu and Saeed AlQarni}, title={Removing Background with Semantic Segmentation Based on Ensemble Learning}, proceedings={11th EAI International Conference on Mobile Multimedia Communications}, publisher={EAI}, proceedings_a={MOBIMEDIA}, year={2018}, month={9}, keywords={deep learning image segmentation background removal}, doi={10.4108/eai.21-6-2018.2276586} }
- Junhong Xu
Hanqing Guo
Aron Kageza
Shaoen Wu
Saeed AlQarni
Year: 2018
Removing Background with Semantic Segmentation Based on Ensemble Learning
MOBIMEDIA
EAI
DOI: 10.4108/eai.21-6-2018.2276586
Abstract
This paper presents a deep learning approach to Kaggle Carvana Image Masking Competition, which aims at extracting the car objects in high quality images with the background removed. We formulate the background extraction problem as an image segmentation problem. In this challenge, we have evaluated different U-Net architectures. We have explored two different techniques in combining encoder downsampling features with decoder upsampling features. In addition, we have experimented replacing different pre-trained networks to accelerate the training process. Finally, we have trained different models at different image scales and predicted the final result with the ensemble method. Our final method has placed us at top 4% in the challenge and achieved a dice coefficient score of 0.99694.