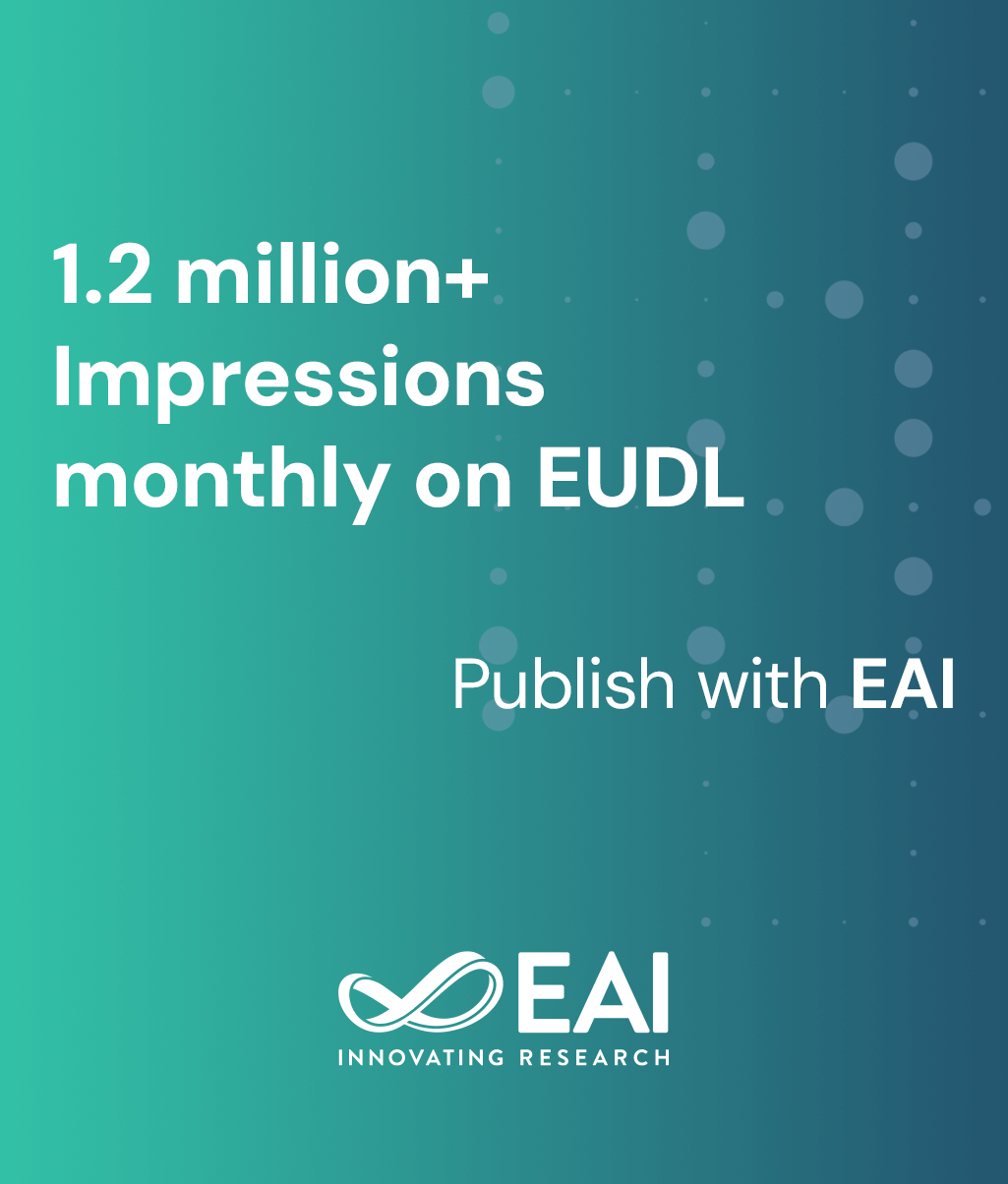
Research Article
Optimizing Human Pose Estimation Using a Simplified UNet Architecture: An Experimental Analysis on Depth and Width Parameters
@INPROCEEDINGS{10.4108/eai.21-11-2024.2354631, author={Shenghao Ren}, title={Optimizing Human Pose Estimation Using a Simplified UNet Architecture: An Experimental Analysis on Depth and Width Parameters}, proceedings={Proceedings of the 2nd International Conference on Machine Learning and Automation, CONF-MLA 2024, November 21, 2024, Adana, Turkey}, publisher={EAI}, proceedings_a={CONF-MLA}, year={2025}, month={3}, keywords={human pose estimation human keypoint detection network structure adjustment unet lsp dataset}, doi={10.4108/eai.21-11-2024.2354631} }
- Shenghao Ren
Year: 2025
Optimizing Human Pose Estimation Using a Simplified UNet Architecture: An Experimental Analysis on Depth and Width Parameters
CONF-MLA
EAI
DOI: 10.4108/eai.21-11-2024.2354631
Abstract
Human pose estimation (HPE) is a significant problem in the field of computer vision, with wide applications in action recognition, intelligent surveillance, and other areas. With the development of deep learning, the accuracy of pose estimation has significantly improved. However, high-precision pose estimation models typically have complex network structures and high computational costs, making them difficult to apply in resource-constrained or real-time scenarios. To address this issue, this paper proposes a simple convolutional neural network named SimpleUNet based on UNet, utilizing a dataset of 2,000 athlete images and their annotated images with 14 visualized joints to perform human keypoint detection tasks. In SimpleUNet, we designed two adjustable parameters to control the depth and width of the network structure: the number of convolutional modules in the encoder and decoder, which defines the depth, and the number of channels in the network, which defines the width. We adjusted the depth from 10 to 100 in steps of 10 and the width from 1 to 9 in steps of 1, conducting a total of 90 experiments. We recorded the best model as well as information on loss, accuracy, and mIoU to analyze the relationship between the complexity of the model network and its performance in human keypoint detection. We ultimately found that moderate depth and width provide the best pose estimation performance, while excessively large or small depth and width each have their drawbacks.