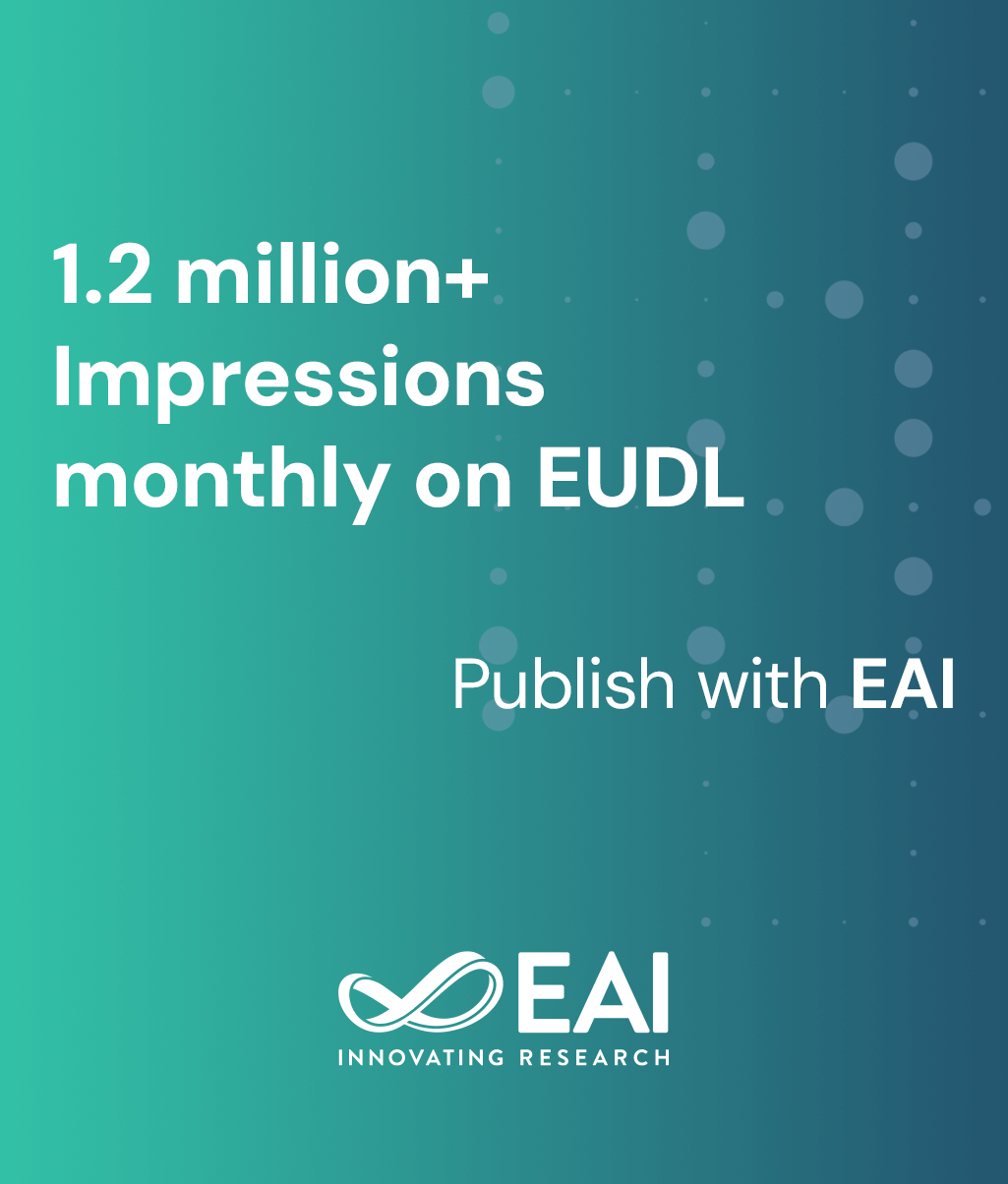
Research Article
Segmentation Algorithm for Cancer Regions in Breast Cancer MRI Images Based on the Improved U2-Net Network
@INPROCEEDINGS{10.4108/eai.21-11-2024.2354611, author={Ye Lin and Zongyan Dai and Qi Jing and Rui Shi}, title={Segmentation Algorithm for Cancer Regions in Breast Cancer MRI Images Based on the Improved U2-Net Network}, proceedings={Proceedings of the 2nd International Conference on Machine Learning and Automation, CONF-MLA 2024, November 21, 2024, Adana, Turkey}, publisher={EAI}, proceedings_a={CONF-MLA}, year={2025}, month={3}, keywords={u\textbackslash(\^{}2\textbackslash)-net network; attention mechanism; dense connections; mri medical image segmentation}, doi={10.4108/eai.21-11-2024.2354611} }
- Ye Lin
Zongyan Dai
Qi Jing
Rui Shi
Year: 2025
Segmentation Algorithm for Cancer Regions in Breast Cancer MRI Images Based on the Improved U2-Net Network
CONF-MLA
EAI
DOI: 10.4108/eai.21-11-2024.2354611
Abstract
Around the world, breast cancer is the most common cancer in women disease. In this paper, an enhanced segmentation algorithm based on improved U (^2) -Net network is proposed, which integrates the convolutional block attention module and dense connection to enhance the efficiency of feature extraction and segmentation of the breast cancer MRI images. Additionally, the model in this paper was rigorously evaluated using a comprehensive dataset of breast cancer MRI images, including both privately labeled and publicly labeled data. Our experimental results and the ablation experiments demonstrate that the integration of CBAM module and dense connection present the significant optimization improvement. Besides, the model in this paper provides a robust framework on the basis of achieving high segmentation accuracy, which can have a larger optimization space in the field of medical image segmentation as well.