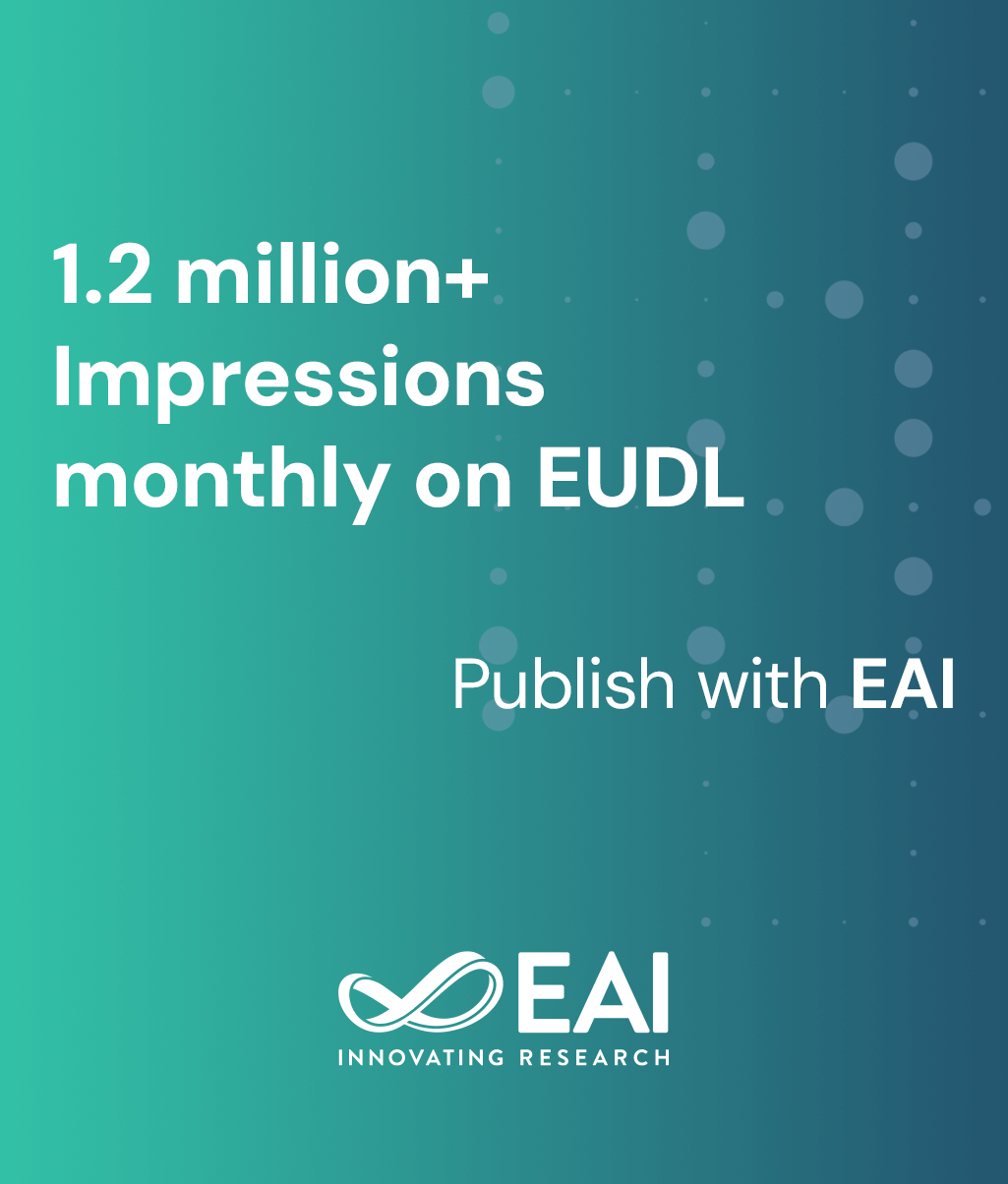
Research Article
Enhancing Emotion Recognition Accuracy with CFRSN-LSTM
@INPROCEEDINGS{10.4108/eai.21-11-2024.2354597, author={Yidan Zhang and Yangyue Zheng}, title={Enhancing Emotion Recognition Accuracy with CFRSN-LSTM}, proceedings={Proceedings of the 2nd International Conference on Machine Learning and Automation, CONF-MLA 2024, November 21, 2024, Adana, Turkey}, publisher={EAI}, proceedings_a={CONF-MLA}, year={2025}, month={3}, keywords={multimodal emotion recognition convolutional feature residual network lstm (long short-term memory) self-attention}, doi={10.4108/eai.21-11-2024.2354597} }
- Yidan Zhang
Yangyue Zheng
Year: 2025
Enhancing Emotion Recognition Accuracy with CFRSN-LSTM
CONF-MLA
EAI
DOI: 10.4108/eai.21-11-2024.2354597
Abstract
Multimodal emotion recognition is a challenging problem partly because the loss of original semantic information during intra- and inter-modal interactions. In this paper we propose to a novel cross-modal fusion network based on self-attention and residual structure (CFNSR-LSTM) for multimodal emotion recognition. We first perform representation learning for audio and video modalities to obtain the spatio-temporal structural features of video frame sequences and the MFCC features of audio sequences by efficient ResNeXt and a simple and effective one-dimensional CNN method, respectively. We then feed the features of the audio and video modalities into the cross-modal blocks separately. The audio features are processed using LSTM to capture temporal characteristics, and the self-attention mechanism is employed for intra-modal feature selection, which enables efficient and adaptive interaction between the selected audio features and the video modality. The residual structure ensures the integrity of the original structural features of the video modality. Finally, we conduct experiments on the RAVDESS dataset to verify the effectiveness of the proposed method. The experimental results show that the proposed CFNSR-LSTM model, with a parameter count of 26.40M, achieves the best performance with an accuracy of 76.25% and outperforms other models.