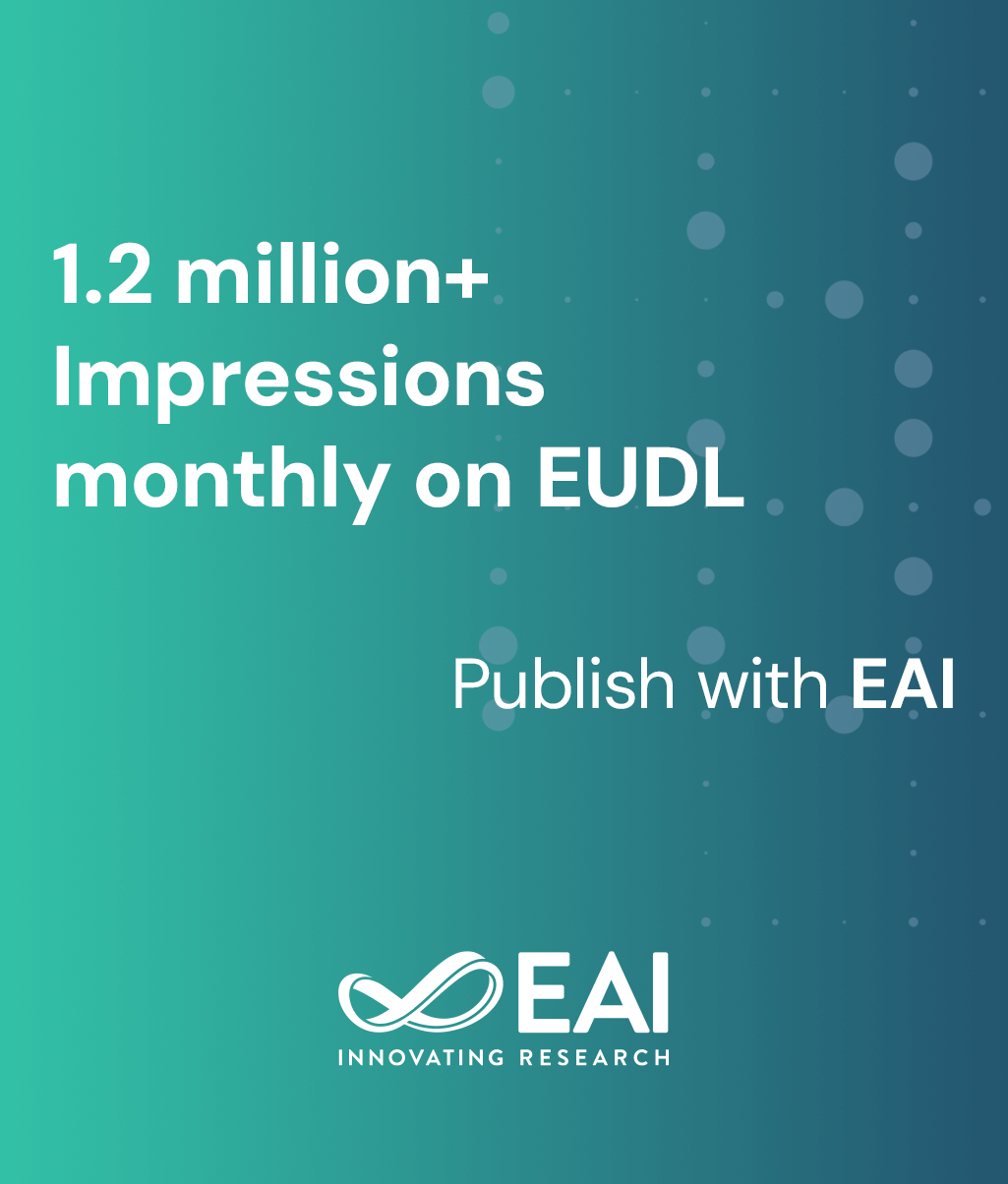
Research Article
Integrating Demographic, Clinical, and Behavioral Risk Factors for Cardiovascular Disease: A Random Forest Approach for Analysis, Prevention, and Prediction
@INPROCEEDINGS{10.4108/eai.21-11-2024.2354595, author={Ai Li and Fanrui Yang}, title={Integrating Demographic, Clinical, and Behavioral Risk Factors for Cardiovascular Disease: A Random Forest Approach for Analysis, Prevention, and Prediction}, proceedings={Proceedings of the 2nd International Conference on Machine Learning and Automation, CONF-MLA 2024, November 21, 2024, Adana, Turkey}, publisher={EAI}, proceedings_a={CONF-MLA}, year={2025}, month={3}, keywords={cardiovascular disease (cvd) risk prediction random forest mendelian randomization (mr) epidemiological data}, doi={10.4108/eai.21-11-2024.2354595} }
- Ai Li
Fanrui Yang
Year: 2025
Integrating Demographic, Clinical, and Behavioral Risk Factors for Cardiovascular Disease: A Random Forest Approach for Analysis, Prevention, and Prediction
CONF-MLA
EAI
DOI: 10.4108/eai.21-11-2024.2354595
Abstract
Cardiovascular disease (CVD) remains a critical health concern worldwide, posing a significant threat to human well-being. Previous studies have established that behavioral factors (e.g. alcohol consumption), specific clinical indicators, and demographic characteristics (e.g., CKD) are key determinants influencing the risk of CVD. To identify the most impactful predictive factors and further enhance the prevention and treatment of CVD, we analyzed two datasets containing various CVD-related factors. Following Exploratory Data Analysis (EDA), we utilized multiple models for prediction, including random forest, MLP, deepFM,XGBoost etc, using GridSearch for best performance. Our findings reveal that the best prediction model is Random Forest model. In dataset A, the primary factors are BMI, AgeCategory (age), SleepTime (sleep duration), GenHealth and PhysicalHealth. While in dataset B, which includes more clinically relevant features, the most significant predictors are HadAngina, State, AgeCategory, ChestScan and BMI. The comparative analysis of both datasets demonstrates that the dataset with more detailed clinical data (dataset B) yields more accurate predictions for CVD risk than the dataset focusing on just behavioral and demographic factors (dataset A). These findings highlight the importance of combining detailed clinical data with behavioral and demographic information to improve the precision of CVD risk prediction and management.