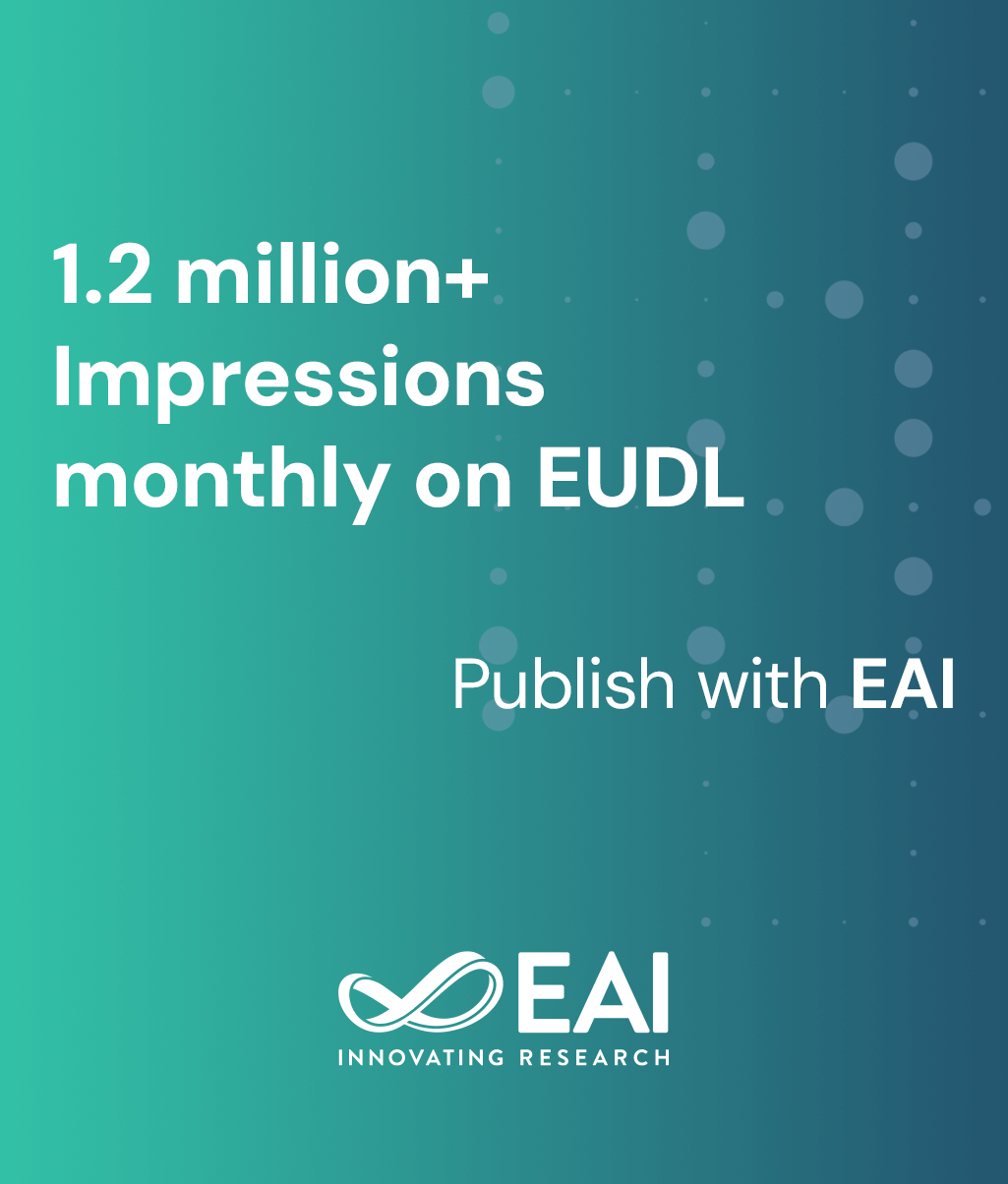
Research Article
Optimizing Web-Based AI Query Retrieval with GPT Integration in LangChain: A CoT-Enhanced Prompt Engineering Approach
@INPROCEEDINGS{10.4108/eai.21-11-2024.2354589, author={Wenqi Guan and Yang Fang}, title={Optimizing Web-Based AI Query Retrieval with GPT Integration in LangChain: A CoT-Enhanced Prompt Engineering Approach}, proceedings={Proceedings of the 2nd International Conference on Machine Learning and Automation, CONF-MLA 2024, November 21, 2024, Adana, Turkey}, publisher={EAI}, proceedings_a={CONF-MLA}, year={2025}, month={3}, keywords={remote learning retrieval prompt engineering chain-of-thought reasoning large language models}, doi={10.4108/eai.21-11-2024.2354589} }
- Wenqi Guan
Yang Fang
Year: 2025
Optimizing Web-Based AI Query Retrieval with GPT Integration in LangChain: A CoT-Enhanced Prompt Engineering Approach
CONF-MLA
EAI
DOI: 10.4108/eai.21-11-2024.2354589
Abstract
Large Language Models have brought a radical change in the process of remote learning students, among other aspects of educative activities. Current retrieval of remote learning resources lacks depth in contextual meaning that provides comprehensive information on complex student queries. This work proposes a novel approach to enhancing remote learning retrieval by integrating GPT-based models within the LangChain framework. We achieve this system in a more intuitive and productive manner using CoT reasoning and prompt engineering. The framework we propose puts much emphasis on increasing the precision and relevance of the retrieval results to return comprehensive and contextually enriched explanations and resources that best suit each student's needs. We also assess the effectiveness of our approach against paradigmatic LLMs and report improvements in user satisfaction and learning outcomes.