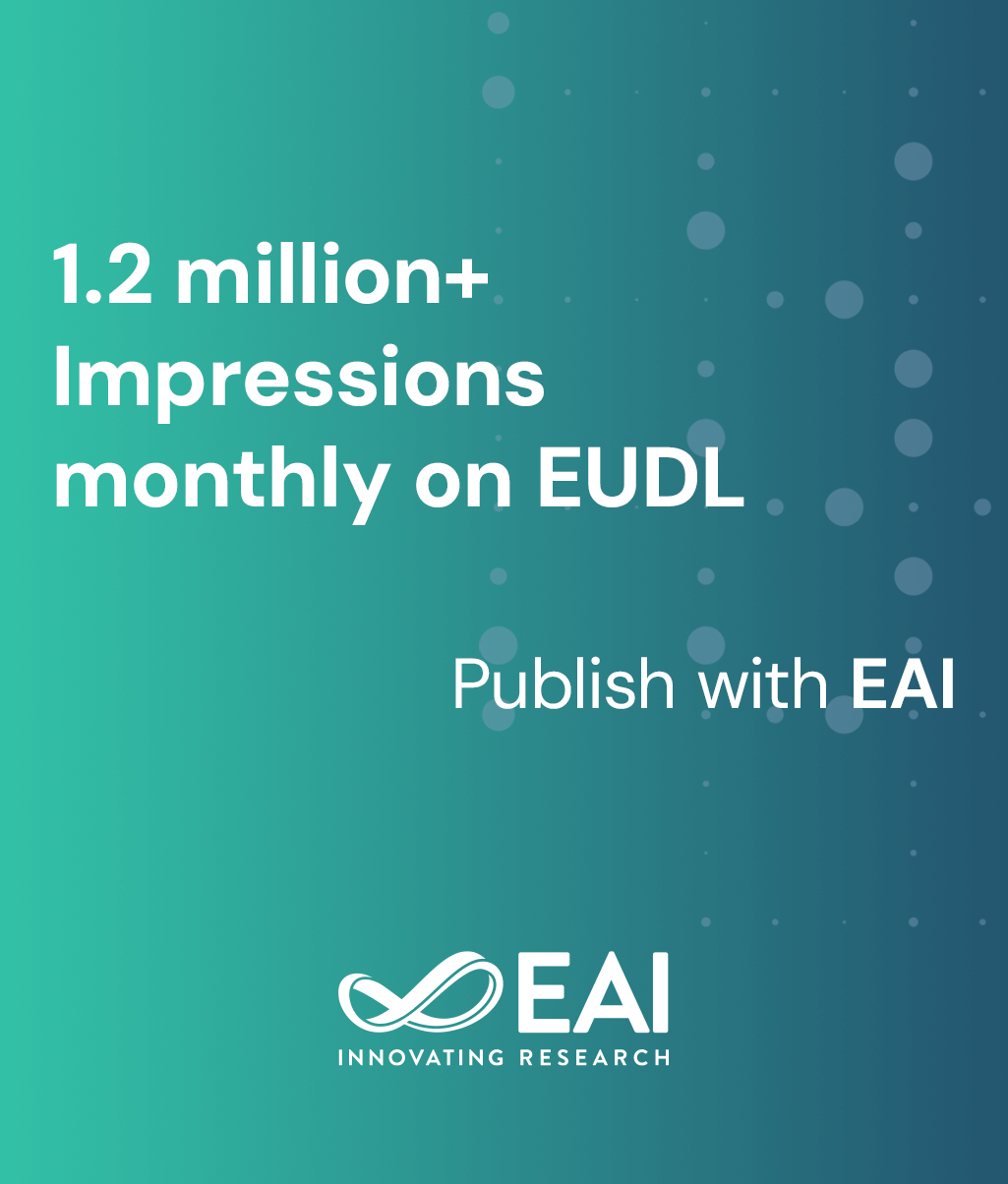
Research Article
XPose: Realism and Stability in Human Video Animations through ControlNet Integration of DensePose and DWPose
@INPROCEEDINGS{10.4108/eai.21-11-2024.2354584, author={Ziheng Jiang and Chentao Zhang}, title={XPose: Realism and Stability in Human Video Animations through ControlNet Integration of DensePose and DWPose}, proceedings={Proceedings of the 2nd International Conference on Machine Learning and Automation, CONF-MLA 2024, November 21, 2024, Adana, Turkey}, publisher={EAI}, proceedings_a={CONF-MLA}, year={2025}, month={3}, keywords={video generation human animation densepose controlnet}, doi={10.4108/eai.21-11-2024.2354584} }
- Ziheng Jiang
Chentao Zhang
Year: 2025
XPose: Realism and Stability in Human Video Animations through ControlNet Integration of DensePose and DWPose
CONF-MLA
EAI
DOI: 10.4108/eai.21-11-2024.2354584
Abstract
Enhancing the realism and stability of human animations in video generation remains a significant challenge, particularly in maintaining consistent facial expressions, body proportions, and clothing. This paper introduces a new framework, which addresses these challenges by integrating Dense Human Pose (DensePose) and Dynamic Warping Pose (DWPose) models within a ControlNet structure, called XPose. XPose combines mid-sample and down-sample ControlNet components to effectively guide the video generation process, while also exploring the impact of Lineart overlay on video quality. The framework dynamically adjusts the contributions of DensePose and DWPose through a weighted concatenation approach, with a 1.5:1 ratio identified as optimal based on quantitative evaluation using Fréchet Inception Distance (FID) and Learned Perceptual Image Patch Similarity (LPIPS) metrics. Evaluated on the TikTok dancing video dataset, XPose demonstrates significant improvements in the consistency and stability of generated animations, particularly in maintaining facial expressions and body sizes. Experimental results indicate that XPose outperforms existing methods such as MagicAnimate, achieving up to a 23.5% improvement in video fidelity under specific configurations. The findings suggest that XPose offers a robust solution for high-quality human video animations, with future research planned to explore the use of ControlNet images for further refinement.