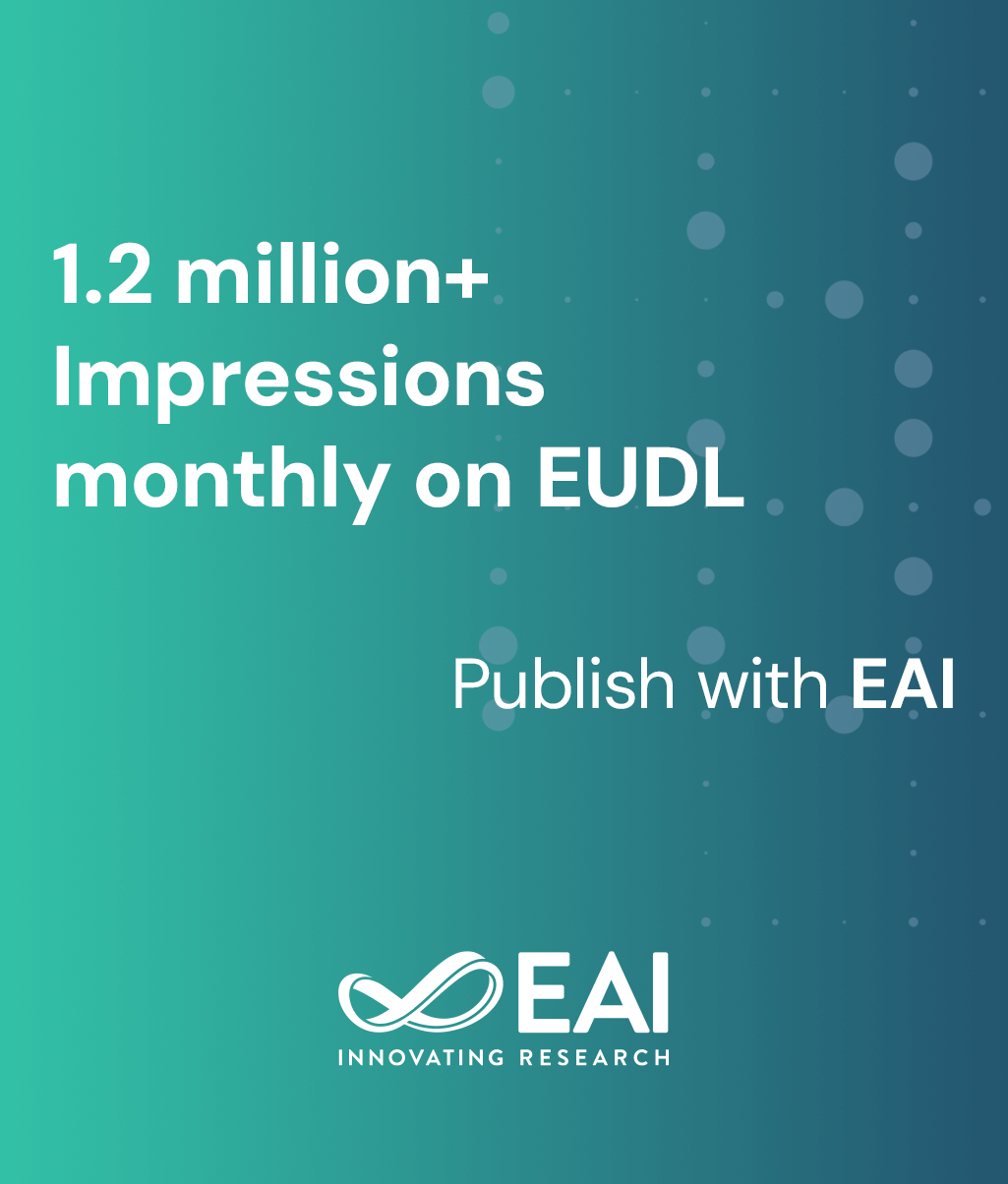
Research Article
LSTM-Driven Smart Home System: A Study of Temperature and Humidity Prediction and Energy Management
@INPROCEEDINGS{10.4108/eai.21-11-2024.2354581, author={Zhenpeng Lai}, title={LSTM-Driven Smart Home System: A Study of Temperature and Humidity Prediction and Energy Management}, proceedings={Proceedings of the 2nd International Conference on Machine Learning and Automation, CONF-MLA 2024, November 21, 2024, Adana, Turkey}, publisher={EAI}, proceedings_a={CONF-MLA}, year={2025}, month={3}, keywords={shs lstm temperature prediction humidity prediction energy management}, doi={10.4108/eai.21-11-2024.2354581} }
- Zhenpeng Lai
Year: 2025
LSTM-Driven Smart Home System: A Study of Temperature and Humidity Prediction and Energy Management
CONF-MLA
EAI
DOI: 10.4108/eai.21-11-2024.2354581
Abstract
Smart home systems (SHS) have gained significant popularity due to their inherent convenience. Using Internet of Things (IoT) technology, SHS can automatically adapt indoor environments according to user needs. However, most existing SHS rely on preset configurations and lack the ability to predict environmental changes, while also exhibiting shortcomings in energy management such as the ability to seamlessly transition between solar and natural gas. In response to these challenges, this paper introduces a SHS built on Long Short-Term Memory (LSTM) network models. By using publicly available datasets from the Kaggle platform for training and validation purposes, the LSTM model demonstrates exceptional performance in the tasks of temperature and humidity prediction as well as energy switching operation. It exhibits high accuracy and holds great potential for practical implementation within SHS applications. The research not only addresses the shortcomings of traditional SHS in environmental prediction and energy management by introducing the LSTM model but also provides an important theoretical foundation for future advancements in SHS. The results indicate that the LSTM model is capable of making precise predictions in intricate environmental conditions, optimizing energy utilization efficiency and promoting the development of smarter, energy-saving home systems.