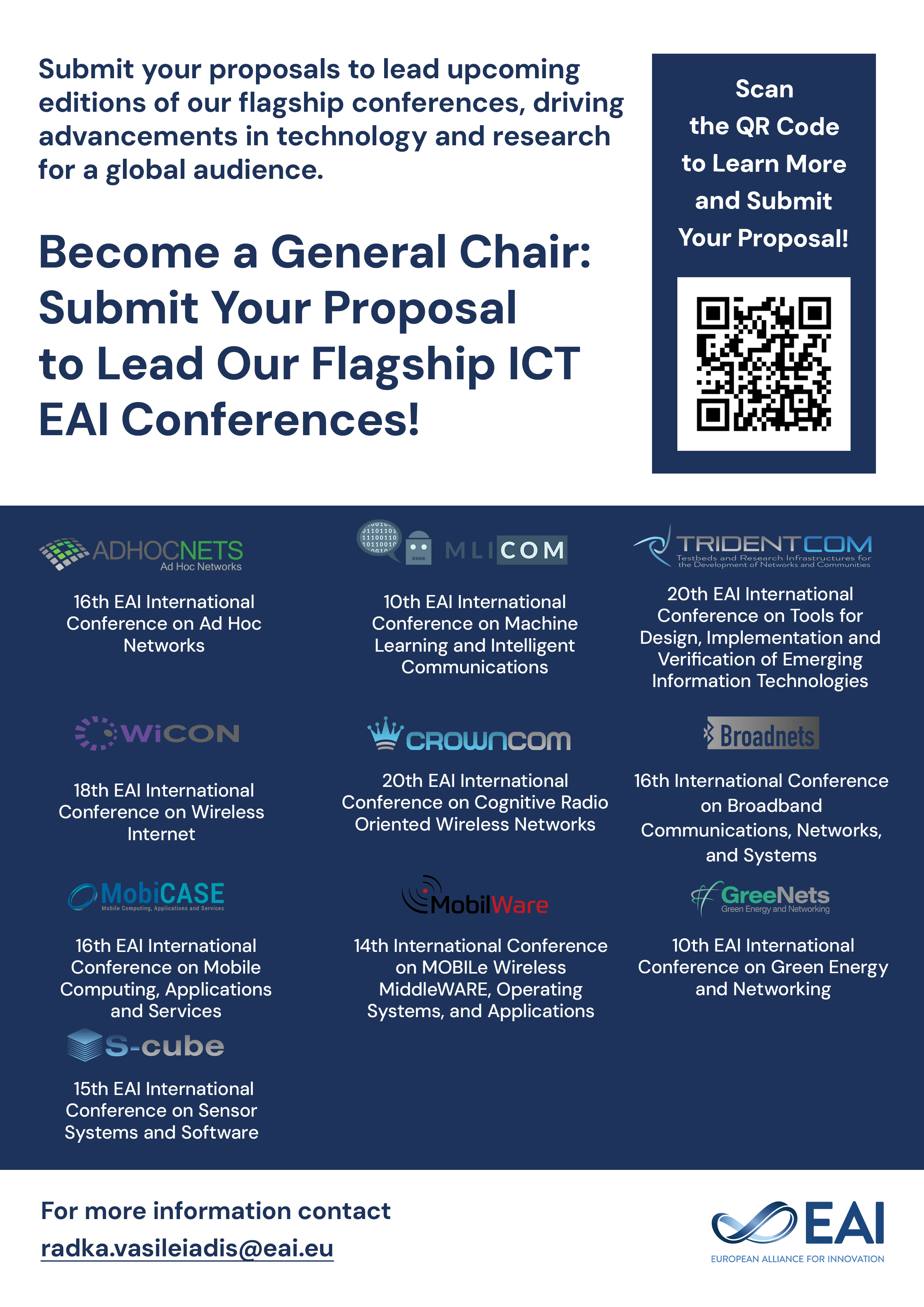
Research Article
Unobtrusive Measurement of Blood Pressure During Lifestyle Interventions
@INPROCEEDINGS{10.4108/eai.20-5-2019.2283509, author={Ariane Morassi Sasso and Suparno Datta and Bjarne Pfitzner and Lin Zhou and Nico Steckhan and Erwin Boettinger and Bert Arnrich}, title={Unobtrusive Measurement of Blood Pressure During Lifestyle Interventions}, proceedings={13th EAI International Conference on Pervasive Computing Technologies for Healthcare - Demos and Posters}, publisher={EAI}, proceedings_a={PERVASIVEHEALTH - EAI}, year={2019}, month={6}, keywords={wearables blood pressure estimation hypertension}, doi={10.4108/eai.20-5-2019.2283509} }
- Ariane Morassi Sasso
Suparno Datta
Bjarne Pfitzner
Lin Zhou
Nico Steckhan
Erwin Boettinger
Bert Arnrich
Year: 2019
Unobtrusive Measurement of Blood Pressure During Lifestyle Interventions
PERVASIVEHEALTH - EAI
EAI
DOI: 10.4108/eai.20-5-2019.2283509
Abstract
Hypertension is one of the most prevalent chronic diseases worldwide. Early diagnosis of this condition can prevent the incidence of stroke and also, cardiovascular diseases (CVDs) such as myocardial infarction and heart failure. Lifestyle interventions, such as intermittent fasting (IF), aim to lower blood pressure (BP) levels and increase the health of patients with cardiometabolic conditions. However, for monitoring BP, we still rely on a cuff that slows the flow of blood, which is both uncomfortable and makes continuous monitoring implausible. Recent research has shown that BP can be estimated using comfortable sensors such as the photoplethysmography (PPG) and the electrocardiography (ECG). Features that can be used for the estimation of BP are systolic upstroke time (SUT) and diastolic time (DT) extracted from the PPG signal, and pulse arrival and transit time (PAT/PTT) derived from the combination of ECG and PPG signals. In this paper we present: (1) a study design to collect continuous physiological signals, before and after a 7-days intermittent fasting (IF) intervention from both cardiometabolic and non-hypertensive patients using wearable devices and (2) initial results for predicting continuous blood pressure from the PPG and ECG signals using statistical and machine learning methods.