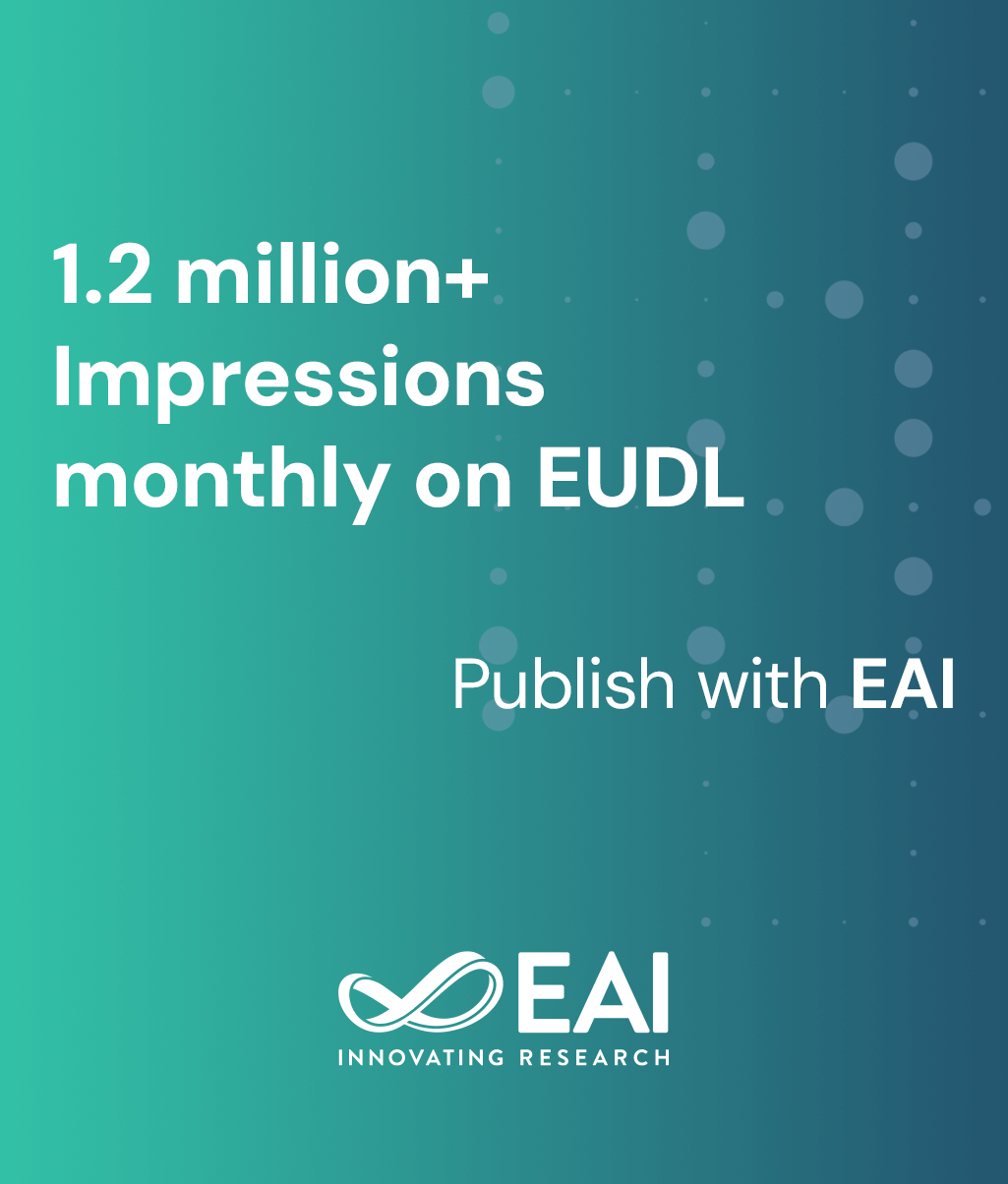
Research Article
The Bootstrap Stratified Random Sampling in Finite Population for Traffic Survey Data
@INPROCEEDINGS{10.4108/eai.2-8-2019.2290544, author={Kristiana Yunitaningtyas and Indahwati Indahwati and Muhammad Nur Aidi and Santi Susanti}, title={The Bootstrap Stratified Random Sampling in Finite Population for Traffic Survey Data}, proceedings={Proceedings of the 1st International Conference on Statistics and Analytics, ICSA 2019, 2-3 August 2019, Bogor, Indonesia}, publisher={EAI}, proceedings_a={ICSA}, year={2020}, month={1}, keywords={bootstrap finite population stratified random sampling traffic survey}, doi={10.4108/eai.2-8-2019.2290544} }
- Kristiana Yunitaningtyas
Indahwati Indahwati
Muhammad Nur Aidi
Santi Susanti
Year: 2020
The Bootstrap Stratified Random Sampling in Finite Population for Traffic Survey Data
ICSA
EAI
DOI: 10.4108/eai.2-8-2019.2290544
Abstract
Traffic survey is an important technique used to measure traffic density and gas emissions produced by vehicles but generally it is carried out for a long period of time. This study aims to apply stratified random sampling to traffic survey data so as to improve the process of data collection and efficiency with a high degree of accuracy. The data is divided into strata based on traffic density and is implemented using the direct bootstrap resampling technique by paying attention to the finite population correction factor. The bootstrap in finite population is expected to resolve the overestimate variance due to the standard bootstrap. Evaluation is done by looking at the criteria of validity, reliability, and accuracy of the bootstrap statistics. The results indicated that the bias and variance decrease when bootstrap replication is large. Bootstrap sample size of 32 produced the lowest distribution of bias, adjusted variance, and MSE value.