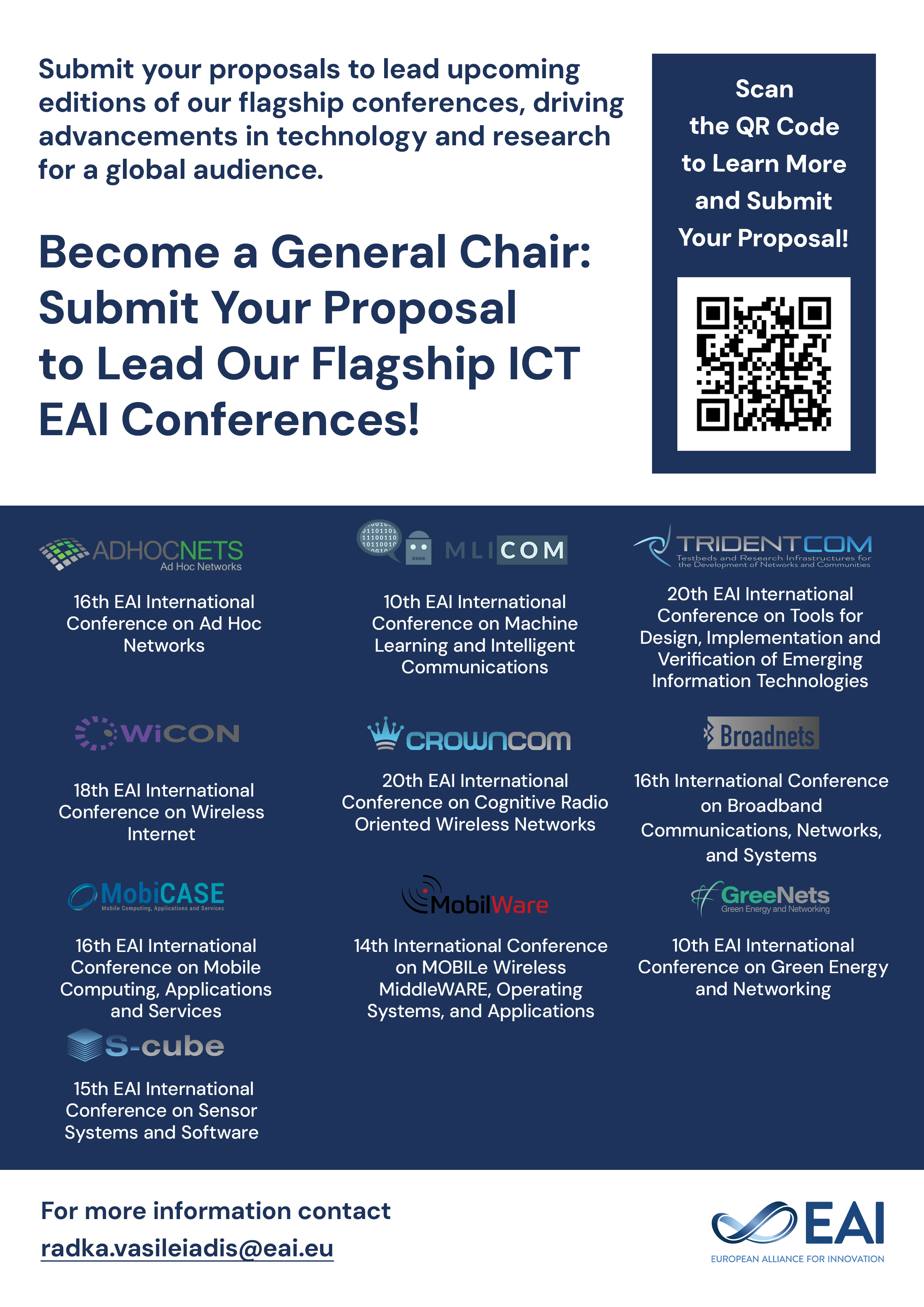
Research Article
Combining Result of PCR and PLSR Statistical Downscaling with Quadratic Optimization for Improving Estimation
@INPROCEEDINGS{10.4108/eai.2-8-2019.2290531, author={Praditya Puspitaninggar and Aji Hamim Wigena and Anwar Fitrianto}, title={Combining Result of PCR and PLSR Statistical Downscaling with Quadratic Optimization for Improving Estimation}, proceedings={Proceedings of the 1st International Conference on Statistics and Analytics, ICSA 2019, 2-3 August 2019, Bogor, Indonesia}, publisher={EAI}, proceedings_a={ICSA}, year={2020}, month={1}, keywords={combined forecast partial least square regression principal component regression statistical downscaling quadratic optimization}, doi={10.4108/eai.2-8-2019.2290531} }
- Praditya Puspitaninggar
Aji Hamim Wigena
Anwar Fitrianto
Year: 2020
Combining Result of PCR and PLSR Statistical Downscaling with Quadratic Optimization for Improving Estimation
ICSA
EAI
DOI: 10.4108/eai.2-8-2019.2290531
Abstract
Statistical downscaling (SDS) using principal component regression (PCR) and partial least square regression (PLSR) used to estimate rainfall. The accuracy measurement of these models was done by calculating root mean square error of prediction (RMSEP) value. The smaller RMSEP value, the closer estimate to actual rainfall. The RMSEP value of PCR and PLSR models tend to be large, so it needs a method to improve the accuracy of estimation. Improving accuracy of rainfall estimate was done by combining the two estimates with the SDS models and minimizing the combined RMSEP as an objective function with or without constraints. The optimization with constraints resulted in the combined RMSEPs larger than the RMSEPs of the two models on few datasets, while the optimization without constraints resulted in the combined RMSEPs smaller than the RMSEPs of the two models on all datasets. The combined rainfall estimate was better than rainfall estimates forming it.