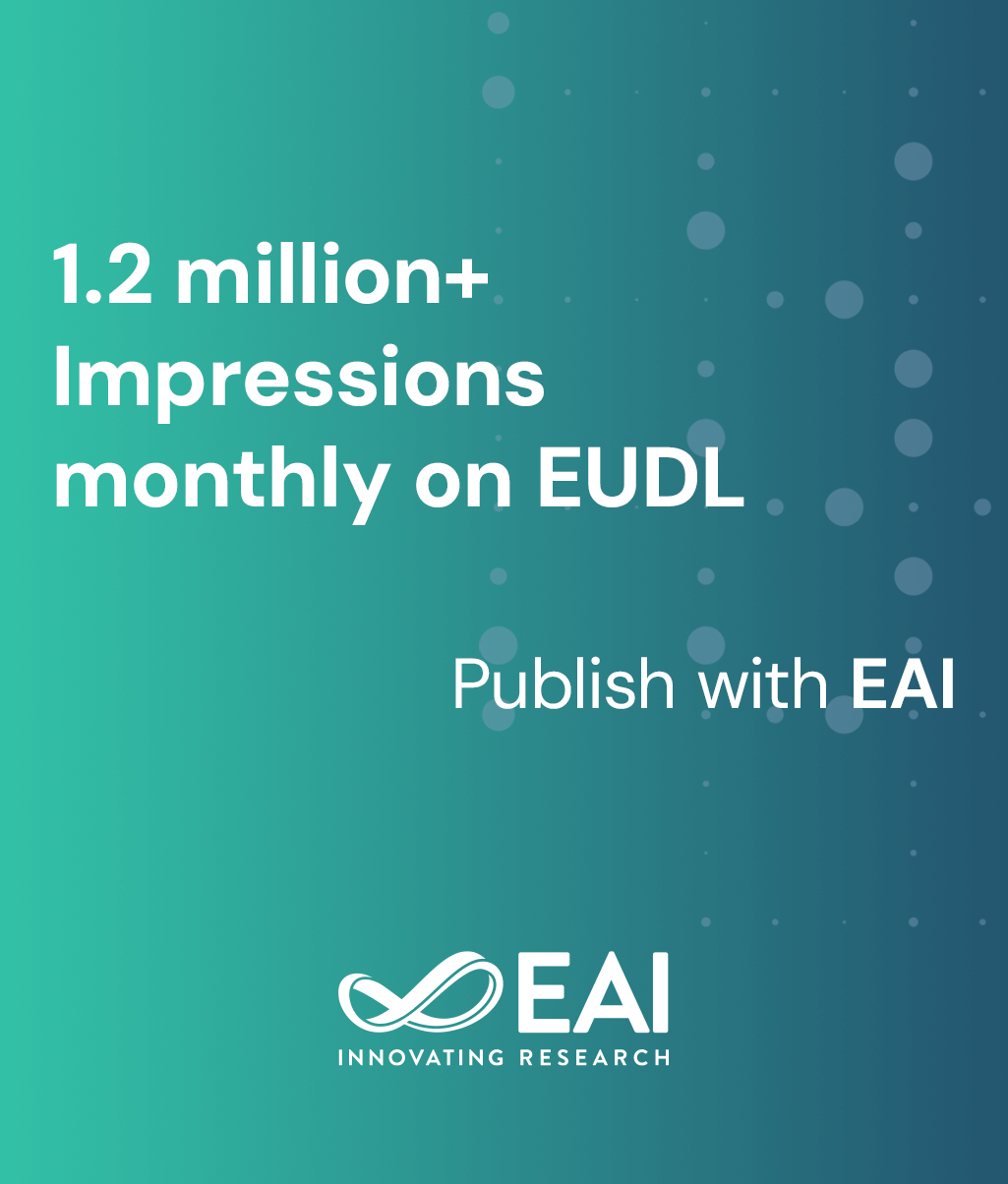
Research Article
Bayes Risk Post-Pruning in Decision Tree to Overcome Overfitting Problem on Customer Churn Classification
@INPROCEEDINGS{10.4108/eai.2-8-2019.2290487, author={Devina Christianti and Sarini Abdullah and Siti Nurrohmah}, title={Bayes Risk Post-Pruning in Decision Tree to Overcome Overfitting Problem on Customer Churn Classification}, proceedings={Proceedings of the 1st International Conference on Statistics and Analytics, ICSA 2019, 2-3 August 2019, Bogor, Indonesia}, publisher={EAI}, proceedings_a={ICSA}, year={2020}, month={1}, keywords={binary class c45 algorithm entropy gain ratio zero-one-loss}, doi={10.4108/eai.2-8-2019.2290487} }
- Devina Christianti
Sarini Abdullah
Siti Nurrohmah
Year: 2020
Bayes Risk Post-Pruning in Decision Tree to Overcome Overfitting Problem on Customer Churn Classification
ICSA
EAI
DOI: 10.4108/eai.2-8-2019.2290487
Abstract
Classification is the process of assigning a set of data into an existing class. Decision tree is claimed to be faster and produces better accuracy compared to another classifier. However, it has some drawbacks in which the classifier is susceptible to overfitting. This problem can be avoided by post-pruning that trimming the small influence subtree in conducting the classification to improve model performance in predicting data. This paper proposes a Post-Pruning method by applying Bayes Risk, in which the risk estimation of each parent node compared with its leaf. This method is applied to two datasets of customer churn classification from the Kaggle site and IBM Datasets with three different sizes for training dataset (60%, 70%, and 80%). For the result, Bayes Risk Post-Pruning can improve decision tree performance and the larger the size of the training dataset was associated with higher accuracy, precision, and recall of the model.