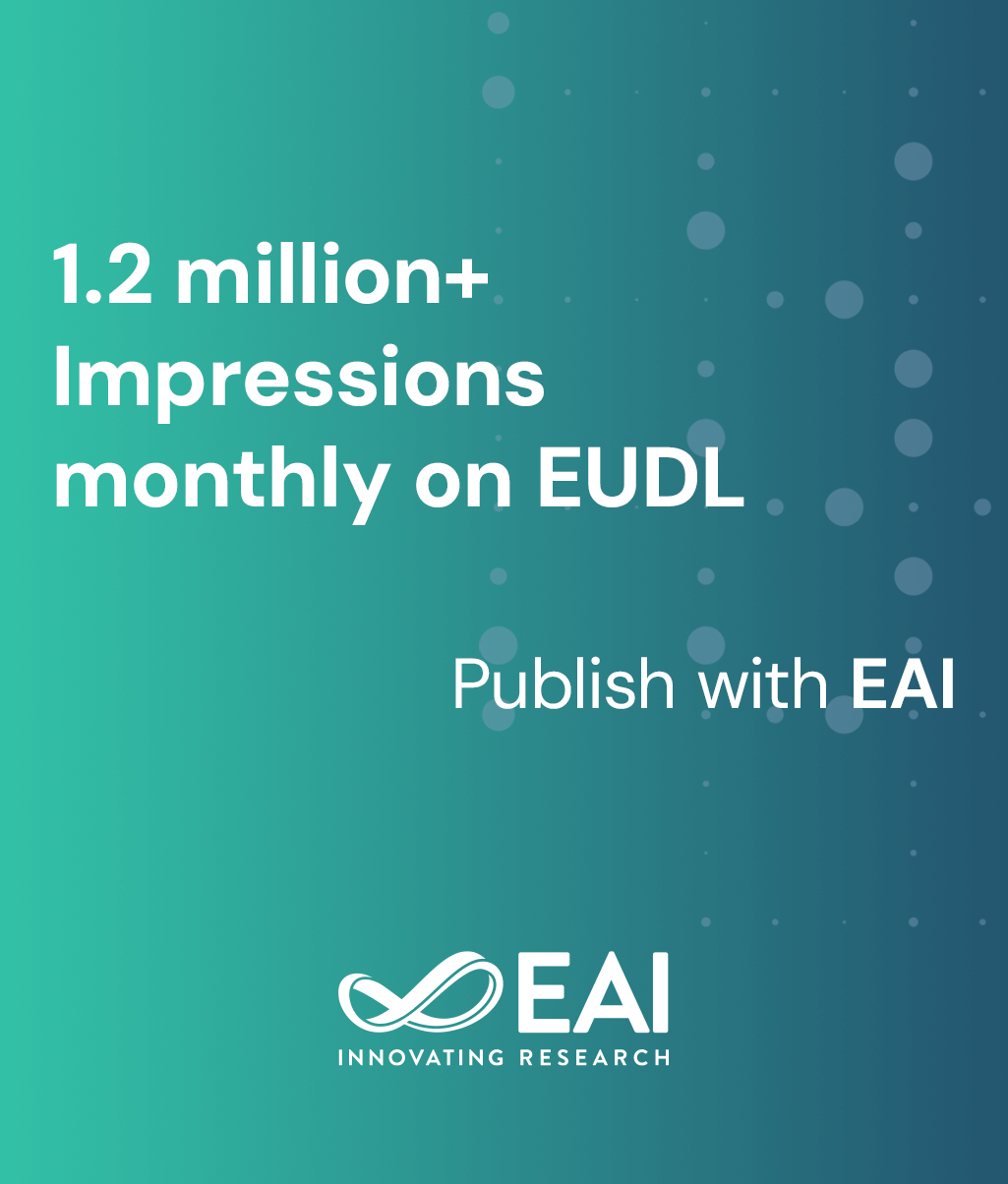
Research Article
Unordered Features Selection of Low Birth WeightDatain Indonesiausing the LASSO and Fused LASSO Techniques
@INPROCEEDINGS{10.4108/eai.2-8-2019.2290344, author={Yenni Kurniawati and Khairil Anwar Notodiputro and Bagus Sartono}, title={Unordered Features Selection of Low Birth WeightDatain Indonesiausing the LASSO and Fused LASSO Techniques}, proceedings={Proceedings of the 1st International Conference on Statistics and Analytics, ICSA 2019, 2-3 August 2019, Bogor, Indonesia}, publisher={EAI}, proceedings_a={ICSA}, year={2020}, month={1}, keywords={fused lasso lasso low birth weight penalized regression unordered features}, doi={10.4108/eai.2-8-2019.2290344} }
- Yenni Kurniawati
Khairil Anwar Notodiputro
Bagus Sartono
Year: 2020
Unordered Features Selection of Low Birth WeightDatain Indonesiausing the LASSO and Fused LASSO Techniques
ICSA
EAI
DOI: 10.4108/eai.2-8-2019.2290344
Abstract
This paper aims to analyze the Low Birth Weight (LBW) data of infants in Indonesia by using the LASSO and Fused LASSO techniques. Fused LASSO is usually used to select parameters for ordered features. In this case, the features are unordered. Therefore, this research adopts three techniques in ordering features. Furthermore, all these Fused LASSO techniques and LASSO are compared. This paper utilizes data on 1,176 LBW infants collected from the 2017 Indonesian Demographic and Health Survey (IDHS). The results showed that LASSO has the sparsest solutionbased on the 5-fold cross-validation. Thefeatures that contribute to LBW are mothers' occupation, mothers' age, antenatal care, multiple birth, and birth order. However, Fused LASSO 1 has the lowest AIC and BIC valuecompared to other ordering techniques.Ordering features using the correlation between the features and the outcomes is recommended as an alternative technique to sort unordered features.