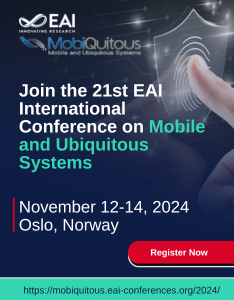
Research Article
Bayesian LASSO Quantile Regression: An Application to the Modeling of Low Birth Weight
@INPROCEEDINGS{10.4108/eai.2-8-2019.2290341, author={Ferra Yanuar and Aidinil Zetra and Arrival Rince Putri and Yudiantri Asdi}, title={Bayesian LASSO Quantile Regression: An Application to the Modeling of Low Birth Weight}, proceedings={Proceedings of the 1st International Conference on Statistics and Analytics, ICSA 2019, 2-3 August 2019, Bogor, Indonesia}, publisher={EAI}, proceedings_a={ICSA}, year={2020}, month={1}, keywords={bayesian lasso quantile regression low birth weight normality assumption outliers quantile regression}, doi={10.4108/eai.2-8-2019.2290341} }
- Ferra Yanuar
Aidinil Zetra
Arrival Rince Putri
Yudiantri Asdi
Year: 2020
Bayesian LASSO Quantile Regression: An Application to the Modeling of Low Birth Weight
ICSA
EAI
DOI: 10.4108/eai.2-8-2019.2290341
Abstract
The modeling of low birth weight using ordinary least square is not appropriate and inefficient. The low birth weight data violates the normality assumption since the data is right skewed. The data usually contains outliers as well. Many researchers used quantile regression approach to model this case but this method has limitation. The limitation of this approach is need moderate to big sample size. This study aims to combine the quantile regression with Bayesian LASSO approach to model the low birth weight. Bayesian method has ability to model small sample size since it involves the information related to data (known as likelihood function) and prior information about the parameter tobe estimated (prior distribution). This study demonstrated that Bayesian quantile regression and Bayesian LASSO (Least Absolute Shrinkage Selection Operator)quantile regression could yield the acceptable model of low birth weight case based on indicators of goodness of fit model. Bayesian LASSO quantile regression produced better estimated parameter values since it yielded shorter 95% Bayesian credible interval than Bayesian quantile regression.