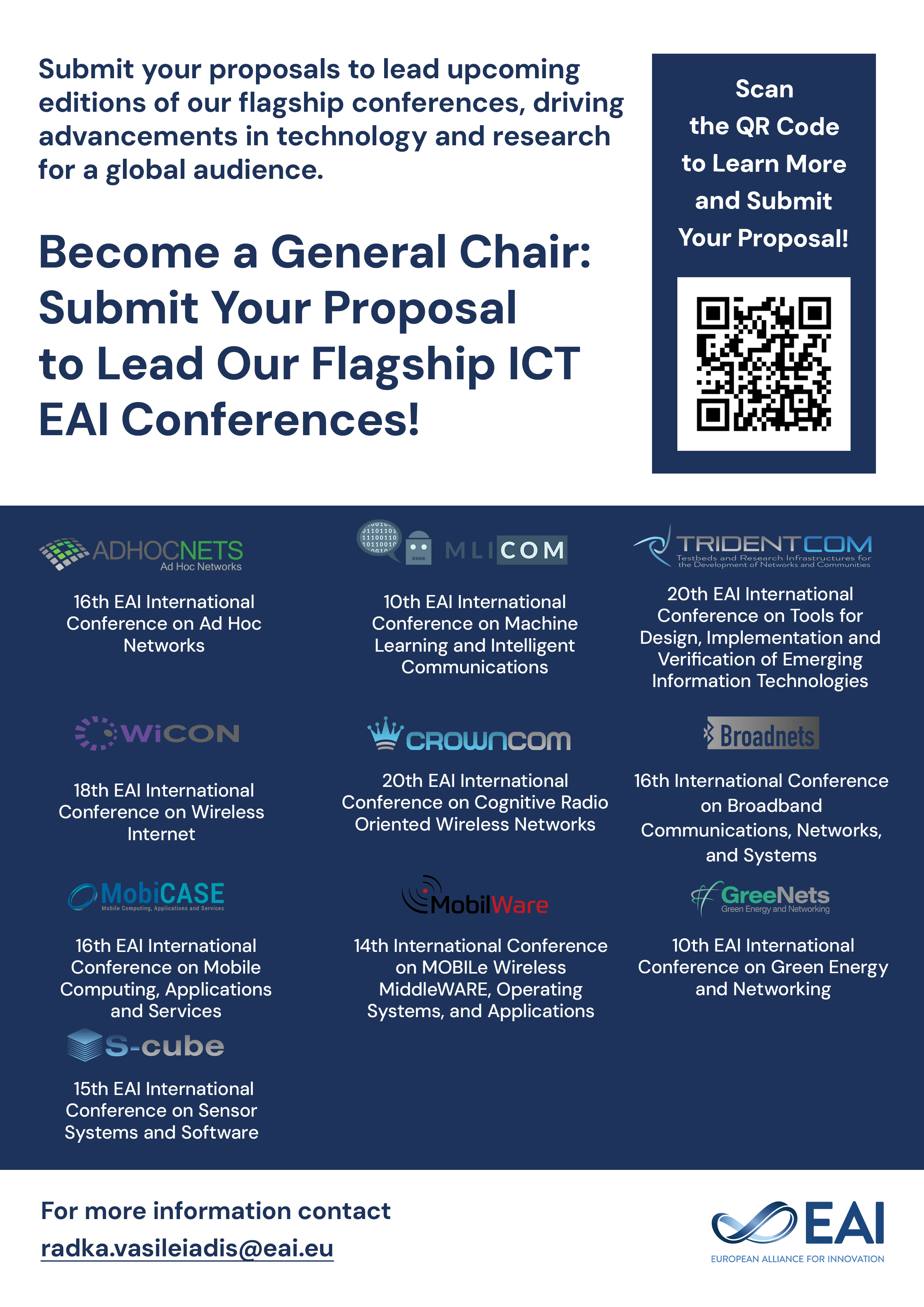
Research Article
Research on Product Marketing Recommendation Methods Based on Maximizing Individual User Influence in the Context of Globalization
@INPROCEEDINGS{10.4108/eai.2-6-2023.2334690, author={Zefeng Shao and Dalong Yu}, title={Research on Product Marketing Recommendation Methods Based on Maximizing Individual User Influence in the Context of Globalization}, proceedings={Proceedings of the 2nd International Conference on Information Economy, Data Modeling and Cloud Computing, ICIDC 2023, June 2--4, 2023, Nanchang, China}, publisher={EAI}, proceedings_a={ICIDC}, year={2023}, month={8}, keywords={product marketing; greedy; influence maximization; mixed integer programming algorithm; social influence}, doi={10.4108/eai.2-6-2023.2334690} }
- Zefeng Shao
Dalong Yu
Year: 2023
Research on Product Marketing Recommendation Methods Based on Maximizing Individual User Influence in the Context of Globalization
ICIDC
EAI
DOI: 10.4108/eai.2-6-2023.2334690
Abstract
Product marketing recommendation is an important tool for companies to gain competitive advantage and increase market share in the context of globalization, how to accurately recommend products to users has become a challenging problem. In view of this, the study proposes a product marketing recommendation method based on influence maximization, in which the process overlaps user influence, introduces greedy greedy algorithm for calculation and optimization, and performs accurate recommendation marketing by analyzing the user's degree of hesitation and interest. The experimental results show that the research method can reach a maximum of 3.4, 12 and 2.3 in the three datasets when conducting influence gain test; when conducting influence calculation number test, the maximum number of calculations of the research method is 726 when the node subset size is 40; the running time of the research method is only about 30ms in the Viki-Vote dataset when the number of companies is 1; the research The study method achieves a user purchase rate increase of 19% or more in all five companies. All of these results show that the research method has good performance in product marketing recommendation and can achieve significant increase in user purchase rate, which provides a new method reference for product marketing recommendation.