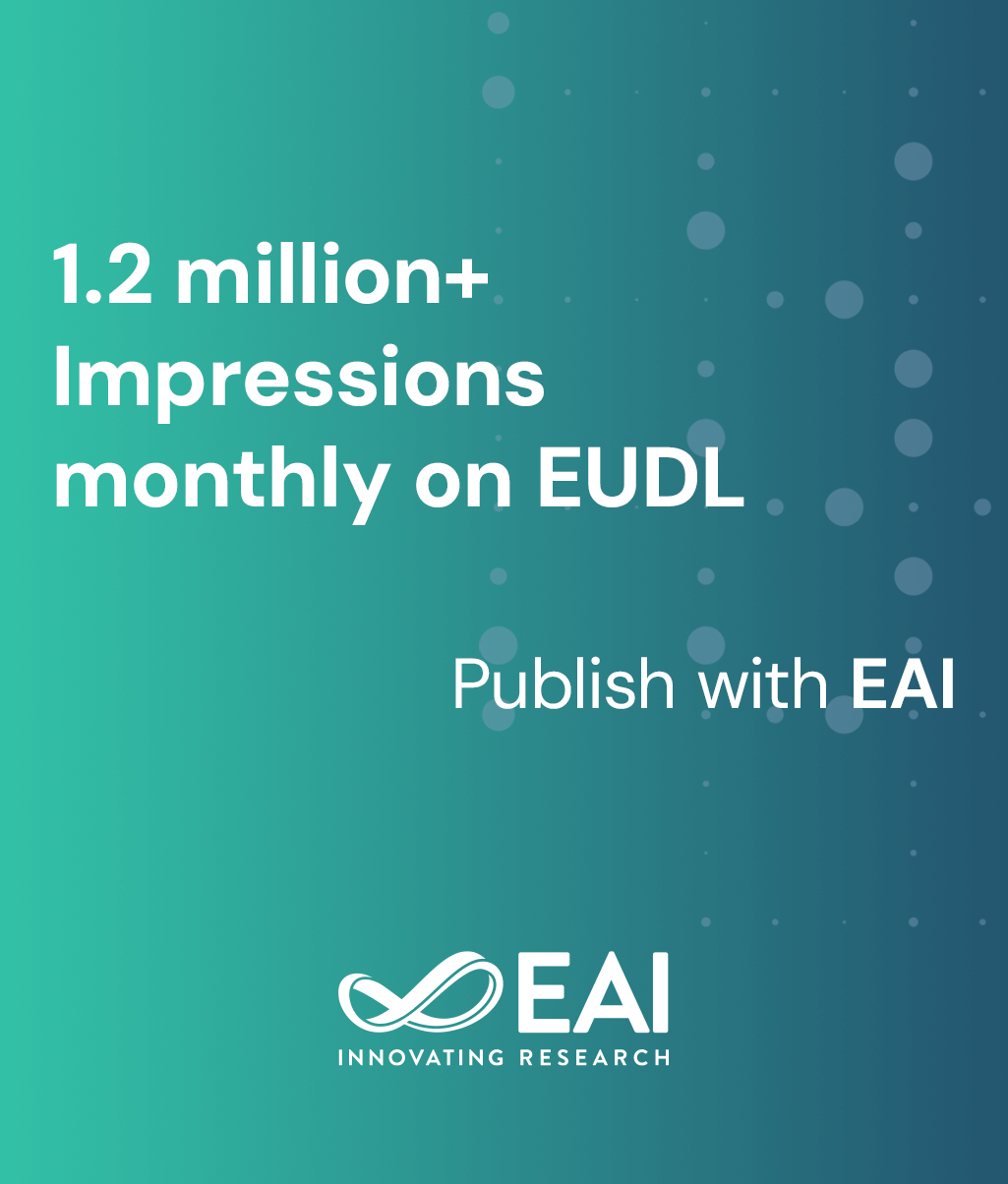
Research Article
A LightGBM Based Default Prediction Method for American Express
@INPROCEEDINGS{10.4108/eai.2-6-2023.2334590, author={Zhiren Gan and Junyuan Qiu and Fuli Li and Qian Liang}, title={A LightGBM Based Default Prediction Method for American Express}, proceedings={Proceedings of the 2nd International Conference on Information Economy, Data Modeling and Cloud Computing, ICIDC 2023, June 2--4, 2023, Nanchang, China}, publisher={EAI}, proceedings_a={ICIDC}, year={2023}, month={8}, keywords={credit default feature engineering lightgbm}, doi={10.4108/eai.2-6-2023.2334590} }
- Zhiren Gan
Junyuan Qiu
Fuli Li
Qian Liang
Year: 2023
A LightGBM Based Default Prediction Method for American Express
ICIDC
EAI
DOI: 10.4108/eai.2-6-2023.2334590
Abstract
With the progress of economy and science and technology, the credit card business has developed rapidly in the financial industry because of its convenient and high profits. However, with the sharp increase in the number of credit card users, the problem of credit card violations has become more prominent. If corresponding measures are not taken in a timely manner to control it, it will cause serious losses to banks and other financial institutions. The task of predicting personal default risk can be seen as a binary classification task. In this study, we utilize data provided by the American Express Company to predict default and mitigate the default risk for consumer finance companies using a model called LightGBM. We discuss related work in the second section, while our methodology and experiments are presented in sections III and IV. In order to assess the performance of our experiments, we conduct experiments using different types of models. We also define new experimental metrics. The results indicate that among these models, LightGBM achieved the highest metric of 0.692, surpassing Xgboost, Lasso, and Catboost by 0.007, 0.032, and 0.008 respectively.