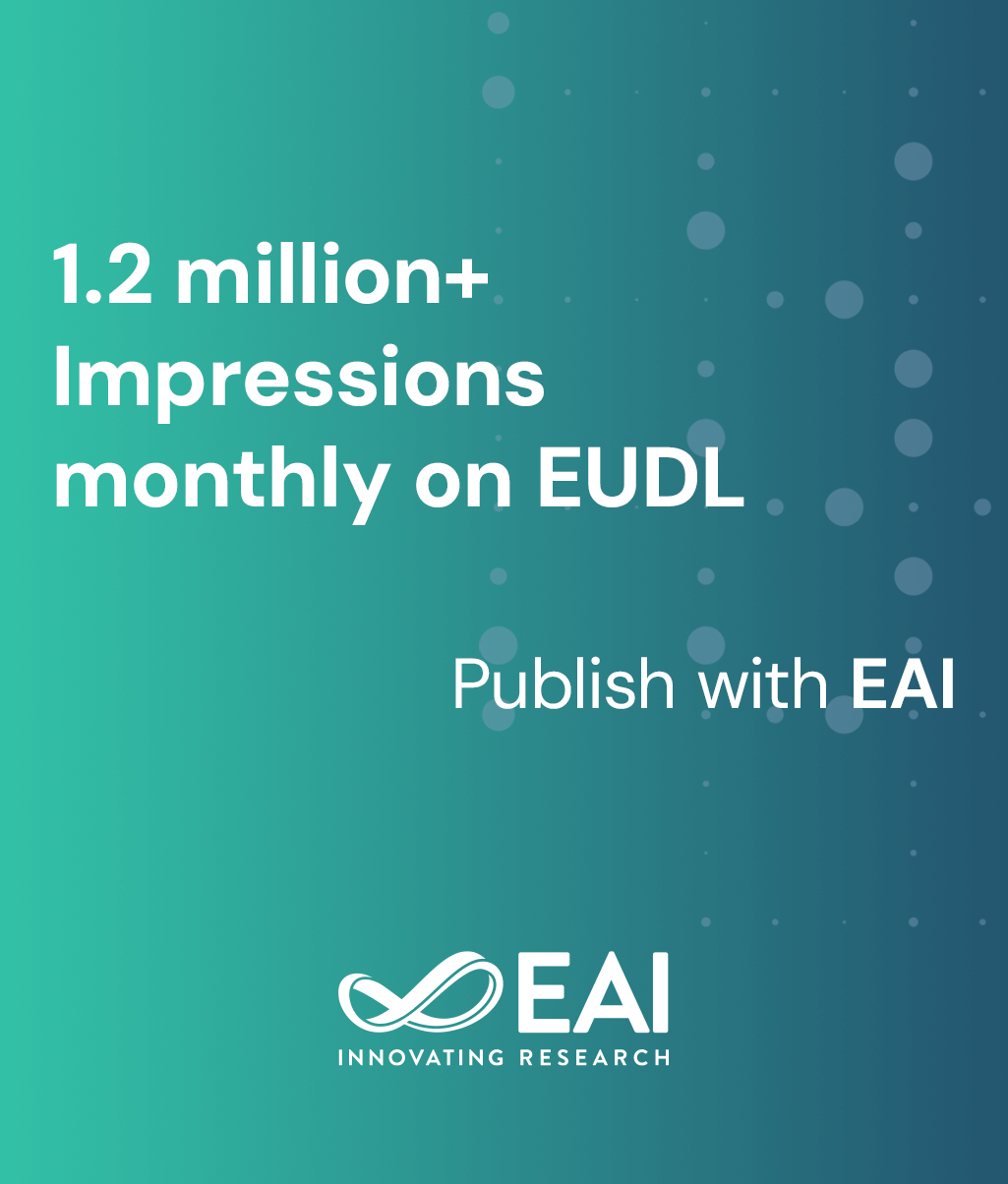
Research Article
Tracking of Vehicle Tax Violations Using Vehicle Type and Plate Number Identification
@INPROCEEDINGS{10.4108/eai.2-5-2019.2284610, author={Arsan Kumala Jaya and Zahir Zainuddin and Syafruddin Syarif}, title={Tracking of Vehicle Tax Violations Using Vehicle Type and Plate Number Identification}, proceedings={1st International Conference on Science and Technology, ICOST 2019, 2-3 May, Makassar, Indonesia}, publisher={EAI}, proceedings_a={ICOST}, year={2019}, month={6}, keywords={vehicle tax vehicle type number plate gmm anpr knn}, doi={10.4108/eai.2-5-2019.2284610} }
- Arsan Kumala Jaya
Zahir Zainuddin
Syafruddin Syarif
Year: 2019
Tracking of Vehicle Tax Violations Using Vehicle Type and Plate Number Identification
ICOST
EAI
DOI: 10.4108/eai.2-5-2019.2284610
Abstract
This paper describes tracking violations of vehicle tax using the identification of type and number plates on vehicles. The case study of this research is in Sulawesi Selatan (Indonesia). The Gaussian Mixture Model (GMM) algorithm is used to detect vehicle types and the Automatic Number Plate Recognition (ANPR) algorithm for detecting vehicle license numbers. The proposed system uses a digital camera with a camera height of 250 cm and a camera tilt angle of 55 degrees. The proposed system design is the stages of preprocessing, feature extraction, feature selection, model selection, and database. For the introduction of types of vehicles are classified based on predetermined ROI while the introduction of vehicle numbers is trained using k-Nearest Neighbor (KNN). The results of the proposed system accuracy are 91% for vehicle type detection and 70% for vehicle license number detection.